Data Science Minute - All the latest AI news to help you in your machine learning career
Science & Technology
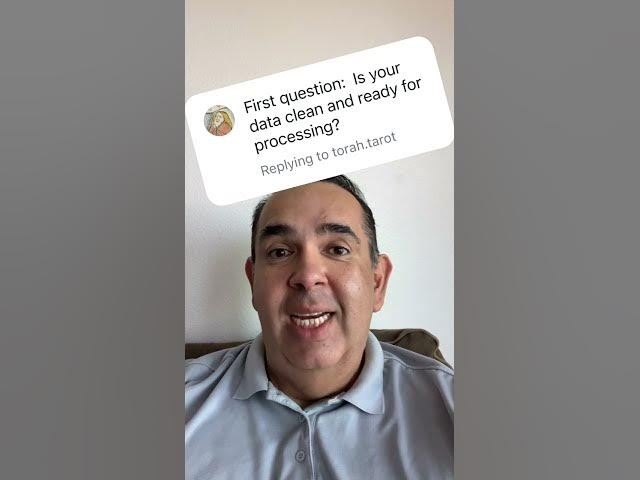
Data Science Minute - All the Latest AI News to Help You in Your Machine Learning Career
In the real world, data is never clean and it's certainly not ready for processing, no matter what the stakeholder tells you. You should always take a trust-but-verify approach to data preparation, cleaning, and acquisition. This is a significant part of what you'll be doing as a data scientist in the real world. In academic settings, data sets are often handed to you, and you receive guidance on how to work with them. However, there will be nothing like that once you start your first job. Therefore, always verify whatever anybody tells you about the data.
Keywords
- Data preparation
- Data cleaning
- Data acquisition
- Data scientist
- Trust but verify
- Real-world data
- Academic settings
- Stakeholder
FAQ
Q: Why is it important to verify data in the real world? A: In the real world, data is often messy and not ready for processing. Verifying data ensures accuracy and reliability, preventing errors in your analysis.
Q: How does data handling in the real world differ from academic settings? A: In academic settings, data sets are usually provided and come with guidance. In the real world, you have to source, clean, and prepare the data yourself.
Q: What does 'trust but verify' mean in the context of data science? A: 'Trust but verify' means you should take initial information or data at face value but perform your own checks to ensure its accuracy and completeness.