Knowledge Graphs are key to unlocking the power of AI
Science & Technology
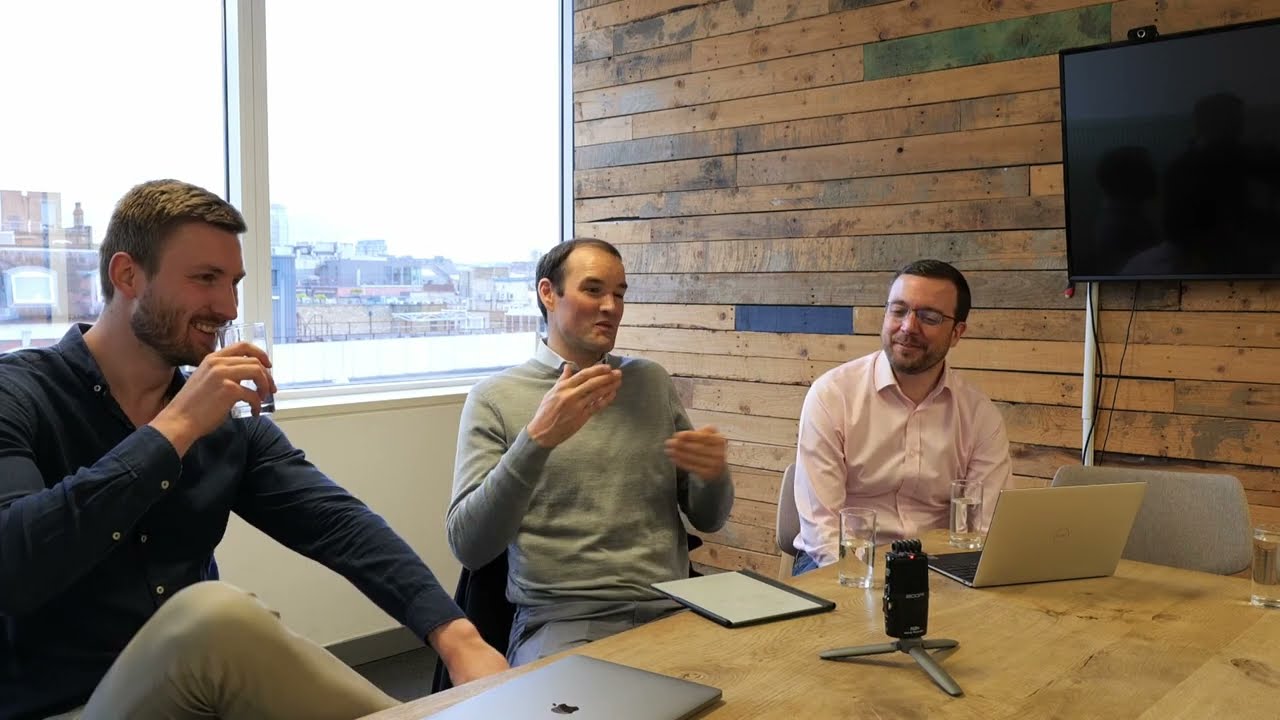
Introduction
In a recent episode of "Architect Tomorrow," a panel discussion centered around the advances in machine learning, specifically focusing on generative AI and the critical role of knowledge graphs. The conversation involved panelists Chris Booth and Tony Seale, who shared their expertise and insights into how knowledge graph technology can integrate with generative AI within enterprise architecture.
Introduction to Knowledge Graphs
Knowledge graphs provide a way to structure data differently from traditional methods like relational databases or spreadsheets. In contrast to these linear structures, knowledge graphs utilize a graph format consisting of nodes (entities) connected by edges (relationships). By employing triples—composed of subjects, predicates, and objects—knowledge graphs can represent complex relationships effectively. For example, one might represent “John has blue eyes” (subject-predicate-object).
Tony Seale emphasized that the concepts behind knowledge graphs have evolved since their introduction. He noted that Google's Knowledge Graph, introduced in 2012, transformed how data is accessed during a search, integrating contextual information directly into search results. The term "semantic web" and its approaches have transitioned over time into what we now identify as knowledge graphs.
The Value of JSON-LD
The discussion also highlighted JSON-LD, a lightweight Linked Data format that allows for simple representation of structured data across various platforms. It enables developers to easily incorporate knowledge graphs into their applications without the steep learning curve associated with traditional graph databases.
Furthermore, this method allows for decentralized data distribution, meaning organizations can maintain functional knowledge graphs spread across cloud services without centralizing all their data. This is especially crucial in maintaining operational efficiency and scalability in enterprise systems.
Practical Applications of Knowledge Graphs and AI
The panelists discussed a proof-of-concept project that illustrated how knowledge graphs could enhance generative AI applications in highly regulated sectors, such as finance. By using knowledge graphs to manage contextual data and questions during customer interactions, organizations can create structured dialogues that fulfill specific objectives while minimizing risks associated with misinformation.
A significant insight from the conversation was the importance of using established ontologies to organize an organization’s data. By aligning vocabulary and concepts with schema.org—a widely adopted ontology—businesses can create a unified and more uniform structure for their data. This not only aids in information extraction but increases interoperability and reduces ambiguity across different business units.
The Future: Data Consolidation and AI
Both Chris and Tony emphasized the need for organizations to consolidate their data. They argued that many businesses sit atop valuable data treasures but struggle to harness them effectively due to disorganization. The introduction of generative AI presents an opportunity for organizations to reevaluate their data management strategies seriously.
As organizations navigate the rapidly evolving AI landscape, success will depend on their ability to organize data efficiently and cultivate a collaborative environment where skilled individuals align their goals—even when faced with inherent political and cultural challenges.
Conclusion
In summary, the combination of knowledge graphs and generative AI can unlock unprecedented potential for businesses, allowing them to draw insights from their data more effectively while enhancing customer interactions. However, the crux of this transformation lies in establishing a robust data structure, applying best practices, and most importantly, fostering a culture that prioritizes data stewardship.
Keyword
Knowledge Graphs, AI, Generative AI, Semantic Web, JSON-LD, Ontologies, Data Consolidation, Enterprise Architecture, Machine Learning, Interoperability.
FAQ
Q1: What is a knowledge graph?
A knowledge graph is a way of structuring data using nodes and edges to represent entities and their relationships, allowing for complex data interconnections.
Q2: How does JSON-LD relate to knowledge graphs?
JSON-LD is a format that allows for the easy representation of linked data in a familiar JSON structure, making it accessible for developers and enabling data integration with knowledge graphs.
Q3: What role do ontologies play in knowledge graphs?
Ontologies provide a structured framework for categorizing and defining relationships within data, ensuring that different data elements are correctly understood and interoperable across various systems.
Q4: Why is data consolidation important for businesses?
Data consolidation helps organizations to organize their information systematically, make better use of their data assets, and enhance their AI capabilities, leading to improved decision-making and operational efficiency.
Q5: What challenges do organizations face when implementing knowledge graphs?
Organizations often encounter political and cultural hurdles, as aligning different business units' definitions and data structures can be a complex and delicate process.