AI Implementation Advice for Data Leaders
Science & Technology
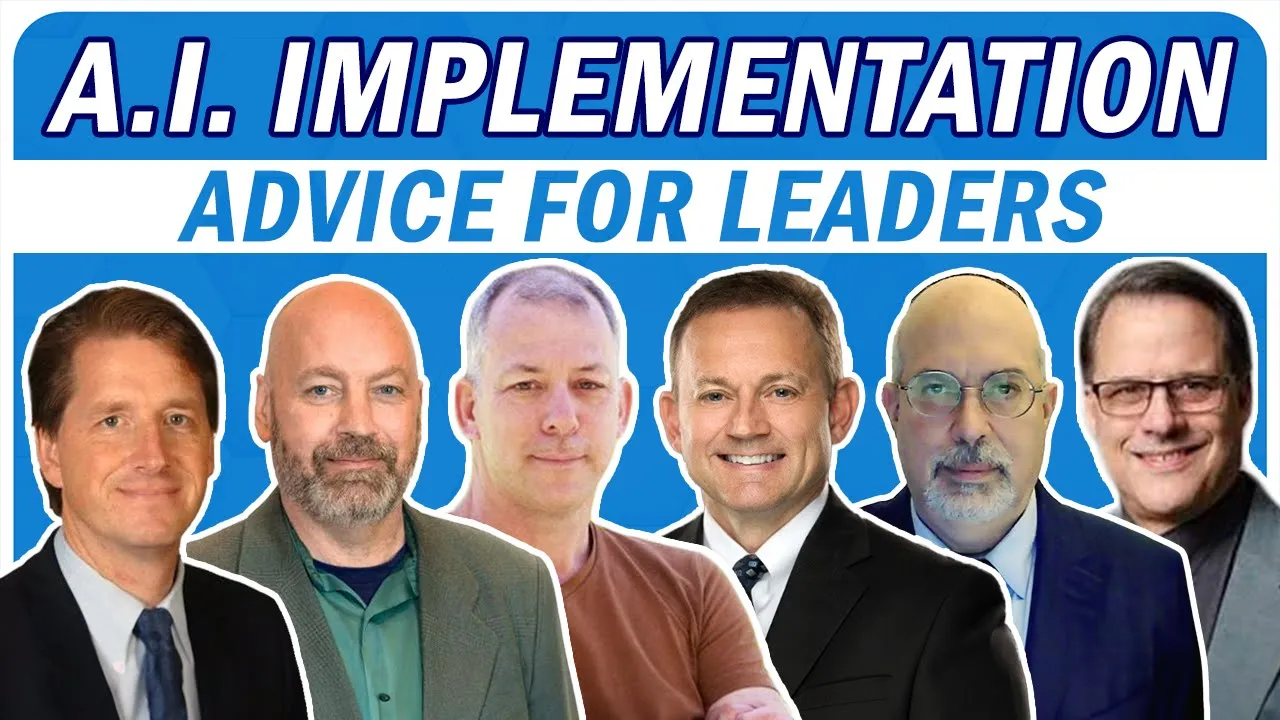
Introduction
As organizations navigate the transformative landscape of artificial intelligence (AI), particularly generative AI, industry experts emphasize the importance of a thoughtful and strategic approach. The current technological shift represents a crucial inflection point, and leaders in data governance are urged to leverage this opportunity effectively while avoiding common pitfalls associated with AI implementation.
Expert Oversight is Essential
While generative AI presents promising capabilities, experts warn against relying solely on machine outputs. Organizations should ensure that domain and legal expertise are embedded within teams to mitigate risks, avoid privacy violations, and maintain consumer trust. Decision-making should not be left entirely to AI, especially considering the complexities inherent in various business operations.
Learning from the Past
Reflecting on past technology trends—such as Hadoop implementations—suggests that organizations often place undue faith in shiny new tools without a sound understanding of the underlying data. The two critical factors behind successful AI integration are:
- Data Quality: Having high-quality, well-governed data is essential for AI systems to function effectively. Organizations need to implement controls to ensure data integrity.
- Systemic Integration: Successful AI implementation requires a long-term view that takes into account existing systems and their complexities. It involves gradual integration rather than expecting instant results simply from adopting new technology.
Balancing Possibilities and Realities
Organizations must remain cautious about the over-promising nature of AI. While the potential benefits are immense, clarity on what AI can and cannot do is vital. Leaders should actively build in-house expertise focused on AI to explore its practical applications for their specific business needs.
Implementing Guardrails
A recurring theme in discussions about AI is the need to establish robust guardrails to navigate its use. Organizations must thoroughly understand the implications and risks associated with the data they input into AI models, as well as the potential consequences of their decisions. Engagement must be business-focused, ensuring that AI initiatives yield tangible value rather than merely serving as a technical exercise.
Regulatory Landscape Awareness
Looking toward the future, organizations should be aware of legislative developments, such as the EU AI Act, that hold them accountable for their AI implementations. Compliance with these regulations will require organizational transparency regarding how AI models and tools are created and used. Limiting the scope of AI implementations and thoroughly testing these models is advisable to align with best practices and legal requirements.
Preparing for the Future
Anticipating the evolving landscape of AI in 2024 and beyond, organizations will face a dual challenge: the pressure to adopt generative AI for productivity gains and managing compliance risks effectively. Establishing a strong AI governance program early in the implementation process is crucial. This governance should outline risks, compliance needs, ethics, and other considerations to allow organizations to innovate confidently and responsibly.
The path forward is not devoid of challenges; however, with the right strategic approach, data leaders can steer their organizations toward successful AI integration that is both compliant and capable of driving significant business value.
Keywords
- AI
- Generative AI
- Data Governance
- Compliance
- Risk Management
- Data Quality
- Systemic Integration
- AI Oversight
- Guardrails
- EU AI Act
FAQ
1. Why is expert oversight necessary for AI implementation?
Expert oversight is critical to mitigate legal risks, ensure data quality, and foster consumer trust. A deep understanding of domain-specific and legal frameworks helps guide responsible AI use.
2. What are the main pitfalls organizations face in AI integration?
Organizations often struggle with data quality and lack of systemic integration, which can hinder the effectiveness of AI systems. Additionally, overestimating the capabilities of AI technology can lead to unrealistic expectations.
3. How can organizations ensure that AI initiatives provide business value?
Businesses should focus on implementing AI initiatives that address specific needs and goals, backed by a thorough understanding of the risks and opportunities involved.
4. What is the importance of compliance in AI use?
Compliance with regulations such as the EU AI Act ensures that organizations manage their AI risk responsibly. Understanding and adhering to these requirements can prevent legal issues and enhance organizational credibility.
5. What steps should organizations take to prepare for future AI challenges?
Establishing a comprehensive AI governance program that encompasses risks, compliance, and ethical considerations is essential for guiding innovation and maintaining alignment with regulatory frameworks.