Generative AI Application Integration Patterns
Science & Technology
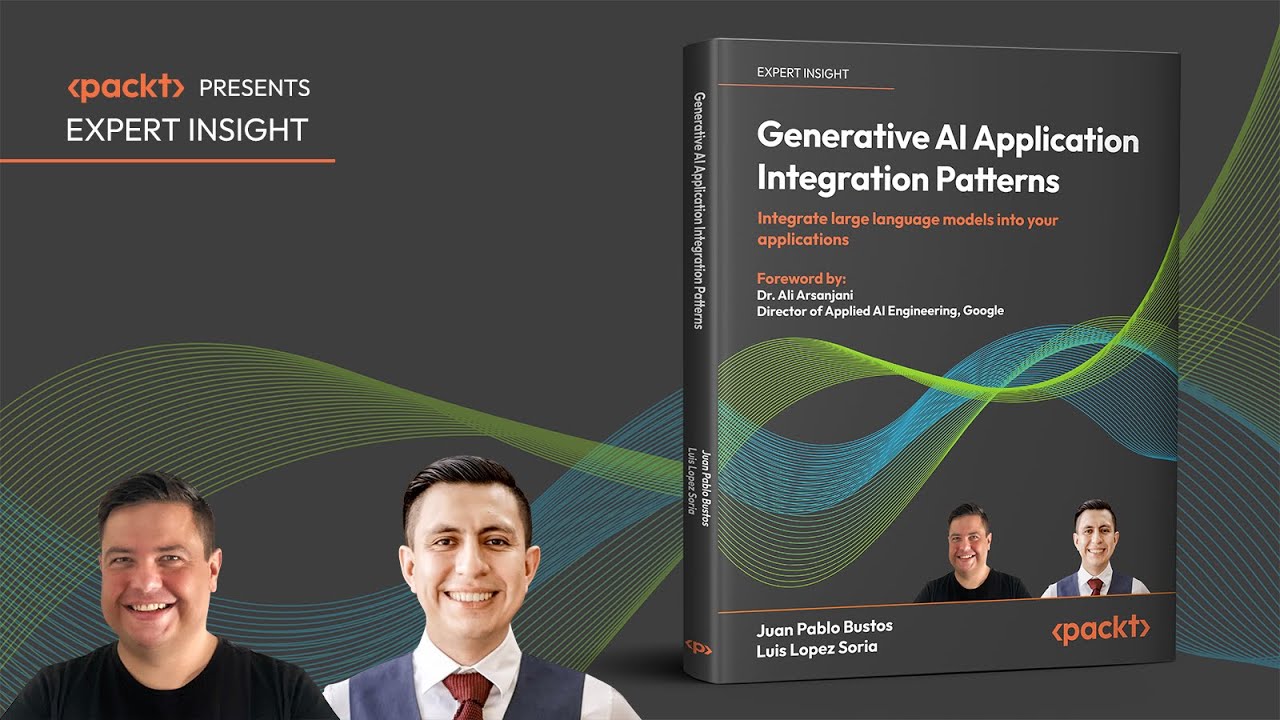
Introduction
Introduction
In a recent engaging session, experts discussed the nuances of integrating generative AI (GenAI) into applications, breaking down common myths and providing practical insights on effectively leveraging this transformative technology. Juan Buos and Luis, specialists at Google, shared their experiences and frameworks detailed in their book on this subject. This article captures the essence of their discussion, along with the significant takeaways, common challenges, use cases, and best practices for developers transitioning from traditional AI models to generative AI.
Understanding Integration Patterns
The integration of generative AI into applications involves understanding the unique patterns that characterize this technology, distinguishing it from traditional machine learning methodologies. While traditional AI models often rely on a defined input-output relationship that can cycle into extensive testing and retraining, generative AI offers a more dynamic and flexible approach. The experts emphasized the evolution from predictive AI to generative AI, highlighting how it significantly changes the product development life cycle.
Practical Frameworks
The authors advocate for a structured framework that outlines how developers can adopt generative AI in their product journeys. This includes identifying valuable use cases, balancing complexity while ensuring computational efficiency, and establishing procedures for successful deployment. The discussions emphasized that using generative AI is not merely about embracing the latest technology but effectively addressing business problems and generating tangible value.
Key Challenges
Transitioning from traditional AI to generative AI poses various challenges. Common hurdles include:
- Loss of Control: Developers may feel a lack of control over the black-box nature of generative AI models, as opposed to traditional models where they own the data and processes.
- Prompt Engineering: With generative AI's reliance on prompts, finding the right framing for questions can be a new challenge for many developers.
- Increased Costs: Hosting models in-house can be resource-intensive, prompting discussions about cost management strategies.
Responsible AI
The session also highlighted the significance of responsible AI practices. As generative AI technology becomes increasingly ubiquitous, ensuring that these systems are designed ethically becomes paramount. Without responsible oversight, risks such as biases in machine learning applications can persist, leading to unfair outcomes in use cases like resume screening or customer interactions.
Use Cases in Enterprises
Generative AI has found applications across various industries, whether enhancing customer experiences through chatbots, automating workflows, or aiding in data extraction from documents. Enterprise-level integration examples showcase the technology's ability to bridge functionality gaps across diverse sectors.
Conclusion
The recent insights from Juan and Luis offer compelling viewpoints on integrating generative AI into business processes. Their emphasis on structured approaches, identifying challenges, and maintaining ethical practices lays an excellent foundation for organizations looking to leverage this cutting-edge technology effectively.
Keyword
- Generative AI
- Integration Patterns
- Prompt Engineering
- Computational Efficiency
- Responsible AI
- Use Cases
- Enterprise Applications
FAQ
1. What are integration patterns for generative AI?
Integration patterns refer to the structured approaches or frameworks used to implement generative AI effectively within existing applications or workflows.
2. How does generative AI differ from traditional AI?
Generative AI is more dynamic and versatile compared to traditional AI, which often follows strict input-output relationships and involves defined data training cycles.
3. What challenges do developers face when transitioning to generative AI?
Challenges include loss of control over the model, understanding prompt engineering for effective interaction with models, and managing increased costs in deploying generative AI solutions.
4. Why is responsible AI important in generative AI applications?
Responsible AI practices are crucial to mitigate biases and ensure ethical standards in AI applications, especially when these systems have significant impacts on people’s lives and decisions.
5. What are some common use cases for generative AI in enterprises?
Common use cases include enhancing customer service experiences through chatbots, automating data extraction processes, improving workflow efficiencies, and generating insights from data analytics.