AI for TB Detection in Nigeria. Impact First Summit. Lisbon, Portugal. Oct 2024
Science & Technology
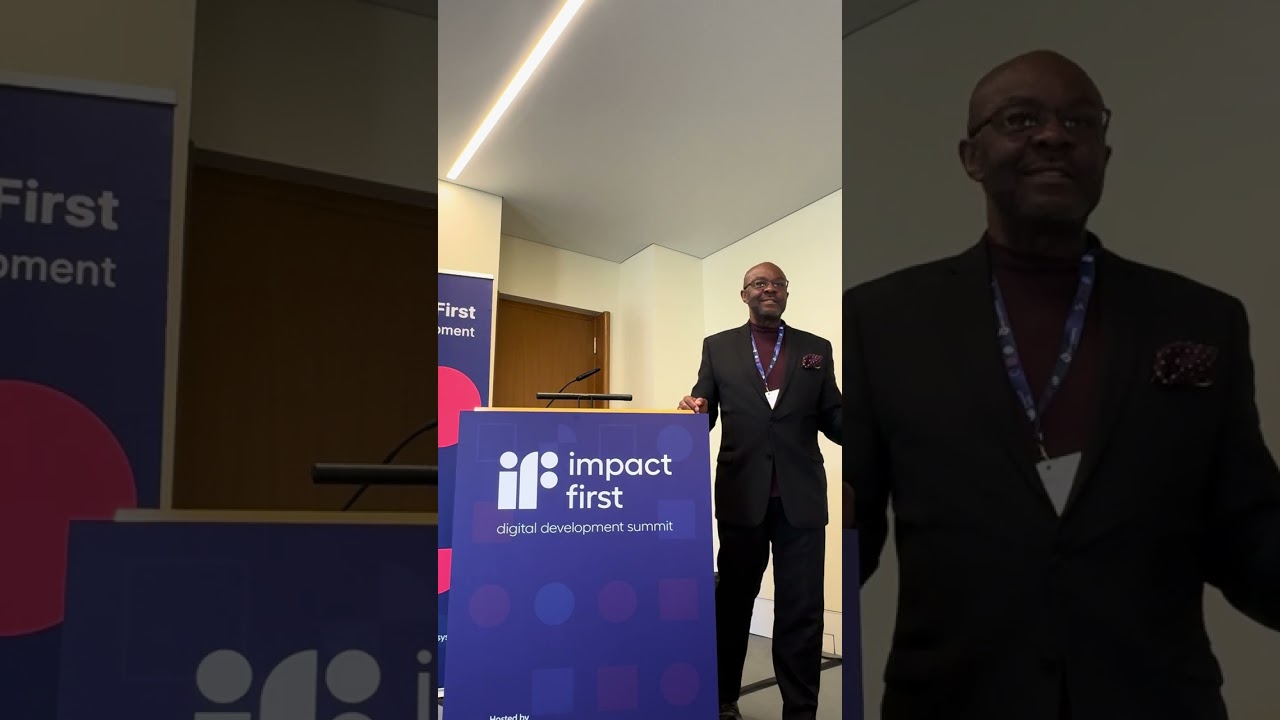
Introduction
Introduction
In this article, we discuss the innovative use of artificial intelligence (AI) for tuberculosis (TB) detection in Nigeria, emphasizing the successful implementation of an early warning disease surveillance system. This system leverages real-time data processing to identify potential outbreaks and mobilize healthcare resources effectively. The initiative is part of a broader objective to enhance healthcare delivery in Nigeria, particularly in underserved areas.
The Origin of the Initiative
The inspiration for utilizing AI in disease detection came from the past experiences with infectious diseases such as Ebola and methanol poisoning in Nigeria, which gained attention only after a significant number of fatalities occurred. This lead to the hypothesis: what if we could preemptively gather and analyze data to signal an outbreak before it escalates into a crisis? By developing an early warning system, we aim to not only identify ongoing health threats but also avert potential outbreaks through data-driven insights.
System Architecture
The AI-based disease surveillance system is designed to be data input agnostic, allowing integration with various Electronic Medical Records (EMR) systems, primary healthcare data collection platforms, and other data sources. The system processes data through cloud technology, yielding three critical outputs: positivity alerts, heat maps, and general reports.
Data Input
Our approach to data gathering is inclusive, pulling from various platforms across the healthcare ecosystem. This flexibility enables us to work with both fully developed digital systems and less sophisticated methods currently in use.
The Three Outputs
Positivity Alerts: These alerts notify program managers about identified TB hotspots at the community level. Alerts are sent out bi-weekly, prompting healthcare workers to mobilize for community screenings based on evidence of increased TB positivity rates.
Heat Maps: Heat maps provide a visual representation of TB hotspots and assist in strategic resource allocation for case detection. Although helpful for planning, these maps have proved less effective as real-time actionable outputs compared to positivity alerts.
General Reports: These reports summarize the data collected over time. However, feedback indicates that stakeholders often prefer receiving key insights directly rather than navigating through comprehensive dashboards that are not used regularly.
Implementation and Results
We played a crucial role in establishing Nigeria’s National TB Surveillance platform. Our collaboration with various local programs demonstrated that focusing resources on identified hotspots led to significant improvements in case detection.
In one notable initiative, community screening led to the identification of numerous TB cases in previously overlooked areas. A retrospective analysis revealed a 25% reduction in the number needed to screen for diagnosing a TB case in hotspot regions compared to non-hotspot areas. Such results underline the efficacy of data-driven approaches in enhancing public health interventions.
HIV Integration
Beyond TB detection, the platform has been adapted for HIV surveillance, focusing on preventing interruptions in treatment. By analyzing patient histories and identifying individuals at high risk of defaulting on medication, healthcare workers can prioritize interventions, thus improving adherence to treatment regimens.
Future Directions
As we refine our algorithms with incoming data, our goal is to evolve from merely identifying where TB cases occur to predicting future occurrences. The potential to integrate machine learning and natural language processing will enhance the system’s usability and contextual relevance.
Conclusion
The experience gained from implementing AI for TB detection in Nigeria showcases the immense potential of data-driven solutions in improving public health outcomes. Our journey continues as we aim to innovate further, ultimately leading to more effective disease prevention and control strategies.
Keywords
AI, tuberculosis, TB detection, Nigeria, disease surveillance, positivity alerts, heat maps, public health, machine learning, HIV
FAQ
Q1: How does the AI system detect potential TB cases?
A1: The AI system analyzes incoming data for positivity rates and generates alerts to inform healthcare workers of potential TB hotspots.
Q2: What are positivity alerts, and why are they important?
A2: Positivity alerts are notifications sent to program managers indicating where increased TB cases have been detected, guiding immediate community screening efforts.
Q3: How do heat maps contribute to TB detection strategies?
A3: Heat maps visually represent TB hotspots, helping allocate healthcare resources effectively, though they are secondary to actionable alerts.
Q4: How is the AI system adapting for HIV surveillance?
A4: The system analyzes patient data to identify those at risk of defaulting on treatment, focusing resources on improving adherence to HIV medications.
Q5: What future improvements are anticipated for the AI model?
A5: Future iterations of the model aim to predict TB occurrences rather than just identify existing ones, incorporating machine learning techniques for greater accuracy and efficiency.