Example of bias in AI models, and how to avoid it
Science & Technology
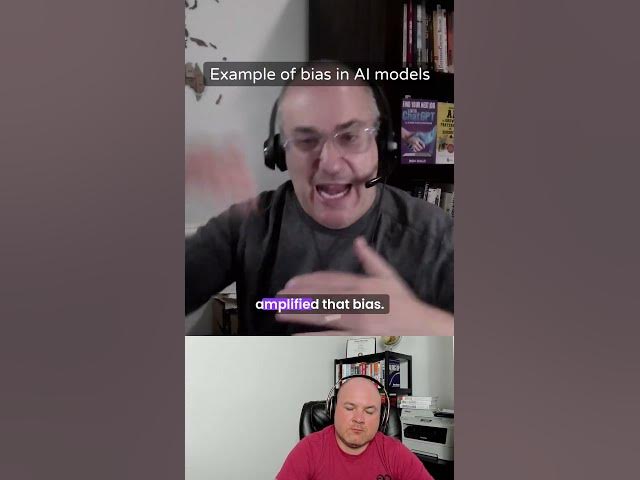
Example of Bias in AI Models, and How to Avoid It
Introduction
Amazon, for instance, developed an AI model to screen resumes. However, there was a pronounced issue: 85% of the people whose data had been used to train the model were male. As a result, the algorithm incorrectly learned that the preferred candidates were male. Consequently, any resumes indicating attendance at a female-dominated school or showcasing feminine traits were immediately dismissed. This occurred because the algorithm processed already biased data, which subsequently amplified the inherent bias.
Identifying Biases in AI
When considering the implementation of AI within an organization, several steps should be taken:
Evaluate the Provider: Scrutinize the company that provides the AI technology. Ensure the algorithms they deploy have been rigorously tested to mitigate biases.
Continuous Verification: Always double-check AI decisions. If using AI at the human resources level for tasks such as resume screening or promotions, verify the outcomes. For example, ensure that rejected candidates lacked the necessary qualifications rather than being excluded due to inherent bias.
Importance of Human Oversight
To guarantee fair and unbiased results, human oversight is crucial. By manually validating the AI's decisions, organizations can ensure that rejections are for legitimate reasons—such as missing qualifications—rather than inappropriate biases related to gender, ethnicity, or other factors.
Keywords
- AI model
- Bias
- Amazon
- Resume screening
- Human resources
- Algorithm
- Verification
- Human oversight
FAQ
Q: Why was the AI model developed by Amazon biased? A: The Amazon AI model was biased because 85% of the data used to train the model were male-dominated, leading the algorithm to learn and amplify gender biases.
Q: What should organizations do to avoid biases in AI systems? A: Organizations should evaluate the AI provider's algorithms for bias testing, maintain continuous verification of the AI's decisions, and involve human oversight to ensure fair treatment of all candidates.
Q: How can human oversight help mitigate AI biases? A: Human oversight ensures that AI recommendations are double-checked, guaranteeing that rejections or approvals are based on valid qualifications rather than biased algorithmic decisions.
Q: What role does data play in AI bias? A: The quality and composition of data used to train AI models play a critical role. Biased data can lead to biased outcomes, as seen in the Amazon example.
Q: How can AI bias affect HR decisions? A: AI bias can result in unfair hiring or promotion practices, such as systematically rejecting candidates based on gender, ethnicity, or other unrelated attributes to job qualifications.
This structure provides a comprehensive understanding of AI biases, emphasizing the importance of selecting tested algorithms and maintaining human oversight to ensure fairness and mitigate bias.