AI in Modern Medicine: Diagnostics and Healthcare Outcomes
Science & Technology
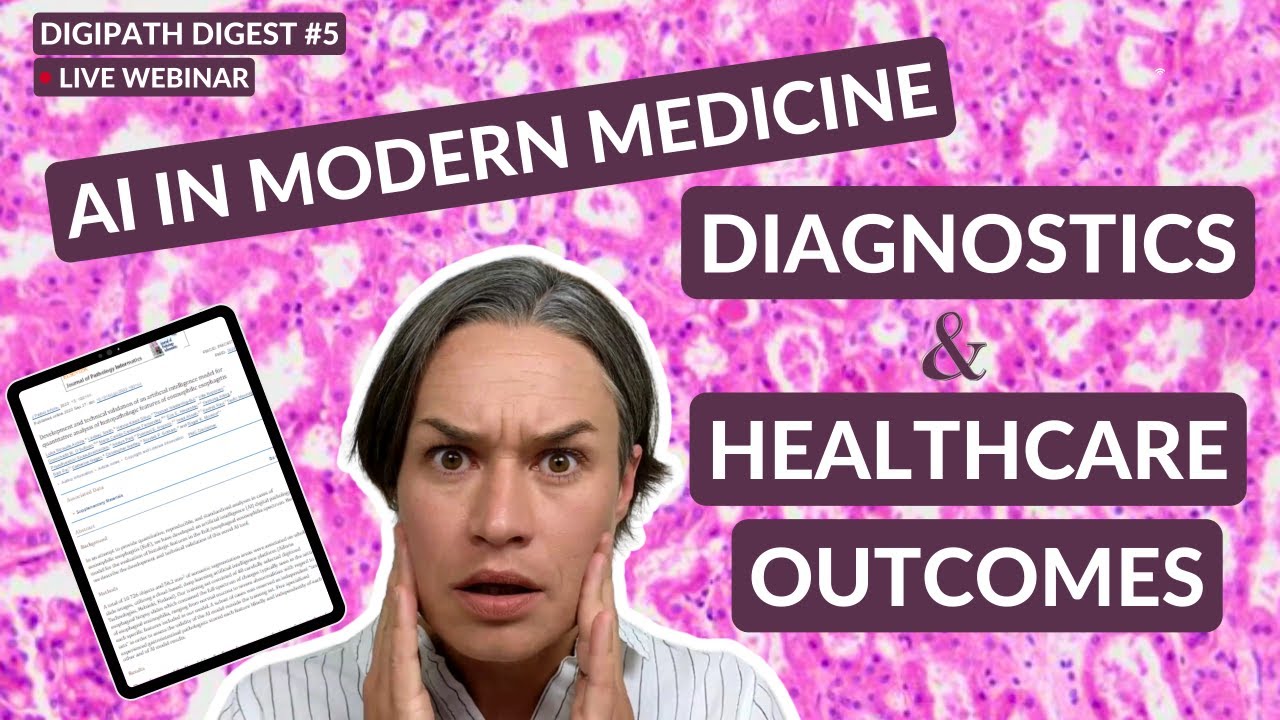
AI in Modern Medicine: Diagnostics and Healthcare Outcomes
Introduction
Date: 6 a.m. in Fairfield, Pennsylvania
Good morning, everyone! It's amazing to see you here again. I'm trying to go live across various platforms and waiting for you to join in. So, whenever you're here, say hi in the comments and let me know where you're tuning in from and what time it is in your location. Here, it’s 6 a.m. in Fairfield, PA.
Today, we're going to dive into some fascinating papers about AI in diagnostics, live across multiple platforms, overcoming minor technical issues with Instagram and TikTok. Let's make the most of our time as people are beginning to join in.
Guest Speakers
Last week, I had two cool guests on the podcast: a radiologist, Dr. Nina Cutler, and a veterinary pathologist working for Aoria, an AI-based sponsor of Digital Pathology Place.
I also shared a bit about my attempt to stay motivated with running, inspired by my coach's renewed energy and Nina Lion's book "Forever Strong," which emphasizes the importance of weight training and protein intake. I tried to find an online gym with live exercise sessions but ended up with pre-recorded content.
Reviewing AI in Modern Medicine
Paper 1: Improving Diagnostic Performance with AI
Title: Improving the diagnostic performance of inexperienced reader for thyroid nodules through digital self-learning and artificial intelligence assistance.
Journal: Frontiers in Endocrinology
Study Group: A team from Korea evaluating digital self-learning efficacy.
Details:
- 26 readers with less than a year of experience in thyroid ultrasound.
- Readers completed online learning and assessed thyroid nodules before and after training.
- AI assistance improved reader performance significantly.
Key Findings:
- The Area Under the Curve (AUC) improved from 0.679 to 0.758.
- AI CAD (Computer Aided Diagnostics) assistance led to improved sensitivity and accuracy in all readers, particularly in the context of self-training.
Paper 2: Cost Impact of AI in Breast Cancer Risk Assessment
Title: US payer budget impact of using an AI-augmented cancer risk discrimination digital histopathology platform to identify high risk of recurrence in women with early-stage invasive breast cancer.
Journal: Journal of Medical Economics
Study Group: PreciseDx, Dana Farber Cancer Institute, Brigham and Women's Hospital, Mount Sinai Health System.
Details:
- A comparative cost analysis between standard care vs its AI-assisted counterpart.
- Analyzing a hypothetical cohort of 1 million US women with early-stage invasive breast cancer.
Key Findings:
- Potential savings of $ 4 million in the first year.
- Pre-treatment patient costs dropped from $ 19,500 to $ 16,900 per patient due to AI assistance.
- Highlighted the importance of AI in reducing testing costs and increasing access.
Paper 3: Efficacy of Few-Shot Learning in Neuropathology
Title: Evaluating the efficacy of few-shot learning for GP4 Vision in neurodegenerative disease histopathology: a comparative analysis with convolutional neural networks.
Journal: Applied Neurobiology
Study Group: Mayo Clinic, University of Pennsylvania.
Details:
- 1,520 histopathological images analyzed, including H&E and immunohistochemistry.
- Utilizing few-shot learning (20 examples) to improve AI's diagnostic performance.
Key Findings:
- GPT-4 Vision improved diagnostic accuracy to 90% after few-shot learning compared to 40% without.
- Comparable performance to YOLO V8 after few-shot learning.
Paper 4: AI in Urine Cytology for Bladder Cancer Diagnosis
Title: Evaluating artificial intelligence-enhanced digital urine cytology for bladder cancer diagnosis.
Journal: Cancer Cytopathology
Company: AIXM from California
Details:
- One cytopathologist, two cytotechnologists reviewed 116 urine cytology slides.
- Using microscopy, H&E images, and the AIXM AI support platform (AX Euro).
Key Findings:
- Sensitivity improved from 25-32% to 63% with AI assistance.
- Reduced screening time by 52-83%.
Concluding Remarks
Digital pathology and AI are making significant strides in various medical fields, from reducing costs to improving diagnostic accuracy. As AI technology advances, it becomes increasingly integral to modern medical workflows.
Let me know where you're tuning in from, and join the discussion about these papers or any questions you may have about AI in pathology.
Keywords
Keywords:
- Digital Pathology
- AI
- Diagnostic Performance
- Thyroid Ultrasound
- Breast Cancer
- Few-Shot Learning
- Neuropathology
- Bladder Cancer
FAQ
FAQ:
Q1: What is Few-Shot Learning? A: Few-shot learning refers to AI models trained with only a few examples compared to traditional models that require large datasets. It adapts quickly to new tasks with minimal data.
Q2: How is AI used in Breast Cancer Risk Assessment? A: AI assists in identifying high-risk patients for recurrence in early-stage breast cancer, reducing the need for expensive gene expression tests and aiding in more accurate prognostic evaluations.
Q3: What improvements did AI bring to Urine Cytology for Bladder Cancer? A: AI significantly improved diagnostic sensitivity and reduced screening time, making the diagnostic process more efficient while maintaining high accuracy.
Q4: What was the impact of AI on diagnosing thyroid nodules? A: AI-assisted readers showed significant improvement in diagnostic performance, enhancing the accuracy and sensitivity of inexperienced radiologists in identifying thyroid nodules.
Q5: How does AI influence cost savings in medical diagnostics? A: AI reduces the need for expensive molecular tests, cutting down on testing and treatment costs while increasing diagnostic efficiency and accuracy. A comparative analysis in breast cancer diagnostics showed a significant reduction in costs per patient.
For more detailed insights, join our next live stream or check out our latest publications on AI in medical diagnostics.