General idea of fine tuning the GenAI model
Science & Technology
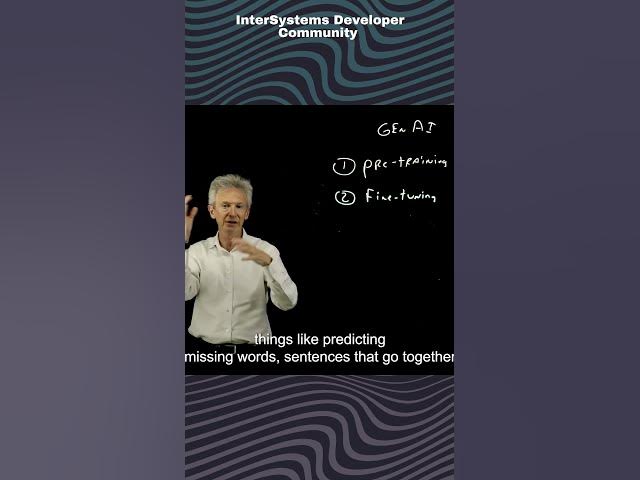
Introduction
Fine-tuning is an optional but highly beneficial step in the process of training generative AI models. Although not mandatory, many practitioners choose to fine-tune their models because it is relatively straightforward and significantly enhances the model's performance.
What is Fine-Tuning?
Fine-tuning is akin to pre-training, where a model is initially trained on a broad corpus of text. This initial training typically involves tasks such as predicting missing words and discerning sentences that logically fit together. However, fine-tuning goes a step further by using documents that are specific to your own subject matter.
The Process of Fine-Tuning
During fine-tuning, you take your own documents—whether they are internal policies, technical documentation, or even source code (for coding models)—and train the model on these texts. This process allows the model to understand the nuances of your specific language and the unique ways that information flows in your particular environment.
The primary goal of fine-tuning is to tailor the model’s responses and performance to be more aligned with your specific context, making it more effective and relevant to your needs.
Conclusion
Through fine-tuning, models become more adept at navigating the particular terminologies and frameworks relevant to your field, ensuring they deliver responses that are not only accurate but also contextually appropriate.
Keywords
- Fine-tuning
- GenAI model
- Pre-training
- Subject matter
- Internal policies
- Source code
- Contextual understanding
FAQ
Q: What is the purpose of fine-tuning a GenAI model?
A: The purpose of fine-tuning is to enhance the model's performance by training it on specific documents related to your subject matter, allowing it to better understand context and terminology.
Q: Is fine-tuning necessary for all GenAI models?
A: No, fine-tuning is optional. However, it is highly recommended as it can significantly improve the model's relevance and accuracy in specific applications.
Q: How does fine-tuning differ from pre-training?
A: Pre-training involves training a model on a large corpus of generic text, while fine-tuning involves training the model on specific documents that pertain to your unique subject matter.
Q: What types of documents can be used for fine-tuning?
A: Fine-tuning can be performed using any documents relevant to your field, such as internal policies, product manuals, technical documentation, or even source code for coding-related models.
Q: Can I fine-tune a model on a small dataset?
A: Yes, fine-tuning can be effective even with a small dataset, as long as the documents are highly relevant to the model's intended application.