How does facial recognition work?
Entertainment
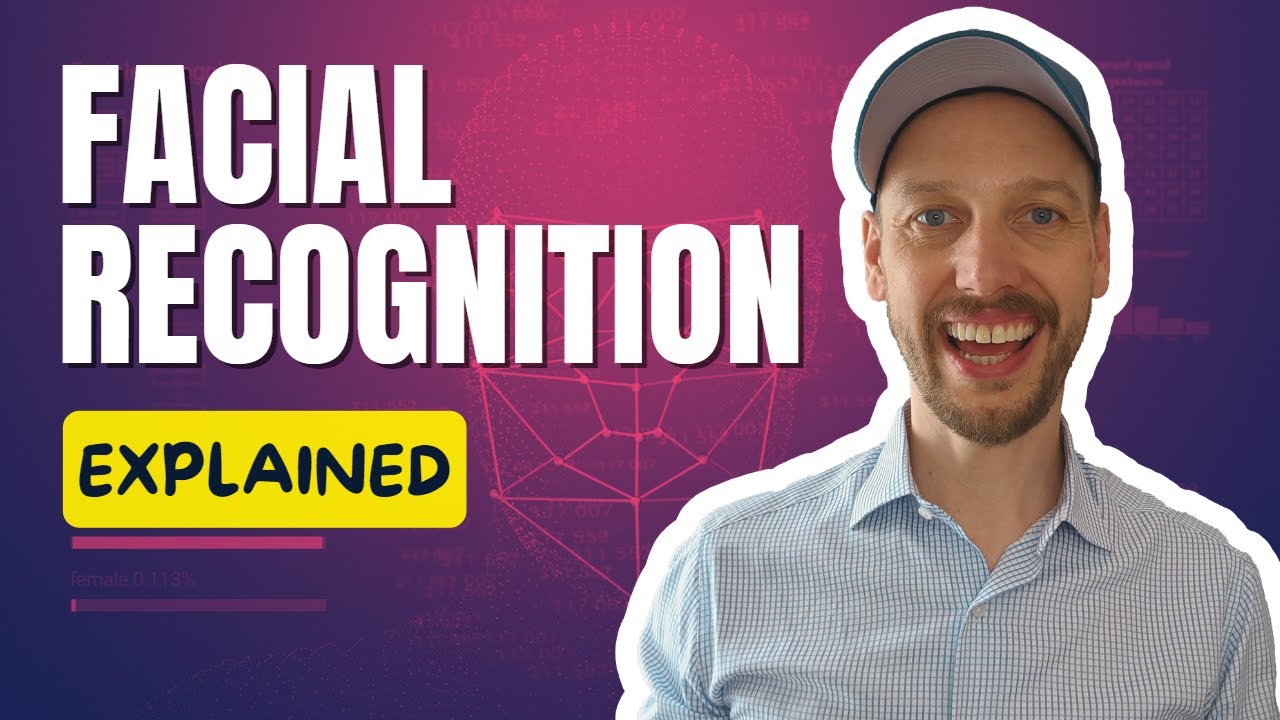
How does facial recognition work?
Hello and welcome, everyone, to another episode of Video Analytics 101. Today, we're delving into facial recognition—a highly complex yet immensely controversial topic. Let's explore the intricate workings behind it.
![Music]
Introduction
Facial recognition technology has been around for many years, and its usage has surged in recent times. We interact with facial recognition daily, whether it's on our phones, during passport control, or for access control, among various other applications. However, it's crucial to understand how this technology works behind the scenes, as there are scenarios where its application may not be ideal. Hence, here is a comprehensive overview.
The Four Fundamental Steps
Facial recognition involves four essential steps: detection, normalization, feature extraction, and matching. Each of these components plays a distinctive role, and understanding them can help us make better choices when deploying the technology.
Detection
The first step is detection, where the camera locates the face within an image. Take, for example, when your camera focuses on a specific face to capture a picture. The system scans the image to identify the regions of interest—in this case, the face.
Normalization
Once the face is detected, we proceed to normalization. This step involves standardizing the face's format to make it comparable with other faces. Faces may vary in distance from the camera, resulting in different resolutions. Different cameras may also have varying color palettes, or even be grayscale. Hence, the face is converted into a common format, often grayscale, and standardized in aspect ratio and resolution. Typically, in deep learning models, the face is converted into a square format, even if it distorts the image slightly.
Feature Extraction
The next step is feature extraction. This is the phase often discussed when explaining facial recognition. Traditionally, it involved measuring distances between facial features like the eyes, nose, and mouth. Nowadays, with deep learning models working as a 'black box,' they autonomously determine the significant points that best describe a facial image. These features are then converted into a string of numbers called a feature vector. This vector essentially serves as a numerical ID for the face in a specific image, allowing for comparison with other feature vectors.
Matching
The final step is matching, where the newly generated feature vector is compared with other stored vectors in a database. The database might contain millions, or even billions, of facial data points. Instead of seeking an identical string of numbers, which is unlikely due to variations in lighting, resolution, and expressions, the system searches for the closest match in the database. This proximity in numerical values indicates that the faces likely belong to the same person.
Verification vs. Identification
An important distinction exists between verification and identification in facial recognition. Though they might appear similar in practice, their technical complexities differ significantly.
Verification
Verification involves one-to-one comparison. A practical example is passport control, where the system compares the passport image with the live image captured by the camera. Essentially, it verifies that the person in front of the camera matches the passport photo. This process is simpler and more mature, with widespread usage at airports and in unlocking devices like phones, where the system knows whose face to expect.
Identification
On the other hand, identification entails a one-to-many comparison, which is considerably more complex. Imagine using facial recognition at a crowded place like Times Square to identify a person among a hundred people. Each person’s face needs to be compared against a large database—say, a database of one million people. This translates to 100 million comparisons per second if there are 100 people to identify, highlighting the significant computational demands of identification versus verification.
Addressing Bias
A final point to consider is bias in facial recognition, a prevalent issue in all machine learning applications but particularly detrimental in this domain. Bias in training data can cause the system to discriminate based on factors like race, gender, age, hair color, or eye color. Therefore, there's a heightened need to mitigate bias in facial recognition systems, a topic we'll explore further in a separate discussion.
Conclusion
In summary, the pipeline of facial recognition involves detection, normalization, feature extraction, and matching, each with its technical significance. Moreover, grasping the differences between verification and identification helps us understand the technology's complexities and limitations. Lastly, addressing bias is critical to ensuring fair and equitable facial recognition applications.
If you have any comments or questions, please leave them here. Don't forget to subscribe for more insightful content, and see you next time!
Keywords
- Facial Recognition
- Detection
- Normalization
- Feature Extraction
- Matching
- Verification
- Identification
- Bias
- Deep Learning Models
- Feature Vector
FAQ
Q: What are the main steps involved in facial recognition?
A: The main steps are detection, normalization, feature extraction, and matching.
Q: What is the difference between verification and identification?
A: Verification involves one-to-one comparison (e.g., passport control or phone unlocking), while identification involves one-to-many comparison (e.g., identifying individuals in a crowd).
Q: Why is bias a concern in facial recognition?
A: Bias can cause the system to unfairly discriminate based on characteristics like race, gender, age, hair color, or eye color, leading to harmful consequences.
Q: What is a feature vector?
A: A feature vector is a string of numbers that uniquely describes a detected face, used for comparing and matching faces in a database.
Q: Why is normalization important in facial recognition?
A: Normalization standardizes the face's format (e.g., converting to grayscale and setting a common resolution), making it comparable with other faces in different conditions and from various sources.