AI For Developers #18
Entertainment
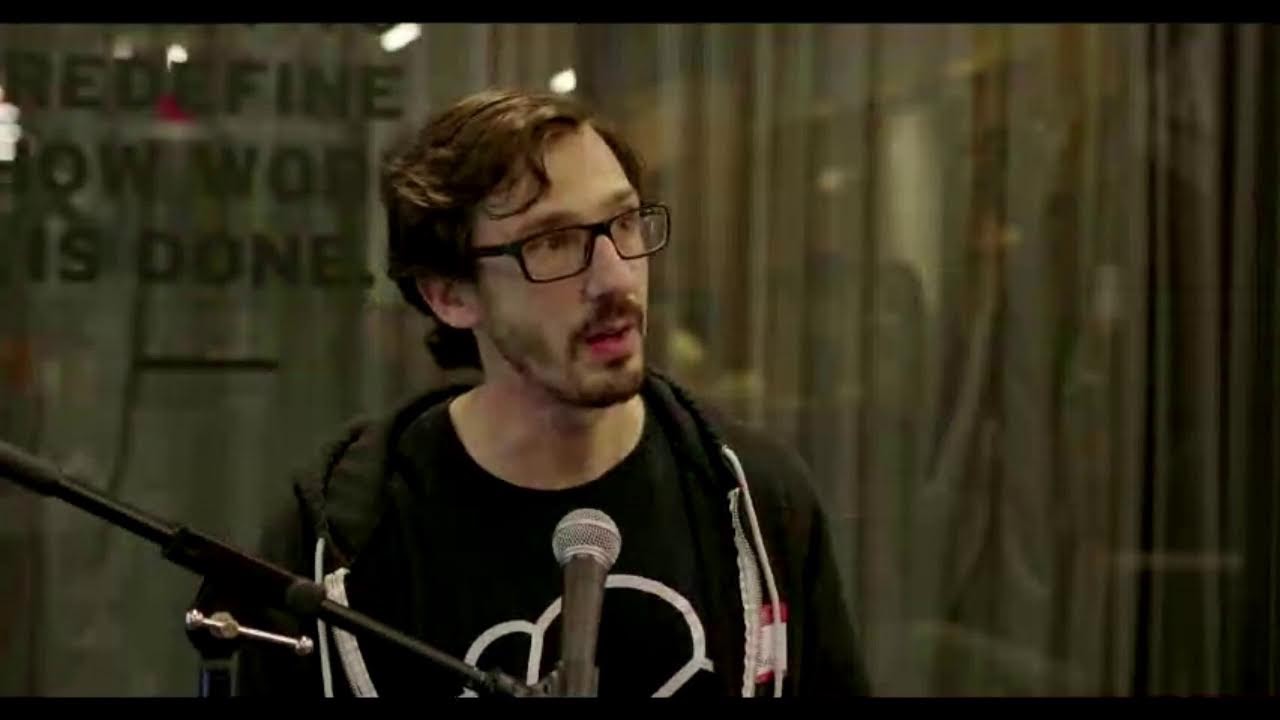
Introduction
Welcome to the 18th edition of AI For Developers! Today, we had an engaging session filled with insightful discussions surrounding the advancements in AI, particularly focusing on small language models (SLMs), misconceptions about retrieval-augmented generation (RAG), and various emerging technologies.
Overview of AI User Group
Tonight marked another milestone for the AI User Group, with an exciting announcement that we are moving into our own dedicated venue at 972 Mission Street! After two years of navigating various temporary venues, we are thrilled to host weekly events to help professionals explore and learn about AI tools, ranging from meetups to workshops and hackathons.
We also announced our collaboration with Active Loop for a conference on October 17, focused on data leaders. This conference is free for attendees who apply, providing a rare opportunity for data professionals to learn and connect.
Features of the Event
The evening began with a demonstration by Josh from Prem AI, who introduced attendees to the power of small language models. He discussed how these models can efficiently handle tasks that require less computational power, making them a viable option for developers working within budget constraints or in environments with limited connectivity.
Prem AI focuses on creating optimized small language models tailored for specific applications, and Josh shared key advantages and challenges associated with deploying these models. The ability of small language models to work offline, handle real-time responses, and be cost-efficient presents numerous opportunities across different domains.
Key Advantages of Small Language Models
- Cost-Effectiveness: Small language models require less computational power, making them suitable for organizations with budget constraints.
- Speed: They can provide rapid responses which are crucial for applications requiring real-time results.
- Versatility: Small language models can be customized and fine-tuned for specific domain-related tasks, yielding superior results compared to generic large-language models.
Josh also touched on some limitations such as contextual understanding and potential accuracy concerns, underscoring the importance of careful fine-tuning.
Misconceptions About RAG
Following Josh's presentation, Philip from Elastic took the stage to address three common misconceptions about retrieval-augmented generation (RAG). He explained that RAG combines the strengths of large language models with the retrieval of context-specific data to improve answer generation.
- Vector Search vs. Other Retrieval Methods: Philip highlighted that RAG does not solely rely on vector search for retrieving relevant information. He explained the importance of combining multiple search techniques, including keyword searches, to enhance contextual retrieval.
- LLMs Beyond Generation: LLMs can also preprocess queries and restructure data to streamline the retrieval process, rather than just generate output at the end.
- Larger Context Windows: The idea that larger context windows make RAG unnecessary is misleading. Philip emphasized that RAG is beneficial to maintain focused relevance, which larger context windows might dilute.
The discussion concluded with real-time demonstrations using Elastic’s technology, showcasing the efficiency of hybrid search techniques.
Community Engagement
Attendees were welcomed to share their announcements during the community news segment, allowing for networking and potential collaboration opportunities. The event wrapped up with pizza and networking at around 8:05 PM.
Conclusion
The AI User Group event brought together developers, data leaders, and technology enthusiasts to delve into the latest advancements in AI tools and techniques, fostering an environment for collaboration and innovation.
Keywords
- AI User Group
- Small Language Models
- Retrieval-Augmented Generation
- Cost-Effectiveness
- Vector Search
- Contextual Retrieval
- LLMs
- Data Leaders
FAQ
What are small language models?
Small language models are AI models that are designed to efficiently handle tasks with reduced computational resources, making them practical for various applications.
Why are small language models considered advantageous?
They offer speed, cost-effectiveness, and versatility in fine-tuning for specific domain-related tasks.
What is retrieval-augmented generation (RAG)?
RAG combines large language models with context-specific information retrieval to enhance the generation of relevant and precise answers.
Do small language models and RAG work together?
Yes, small language models can be fine-tuned to work effectively within RAG applications, providing targeted responses based on retrieved data.
How can organisations benefit from using small language models and RAG?
Organisations can leverage them for cost savings, speed in processing, and improved accuracy in answers by focusing on specific data relevant to their domain or sector.