DeepMind's RETRO vs Google's LaMDA
Science & Technology
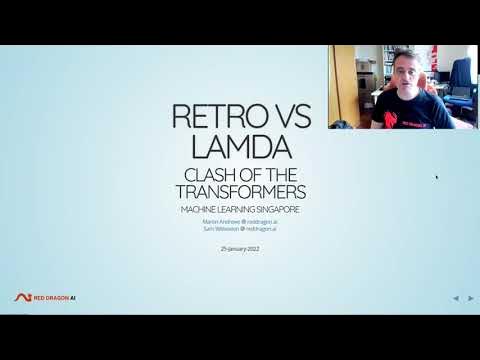
Introduction
DeepMind and Google have recently made significant advancements in transformer-based language models. DeepMind's RETRO model and Google's LaMDA model offer unique features and improvements in terms of size, factuality, efficiency, and safety. Let's delve into a detailed comparison between these models and explore the key advancements they bring to the field of natural language processing.
Transformer Basics
Before diving into the comparison, let's briefly discuss how transformers work. Transformers are neural network models that process sequential data, such as text, by focusing on the relationships between words. They consist of transformer layers that allow information to flow between words, leveraging attention mechanisms to incorporate context.
Battle for Size: Bigger is Better?
In the race to build better models, there has been a focus on increasing model size, with the assumption that bigger models perform better. OpenAI's GPT-3, which boasts 175 billion parameters, captured significant attention. However, subsequent models, such as Microsoft and NVIDIA's Megatron-Turing NLG with 530 billion parameters, and DeepMind's Gopher with 280 billion parameters, have showcased even better performance. It is important to note that larger models may not always guarantee the best outcomes.
Advancements in Factuality, Efficiency, and Safety
DeepMind and Google have made notable strides in improving factuality, efficiency, and safety within transformer models. Factuality refers to the accuracy of the information generated by the model, while efficiency pertains to the speed and resource requirements of the model. Safety ensures that the model avoids generating offensive or harmful content.
DeepMind's RETRO model prioritizes efficiency and factuality. By incorporating dense embedding search and retrieval mechanisms, the model can look up facts in real-time rather than memorizing them in model weights. This approach allows for easy updates and enhances model performance with fewer parameters.
On the other hand, Google's LaMDA model emphasizes safety and groundedness. With a focus on preventing the model from generating false information, LaMDA implements re-ranking and fine-tuning approaches. These techniques enable the model to select the most sensible, specific, and safe responses from a range of possible outputs.
Both RETRO and LaMDA demonstrate impressive performance, with DeepMind reporting better performance than larger models like GPT-3. RETRO's smaller size of less than 8 billion parameters offers comparable results to models with significantly more parameters.
Keywords
- RETRO
- LaMDA
- Transformer models
- Factuality
- Efficiency
- Safety
- Model size
Introduction
What are RETRO and LaMDA? RETRO is DeepMind's efficient and factually grounded language model that incorporates dense embedding search and retrieval mechanisms. LaMDA is Google's safety-focused language model that employs re-ranking and fine-tuning approaches to ensure sensible, specific, and safe responses.
How do RETRO and LaMDA improve factuality? RETRO avoids storing facts in model weights and instead looks up facts in real-time using retrieval mechanisms. LaMDA undergoes re-ranking and fine-tuning processes to select the most factual responses.
What are the advantages of smaller models like RETRO over larger models? Smaller models, like RETRO, offer comparable performance to larger models while requiring fewer parameters and resources. This makes them more efficient and easier to update.
How does LaMDA prioritize safety in language generation? LaMDA implements re-ranking and fine-tuning to select the safest responses and prevent the generation of offensive or harmful content.
Are RETRO and LaMDA superior to GPT-3? RETRO and LaMDA have demonstrated better performance than GPT-3 in terms of factuality, efficiency, and safety. They showcase the potential for smaller models to outperform larger ones.