Contextual Retrieval: Anthropic's New Approach to Boost AI Accuracy
People & Blogs
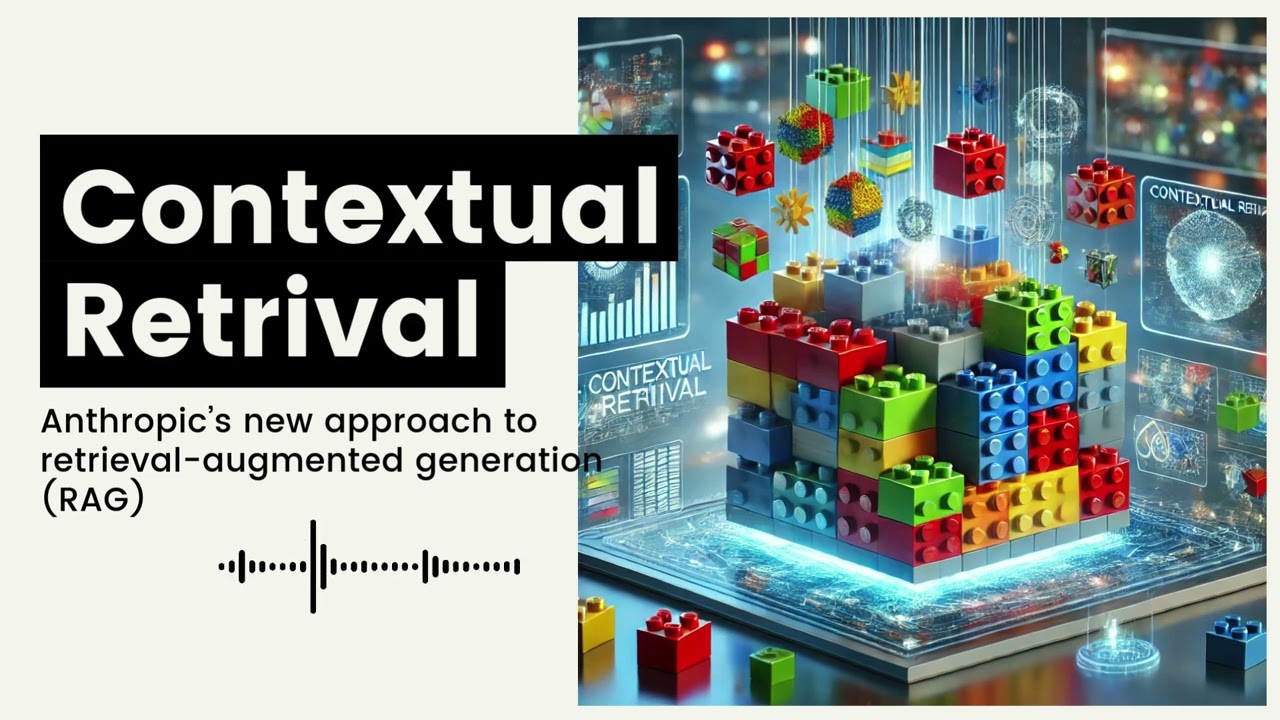
Introduction
Ever wished your AI could understand the context of your questions instead of just spitting out random facts? It seems that Anthropic has taken a huge leap in this direction with a concept they are calling "contextual retrieval." This advancement aims to move beyond traditional AI that merely recognizes keywords, striving instead for a deeper understanding of nuance and the bigger picture—something we naturally do as humans.
The Limitations of Traditional AI
Asking a typical AI a question can often be a gamble. You might get lucky and receive the relevant information you are seeking, but just as frequently, you may be met with responses that seem completely misguided, as if the AI had not read the same document. This often results from traditional retrieval-augmented generation (RAG) systems, which tend to treat information like a giant jigsaw puzzle. Each piece is analyzed in isolation, without grasping how the pieces fit together. This approach leads to nonsensical responses because the AI lacks an understanding of the overall context required to provide accurate answers.
What is Contextual Retrieval?
Contextual retrieval aims to restore context to the conversation. Anthropic employs techniques like contextual embeddings to help the AI understand the meaning of words based on their surrounding context. For instance, the word "bright" could mean something entirely different depending on whether it's about a "bright idea" or "bright light." By taking the surrounding words and sentences into account, the AI can discern these subtle differences and comprehend linguistic nuances.
Another technique is contextual BM25, which helps the AI hone in on the most relevant information by considering the entire document as context. This dual approach allows the AI to prioritize information that aligns with the broader topic, rather than merely extracting every instance of a given word.
Enhancing User Experience
For everyday users, these advancements could mean a more intelligent and seamless experience. Imagine asking your AI assistant to research a complex topic and receiving curated information pinpointing the most relevant details instead of a jumble of vaguely related snippets. This saves you the hassle of sifting through irrelevant information and piecing together a coherent narrative.
The potential benefits extend beyond just research. Think of customer service chatbots that can genuinely understand and address your concerns or educational tools that adapt to your learning style to provide clearer explanations.
The Role of Prompt Caching
Anthropic has also introduced a feature known as prompt caching, which allows the AI to retain context from previous interactions. This is significant because understanding context in real-time can require substantial computational resources. With prompt caching, the AI essentially remembers previously learned context for specific topics, making subsequent queries more efficient.
Continuous Improvement
Although contextual retrieval is a significant advancement, it's important to note that it is not a magic solution. The effectiveness of this technique relies on the quality of the underlying language models and the size of the information pools being used. Nevertheless, Anthropic continues to refine their approach, incorporating additional layers such as reranking to enhance accuracy further.
Reranking: Enhancing Accuracy
Reranking functions as an effective editor for the AI. It identifies relevant information chunks and prioritizes the best options, significantly reducing error rates by 67%. However, this added feature may come at the cost of increased processing time, especially with large datasets.
Conclusion
In essence, we appear to be on the brink of a revolution in how we interact with information thanks to contextual retrieval. As AI develops the ability to understand context with greater sophistication, the implications for how we learn, research, and create are bound to be profound and far-reaching.
Keywords
- Contextual Retrieval
- AI Accuracy
- Contextual Embeddings
- RAG System
- Semantic Nuance
- User Experience
- Reranking
- Prompt Caching
FAQ
What is contextual retrieval?
Contextual retrieval is an approach used by Anthropic to enhance AI's understanding of context, allowing it to provide more accurate and relevant information based on user inquiries.
How does contextual retrieval improve user experience?
It offers a more intelligent interaction by providing curated, context-aware answers instead of scattered and irrelevant snippets of information.
What are contextual embeddings?
These are a technique used to help AI understand the meaning of words based on their surrounding context, allowing for nuanced interpretations.
What is reranking in AI?
Reranking serves as an editing phase where the AI gathers information and prioritizes the most relevant pieces, significantly improving the accuracy of responses.
Why is prompt caching important?
Prompt caching allows AI to remember context from prior interactions, making it more efficient in responding to related queries without starting from scratch each time.