Analyst vs. AI: Visualizing Employee Survey Responses with ChatGPT
Education
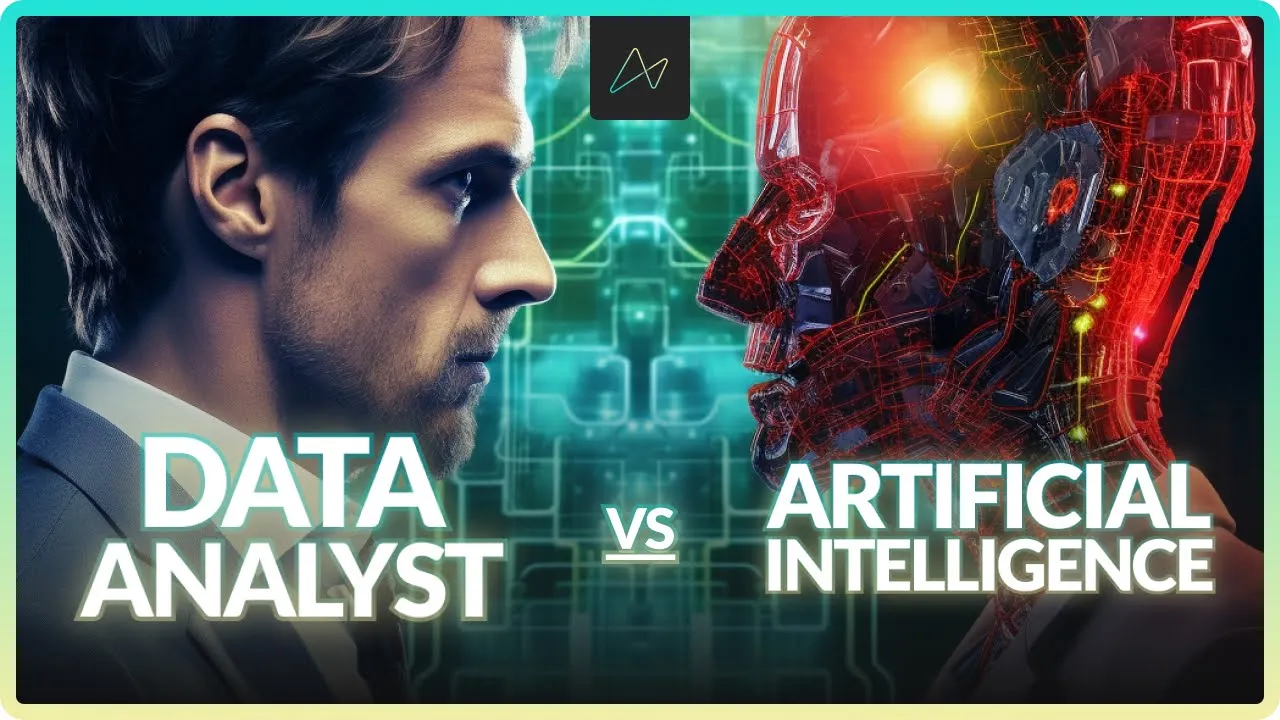
Introduction
In a rapidly evolving data landscape, the debate surrounding the capabilities of artificial intelligence (AI) versus human analysts continues to gain momentum. Chris from Maven Analytics dives into this ongoing conversation by hosting a "skills showdown" between an analyst and AI through the lens of an employee survey dataset. Here's a breakdown of the process, insights, and outcomes of this analysis.
Exploring the Dataset
Chris begins by introducing a dataset that incorporates actual survey responses from employees in Washington State. The data comprises unique identifiers, response statuses (complete or incomplete), departmental information, roles (e.g., director, supervisor), and 10 survey questions with responses rated from 0 to 4. The scores are interpreted as follows:
- 0: Not Applicable
- 1: Strongly Disagree
- 2: Disagree
- 3: Agree
- 4: Strongly Agree
To enrich this demonstration, Chris has previously introduced some data quality issues intentionally within the dataset. This establishes a baseline to evaluate how well AI tools, specifically ChatGPT, perform in identifying these problems in contrast to a human analyst.
Profiling and Cleaning the Data
Initially, Chris employs basic pivot tables to perform exploratory data analysis (EDA) and quality assurance (QA). He targets three primary quality issues by checking:
- Uniqueness of Response IDs: Chris identifies 15 duplicate response IDs through a pivot table, leading him to remove these duplicates.
- Inconsistent Question Wording: By reviewing the questions, he finds duplicate instances of questions due to typographical errors (e.g., "and" vs. "&") and instances of invisible trailing spaces.
- Blank Values: Chris discovers about 100 records that have blank responses, leading him to delete these from the dataset.
Following this, Chris implements cleaning techniques in Excel, including removing duplicates, standardizing question wording, and filtering out blank values.
Analyzing the Data
Once the data is cleaned, Chris sets up the dataset for visualization. He uses Excel to create calculations for the count of responses (1 to 4) and the average response per question. By excluding zero values from the averages, he gains a clearer picture of sentiment from the survey responses. The visualization employs a 100% stacked bar chart to effectively communicate the distribution of responses.
The analysis is then translated into actionable insights for the HR Director. Chris recommends enhancing employee recognition programs and fostering social connections to address areas of concern highlighted in the survey results.
The AI Perspective
Next, Chris uploads the same dataset to ChatGPT and instructs it to conduct a similar analysis. The AI promptly acknowledges the dataset structure, identifies data quality issues, and successfully removes duplicates and blank values.
Although ChatGPT initially includes zero responses in its average calculations, after Chris's prompt to exclude zeros, it adjusts appropriately. The AI produces a similar statistical overview, generating a table of response counts and averages. However, it struggles with visualizations at first but is eventually guided to create a 100% stacked bar chart with a proper color scheme.
Ultimately, ChatGPT delivers meaningful insights and recommendations based on its analysis, paralleling many of the findings made by Chris.
Recap and Takeaways
Throughout this showdown, Chris observes that ChatGPT performed admirably in terms of data cleaning and preliminary insights but required guidance to ensure accuracy in certain areas, such as the exclusion of zero values and effective visualizations.
The results highlight that while AI tools like ChatGPT can serve as efficient assistants for data analysis and visualization, they still necessitate knowledgeable oversight from skilled analysts to ensure robust and accurate outcomes.
Keywords
- Data Analysis
- Employee Survey
- Data Quality
- ChatGPT
- Visualization
- HR Recommendations
- Exploratory Data Analysis (EDA)
FAQ
Q1: What is the role of human analysts in data analysis with AI tools like ChatGPT?
A1: Human analysts provide critical insights, context, and oversight that enhance the accuracy and effectiveness of AI analyses. Skilled operators are needed to guide the AI and ensure comprehensive evaluations.
Q2: What common data quality issues were identified during the analysis?
A2: Common issues included duplicate response IDs, inconsistent question wording, and blank values in the dataset.
Q3: How did ChatGPT perform in comparison to the human analyst?
A3: ChatGPT successfully identified several data quality issues and generated insights, though it required specific guidance on excluding irrelevant data and setting up effective visualizations.
Q4: Can AI tools like ChatGPT replace human analysts?
A4: While AI tools can supplement analyst capabilities, they are unlikely to replace human analysts entirely due to the need for context, critical thinking, and subjective interpretation of results.