Best Practices for Generative AI Risk Management and Prevention
People & Blogs
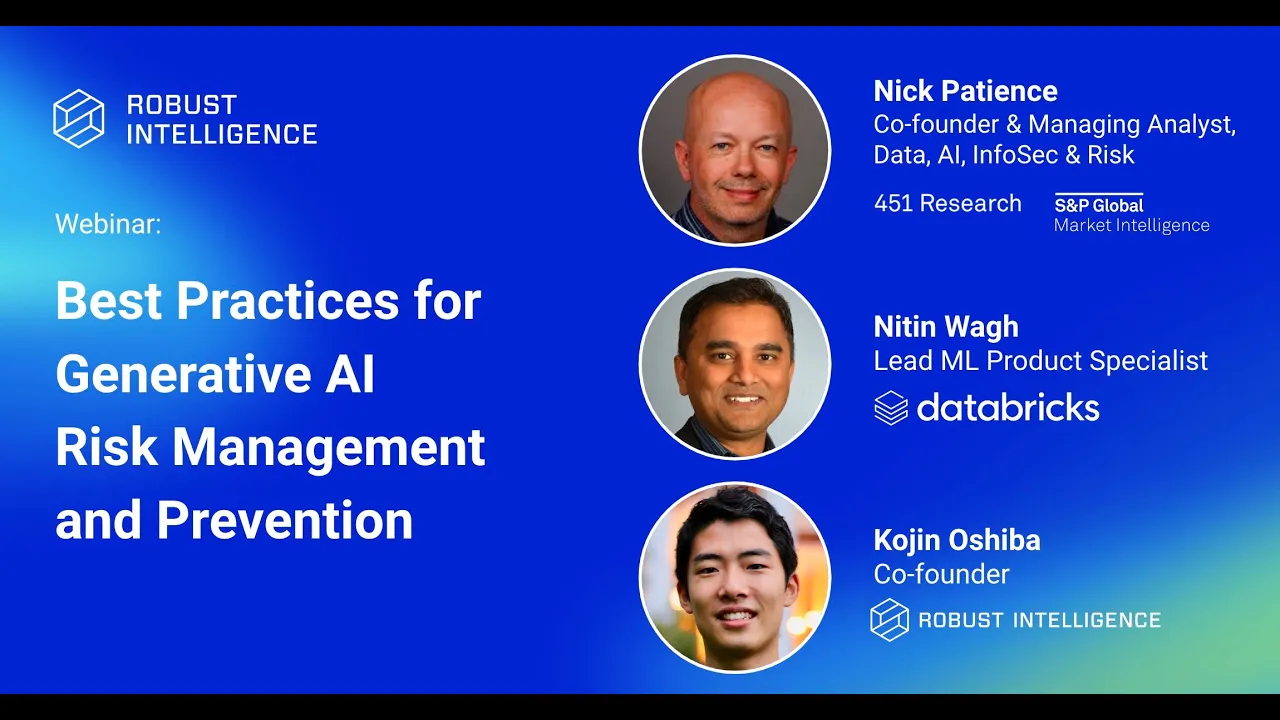
Introduction
Introduction
In a recent webinar, industry experts gathered to discuss the evolving landscape of generative AI and the associated risks. The session, hosted by Nick Patience, Managing Analyst for data AI at S&P Global Market Intelligence, included insights from Coen Asiba of Robust Intelligence and Nathan W from Databricks. The goal was to develop a comprehensive understanding of generative AI risk management and prevention strategies, focusing on best practices enterprises can implement.
Key Challenges of Generative AI
Generative AI presents distinctive risks compared to traditional AI models. The shift from batch processing to real-time output generation poses significant challenges. Unlike previous AI implementations that relied on human oversight to filter and moderate results, generative AI operates in real-time, meaning outputs are more vulnerable to manipulation. This necessitates the development of real-time controls and governance mechanisms embedded in the AI workflows.
The discussion highlighted the importance of transitioning to a structure of risk management akin to cybersecurity models, with a focus on:
- Data Quality and Governance: Data is the backbone of generative AI. Ensuring that the data used for training is clean, comprehensive, and well-governed is critical. Access control to sensitive data must be maintained throughout the AI lifecycle.
- Model Monitoring and Evaluation: Continuous validation of models in production is essential. Organizations must be prepared to adapt to model updates, evaluate model health, and thread safety measures to guard against potential abuse.
- Ethics and Bias: Ethical concerns regarding AI outputs, such as bias or toxicity, also need to be at the forefront of risk management strategies.
Best Practices for Risk Management
As the field of generative AI matures, the experts provided several best practices for organizations to consider, including:
AI Continuous Validation: Regular and automated validation processes should be built into the AI lifecycle to identify potential risks, including prompt injections and data leaks.
Embedding Security in Development: Introduce security measures early in the development process. Encourage developers to adopt test-driven development (TDD) practices to anticipate and identify vulnerabilities.
Utilizing a Risk Database: Organizations should establish or leverage existing databases to identify and track vulnerabilities across models, similar to how software security vulnerabilities are tracked.
Prompts Management: With the complexity of prompt engineering, organizations must maintain a rigorous system for managing prompt versions and ensuring compliance with data access policies.
Leverage Expertise: Organizations should invest in AI risk management expertise, drawing from both internal teams and external partners specializing in AI security.
Conclusion
The future of generative AI risk management is poised to evolve dramatically in the coming years. Companies are encouraged to foster a culture of collaboration across teams—data science, security, governance—and prioritize robust processes to mitigate risks.
Keywords
- Generative AI
- Risk management
- Real-time output
- Data governance
- Continuous validation
- Ethical concerns
- Automated validation
- Prompt engineering
- Model monitoring
- Cybersecurity parallels
FAQ
Q: What are the key differences between generative AI and previous AI models?
A: Generative AI produces outputs in real-time, requiring different security measures compared to traditional batch-processed AI models.
Q: How can companies ensure data governance in generative AI?
A: Companies should establish strict data access controls and ensure that the data used for training AI models is clean and well-governed.
Q: What is AI continuous validation?
A: AI continuous validation involves regularly and automatically evaluating models in production to identify risks such as prompt injections and data leaks.
Q: How important is prompt management in generative AI risk management?
A: Prompt management is critical, as it directly affects the outputs of generative AI. Organizations need to maintain controls for prompt versions and ensure compliance with data access policies.
Q: Why should companies incorporate external expertise into their generative AI strategies?
A: Due to the complexity of AI risks, organizations should leverage both internal capabilities and external expertise to ensure thorough oversight and optimally reduce vulnerabilities.