Is Stable Diffusion Actually Better Than Dall-e 2?
Science & Technology
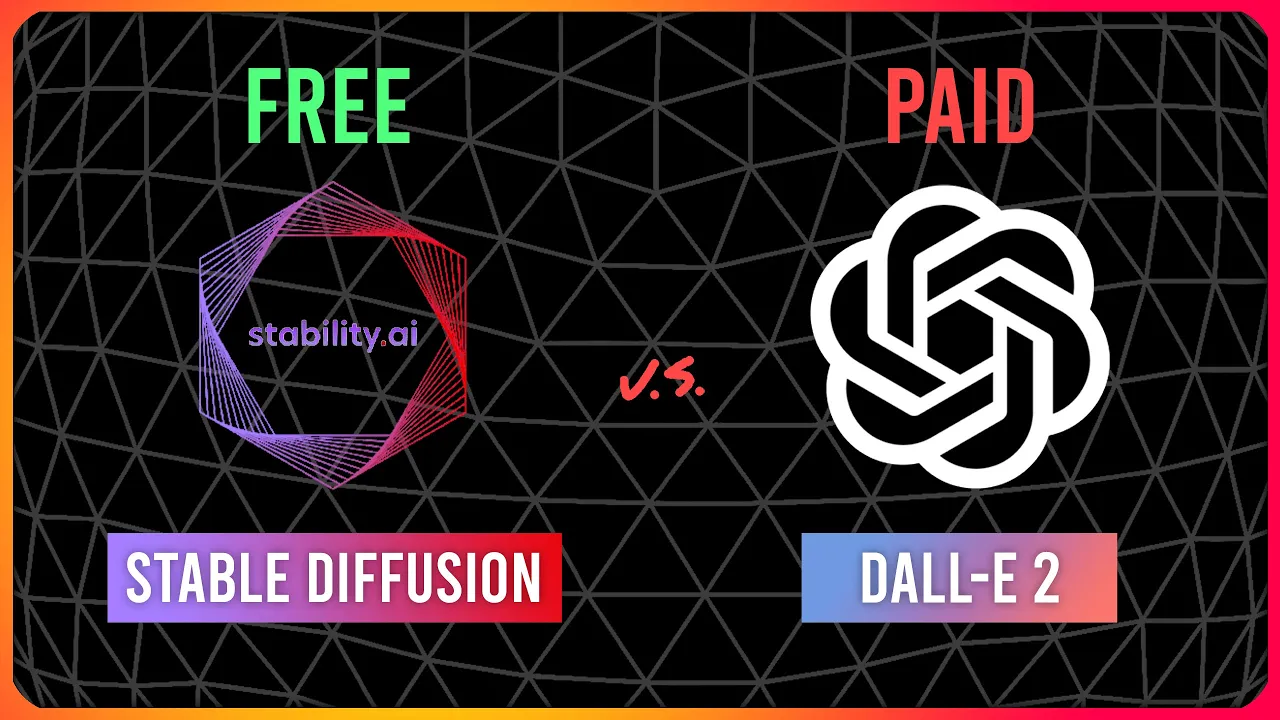
Is Stable Diffusion Actually Better Than Dall-e 2?
Recently, I stumbled upon a hilarious meme on Twitter, contrasting Stable Diffusion with Dall-e 2 concerning text-to-image synthesis. The meme humorously critiqued Dall-e 2's heavy censorship, inadequacy in generating anime-related images, and its non-free nature, while praising Stable Diffusion for being open-source, free, and capable of running on a consumer-grade computer. This piqued my curiosity about the performance differences between the two. Since I haven't made dedicated videos about them, I've organized a comparison of their prompt generations, pros and cons, and what you should expect from each model.
For those not familiar, Stable Diffusion is a project funded by Stability AI and Runway, built upon the research paper "High-Resolution Image Synthesis with Latent Diffusion Models." It was mainly trained on the Leon 5B dataset, which includes 5.85 billion CLIP-filtered image-text pairs, making it the largest open-sourced image-text database. Unlike other models, Stable Diffusion only requires at least 5GB of VRAM and generates images in about 3 seconds, whereas Disco Diffusion requires at least 12GB, and Dall-e 2 likely can't run on a consumer's GPU.
Dall-e 2, also a text-to-image synthesis model, was developed by OpenAI. Although OpenAI hasn't disclosed much about its inner workings, we know that it uses CLIP and diffusion, and was trained on the same dataset as Dall-e 1, which consists of 250 million images. However, I highly suspect a substantial portion of these images are stock photos.
Functions and Implementations
Stable Diffusion, being open-source, allows for intriguing implementations, such as a collage tool that fills a rectangle with a specified prompt or generating videos with unknown techniques but visually impressive results. Another exciting development is a text-to-video editing tool in Runway's editing app. The possibilities are endless, and it can adapt to whatever Dall-e 2 offers.
In contrast, Dall-e 2's text-to-image synthesis contains relatively limited functions since it's not open-source. It can generate variations of an image or use an in-painting tool, which allows users to edit parts of an image based on a prompt. Dall-e 2 also has a popular cropping function that can expand an image's borders, making it seem like you're zooming out of the frame. Despite not having access to Dall-e 2,giving a tour of Dall-e 2's functions.
Comparing Results
When comparing Stable Diffusion and Dall-e 2 regarding prompt text, both struggle with long, specific prompts and counting but Dall-e 2 excels at generating hyper-specific details. Stable Diffusion sometimes misinterprets prompts when faced with opposing concepts, while Dall-e 2 can create coherent images even in these scenarios.
Despite this, Stable Diffusion's images often turn out aesthetically pleasing compared to Dall-e 2's dull and plain results if not provided with enough modifiers. For instance, when generating book covers, Stable Diffusion produces creative and colorful images, while Dall-e 2 results in lackluster images resembling stock photos.
Creativity and Dataset
Dall-e 2 sometimes avoids generating clear, accurate faces, especially of famous people, likely due to its censorship policies. In contrast, Stable Diffusion excels in generating faces, particularly in anime and japan-themed images. Dall-e 2 struggles in this area due to a lack of anime-related data in its dataset.
Moreover, Dall-e 2 has issues with keywords like "3D" or "8K," resulting in poorly lighted images, likely indicating its training on stock images. In general, Dall-e 2's adherence to stock image styles is evident when generating simple images.
In conclusion, both models have their strengths, but free tools like Stable Diffusion tend to have an edge over paid alternatives like Dall-e 2.
Big shout out to Andrew Leschelius, Chris Ledoux, Dan Kennedy, and others supporting me through Patreon or YouTube. If you like my videos, you already know what to do.
Keywords
- Stable Diffusion
- Dall-e 2
- Text-to-image synthesis
- Open source
- Anime images
- Image generation
- AI models
- Prompt text
- Dataset
- CLIP
- Diffusion
- Runway
- Stock images
FAQ
What is Stable Diffusion?
Stable Diffusion is an open-source project funded by Stability AI and Runway, built upon the research paper "High-Resolution Image Synthesis with Latent Diffusion Models”. It is designed for text-to-image synthesis and can run on consumer-grade hardware.
How does Dall-e 2 differ from Stable Diffusion?
Dall-e 2 is developed by OpenAI and is not open-source. It also requires more specialized hardware to run effectively compared to Stable Diffusion. Dall-e 2 excels at generating hyper-specific details and maintaining coherence in complex prompts.
Why is Stable Diffusion considered better for anime-related images?
Stable Diffusion's dataset includes a variety of anime-related images, making it more effective at generating anime-style art. In contrast, Dall-e 2's dataset lacks substantial anime content.
What are some unique features of Stable Diffusion?
Stable Diffusion's open-source nature allows for creative implementations such as collage tools, text-to-video editing, and various customizations by the community.
Why are Dall-e 2's images sometimes dull and plain?
Dall-e 2 may generate dull images when not provided with detailed modifiers, likely due to a portion of its training dataset consisting of stock images.
Can both models generate faces accurately?
Stable Diffusion is more adept at generating accurate faces, including famous personalities. Dall-e 2 may avoid generating clear faces, possibly due to its censorship policies.
Are there any tools for video generation with these models?
Yes, Stable Diffusion has been used for creating videos, and there is a text-to-video editing tool in development for Runway's editing app. Dall-e 2 also has cropping functionalities that can create zoom-in and zoom-out effects in videos.