Generative AI Explained | What is Generative AI? | How Generative AI Works? | Generative AI Meaning
Education
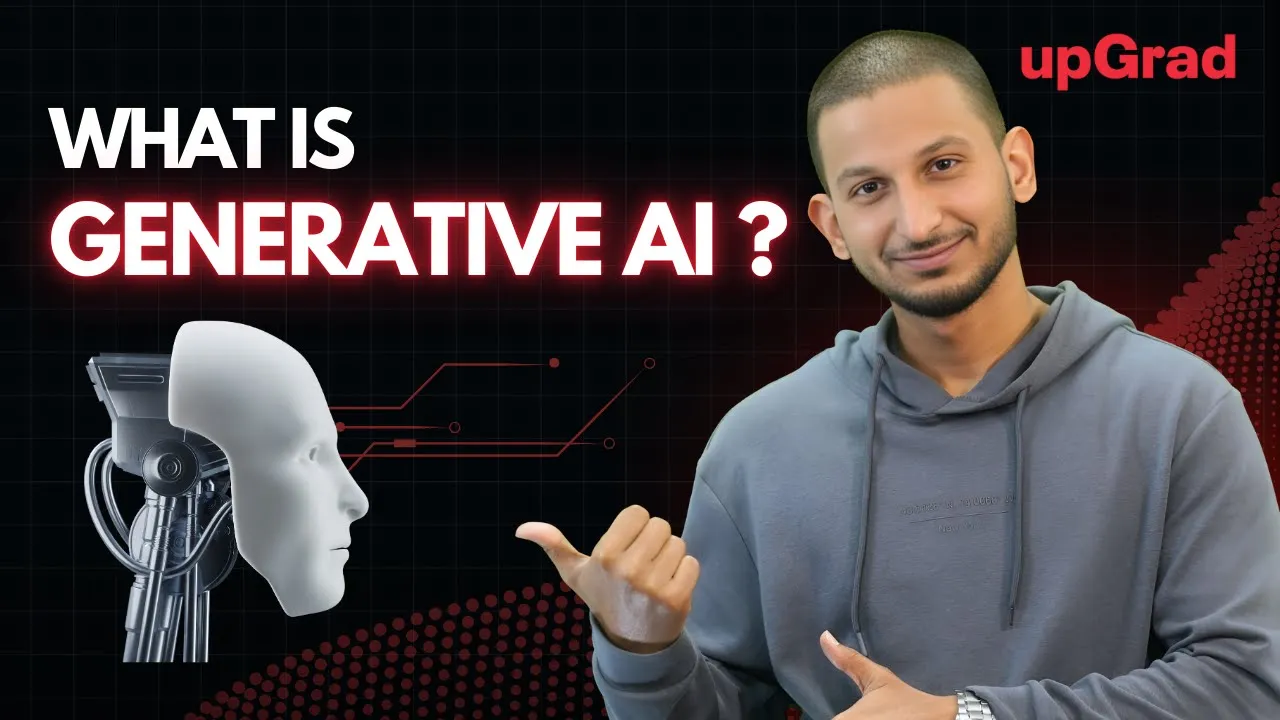
Generative AI Explained | What is Generative AI? | How Generative AI Works? | Generative AI Meaning
Welcome to the realm of generative AI, where artificial intelligence isn't just about learning; it's about creating. Let's unlock the secrets of how these digital brains are transforming ideas into reality across art, literature, and technology.
Table of Contents
- What is Machine Learning?
- What is Deep Learning?
- What is LLM?
- What is Generative AI?
- Why Should You Learn Generative AI?
- Various Generative AI Models
- Generative Adversarial Networks (GANs)
- Diffusion Models
- Variational Autoencoders (VAEs)
- Prejudice and Principles in Generative Artificial Intelligence
Before we dive into these topics, make sure to hit that subscribe button. It's just a click away. Subscribe and join our community of curious minds and bright sparks!
What is Machine Learning?
Welcome to the world of machine learning, where computers learn from data and make decisions and predictions. Machine learning is an application of AI that enables computers to learn without explicit programming. It mimics the way a young child learns to recognize animals, but with data used by computers in place of language.
Types of Machine Learning:
- Supervised Learning: Uses labeled data to train models. Example: Email spam filters.
- Unsupervised Learning: Deals with unlabeled data to understand underlying structures. Example: Customer grouping in marketing.
- Reinforcement Learning: Allows an algorithm to learn through trial and error. Example: AI in video games improving through rewards and punishments.
Deep dive into neural networks, which are inspired by the human brain and essential for deep learning.
What is Deep Learning?
Deep learning is the powerhouse behind much of the AI you encounter daily. Think of it as a supercharged version of machine learning, using multiple layered neural networks to handle vast amounts of unstructured data like images and text, making decisions and recognizing patterns at incredible speed.
Applications of Deep Learning:
- Self-Driving Cars: Navigates safely using inputs from sensors and cameras.
- Generative AI: Creates new content from art to synthetic voices.
- Voice Activated Devices and Security Systems: Perform tasks and recognize faces.
Deep learning models learn from raw data without extensive pre-processing, automating complex processes like identifying fraudulent transactions.
What is LLM (Large Language Models)?
LLMs are trained on massive volumes of text to understand and produce language like humans. They use complex architectures known as Transformers, consisting of encoders and decoders.
Key Functions of LLMs:
- Sentence Completion
- Document Summarization
- Question Answering
- Language Translation
- Content Creation
LLMs make information accessible and interactions more natural, enhancing virtual assistants and search engines.
What is Generative AI?
Generative AI is not just reactive; it's creative, capable of producing new content including text, images, videos, and music. It mimics human intelligence in areas like image recognition and natural language processing.
Uses of Generative AI:
- Creativity: Poetry, writing, and music composition.
- Scientific Research: Modeling biological processes or chemical molecules.
- Technology Development: Debugging and automation for programming languages.
- Businesses: Enhancing client support, media production, and product design.
Benefits of Learning Generative AI:
- Automation and Efficiency: Automates content creation, increasing productivity.
- Ethical and Social Implications: Raises questions on authenticity, privacy, and potential misuse.
Various Generative AI Models
1. Generative Adversarial Networks (GANs): GANs use two neural networks—Generator and Discriminator—that compete against each other to create realistic synthetic data.
2. Diffusion Models: These models initialize with random noise and gradually transform it into data resembling the training set using a series of learning processes.
3. Variational Autoencoders (VAEs): Merges principles of autoencoders with probabilistic modeling. Comprises an Encoder (compresses input) and Decoder (reconstructs input).
Prejudice and Principles in Generative Artificial Intelligence
Bias and Training Data: AI models can replicate and even increase biases based on gender, ethnicity, or other identity metrics due to biased training data.
Fairness Principles:
- Equity and Fairness: Ensure models treat all individuals equally.
- Transparency: AI systems must explain their decision-making processes.
- Accountability: Companies and developers are responsible for ethical AI use.
- Diversity and Inclusivity: Use diverse data sets to minimize bias.
- Ethical Design: Prioritize human rights and social values in AI development.
Are you ready to lead your organization into the future with generative AI? Our exclusive course, "Generative AI for Leaders," is crafted for forward-looking professionals who want to move beyond theory into real-world solutions.
If you found this article useful, please like, subscribe, and hit the bell icon to stay updated on our latest tech content. Thank you for reading!
Keywords
- Generative AI
- Machine Learning
- Deep Learning
- Large Language Models (LLMs)
- Generative Adversarial Networks (GANs)
- Diffusion Models
- Variational Autoencoders (VAEs)
- Ethical AI
- Bias in AI
FAQ
Q: What is Generative AI? A: Generative AI is a form of artificial intelligence that can produce new content such as text, images, videos, and music. It mimics human creativity and intelligence.
Q: How is Generative AI different from Traditional AI? A: While traditional AI focuses on recognizing patterns and making decisions based on pre-existing data, generative AI creates new data and content.
Q: What are some real-world applications of Generative AI? A: Generative AI is used in various fields including creativity (poetry and music), scientific research (modeling biological processes), and business (enhancing customer support and product design).
Q: What are the different types of Generative AI models? A: The main types include Generative Adversarial Networks (GANs), Diffusion Models, and Variational Autoencoders (VAEs).
Q: Why is it important to address bias in Generative AI models? A: Bias in generative AI models can lead to unfair and biased outputs, which can perpetuate social injustices. Addressing bias is crucial for creating fair and ethical AI systems.