Can AI Read Your Mind?
Science & Technology
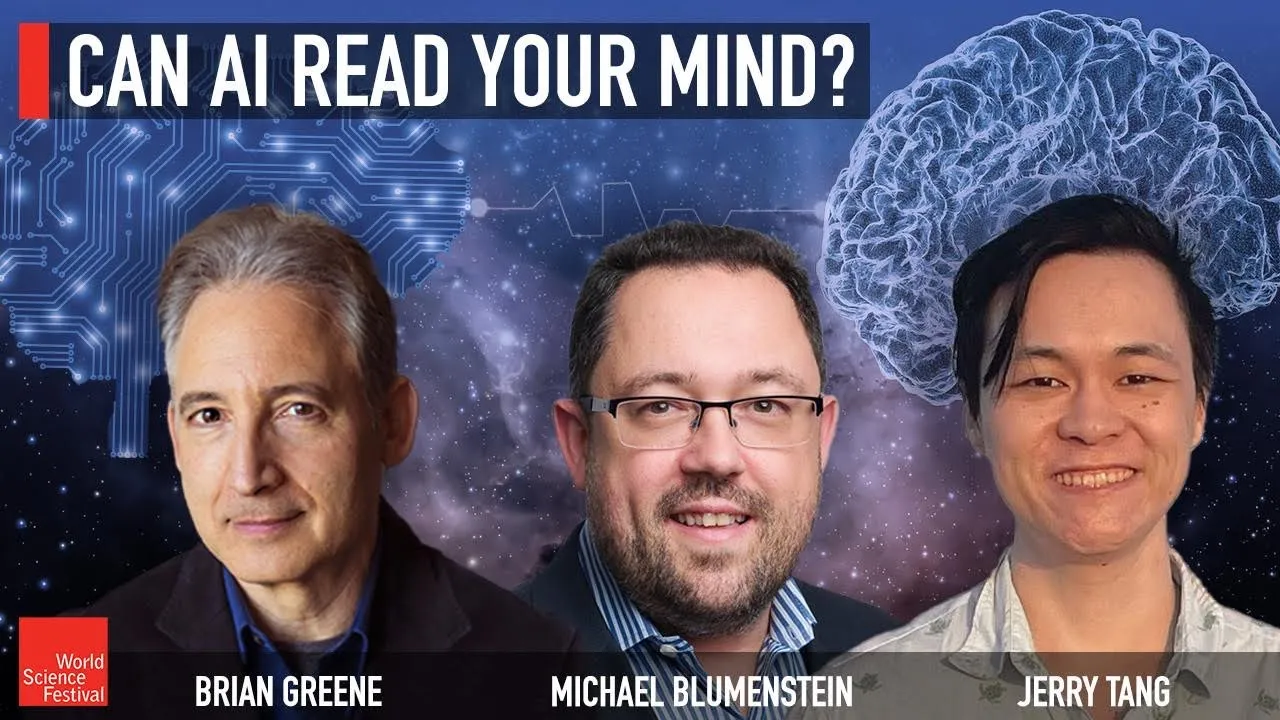
Introduction
The human heart has hidden treasures in secret kept, in silence sealed; the thoughts, the hopes, the dreams, the pleasures, whose charms were broken if revealed. Charlotte Bronte wrote those words well over a century ago, during a time when the mind was the ultimate refuge of any human being—the sacred sanctuary into which no one could enter unbidden. Anything else could be taken from us, but our thoughts? They were ours to control.
But now, a century and a half later, in a world in which our privacy is under increasing assault from advances in technology, applications, surveillance, and social media, Bronte's vision of hidden mental treasures is being revised. In recent years, researchers in at least two different laboratories around the world have made significant breakthroughs in the quest to decode complex human thoughts with custom-built AI, powered by large language models. And they’ve accomplished this without implanting electrodes in a subject’s brain.
At the University of Texas, this has been accomplished via fMRI scans. In Australia, a team at the University of Technology Sydney has gone a step further, decoding brain waves into complex speech with an EEG monitor, which, unlike an fMRI scanner, is portable. These innovations may one day give voice to the voiceless: they may one day help doctors assess a patient's level of consciousness after an accident, help families communicate with a loved one who has had a stroke, or vastly enrich the communication of someone who’s paralyzed. But these advances may also one day spell the end of a freedom our species has always taken for granted: the freedom of inner thought.
An Ethical Dilemma Worth Considering
Researchers Michael Blumenstein from the University of Technology Sydney and Jerry Tang from the University of Texas both grapple with these concerns. Michael emphasizes that because there is a connection to medical elements and the health field, there probably needs to be an extra layer of ethical considerations. Ethical standards and approaches in universities can be useful to mitigate this. Jerry echoes that mental privacy is incredibly important, suggesting that nobody's brain should be decoded without their full cooperation.
The Frontiers of AI-Powered Thought Decoding
Jerry Tang elaborates that their goal is to take brain recordings from a user and predict the words the user was hearing or imagining. This involves training a language decoder on brain responses from the user, then applying the decoder to new brain responses from that user. Using fMRI, they recorded 16 hours of brain activity while users listened to narrative stories. This rich dataset allows machine learning models to relate words to specific patterns of brain activity.
An example of this decoder’s work shows promising results: although not perfect word-for-word, the decoder often captures the general gist or meaning of the thoughts. Jerry's team finds that the general organization of language in the brain is consistent across individuals, but fine-grain differences mean decoders trained on one person don't work well on another.
Technological Comparisons and Challenges
Michael Blumenstein's team uses EEGs instead of fMRI. They measure electrical activity in the brain through a portable cap with sensors, which is less invasive. The EEG approach involves reading text aloud and correlating EEG signals to the words while using self-supervised learning for machine learning.
Unlike the high spatial resolution of fMRI, EEGs provide better temporal resolution but coarser data. Despite this, Blumenstein mentions they're achieving reasonably accurate decoding of words, though not exact. The EEG data combined with large language models allows interpretation of thoughts into coherent sentences.
The Future of Mind-Reading Technologies
Both researchers see vast potential for improving accuracy and practicality. Tang points to the need for better quality data, more powerful models, and new experimental approaches. Blumenstein highlights the need for collaboration with clinicians and health practitioners and emphasizes that we're still in the early stages of understanding how language models work in the brain.
Keywords
- AI
- Brain decoding
- fMRI
- EEG
- Language models
- Machine learning
- Mental privacy
- Medical technology
- Neural decoding
FAQs
Q: What technology does the University of Texas use for decoding thoughts?
A: They use fMRI scans to measure brain activity and decode thoughts using AI-powered language models.
Q: How is the University of Technology Sydney’s approach different?
A: They use EEG monitors instead of fMRI to decode brain waves into speech. EEG monitors are portable and measure electric activity on the scalp.
Q: What are the ethical concerns surrounding this technology?
A: The primary concern is the potential breach of mental privacy, as AI could decode thoughts without explicit permission, posing significant ethical dilemmas.
Q: How accurate are these technologies currently?
A: Both technologies show promise but aren't perfect. fMRI decoding often captures the gist of thoughts, while EEG decoding approximates about 60% accuracy in interpreting spoken thoughts.
Q: What potential applications do these technologies have?
A: They could help give voice to the voiceless, assess consciousness levels after accidents, aid stroke patients, and provide communication methods for paralyzed individuals.
Q: Will there be a time when this technology becomes user agnostic?
A: Researchers hope so. Improvements in data capture, algorithms, and machine learning could eventually make these systems more universally applicable without individualized training.