Large Language Models Are Zero Shot Reasoners
Education
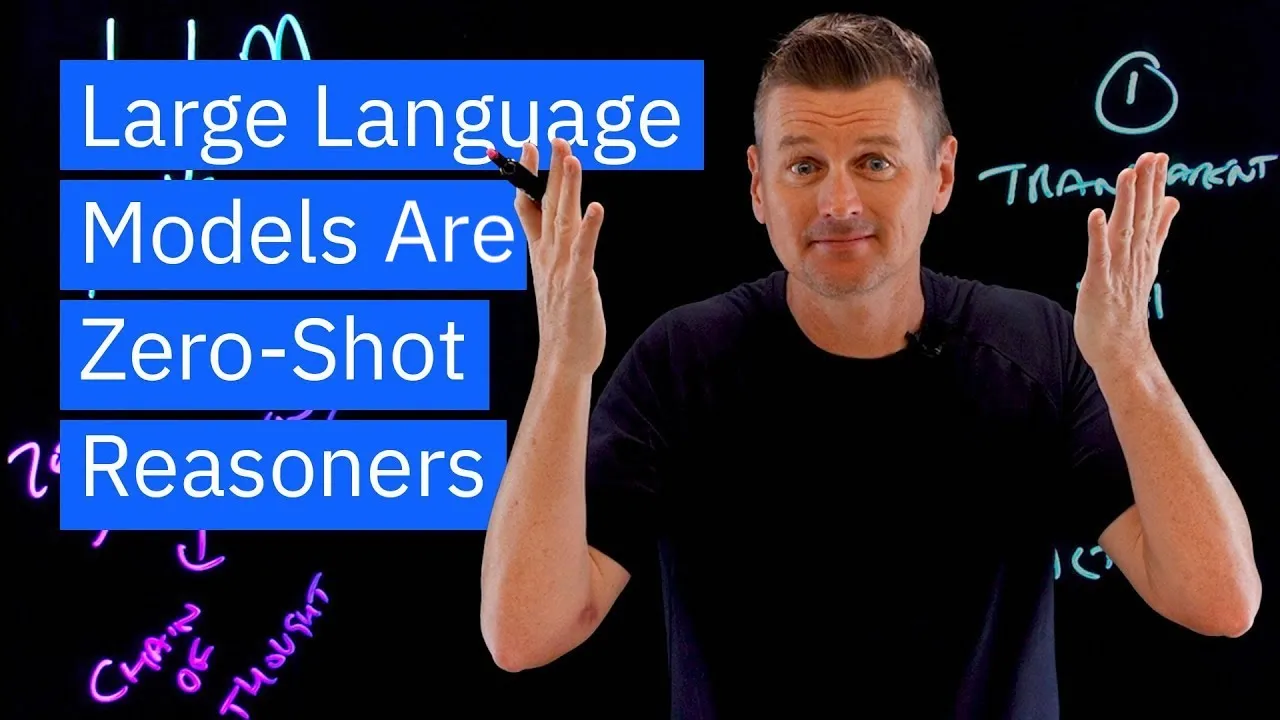
Introduction
If you're not getting the responses you want from AI language models like GPT, it might be due to how you're prompting them. This article discusses the importance of prompting, the differences between zero shot and few shot prompting, and the use of chain of thought prompting to improve the reasoning abilities of large language models.
The way we prompt large language models plays a significant role in the quality of the responses they generate. Zero shot prompting involves providing a single question or instruction without additional context, which can sometimes lead to sub-optimal responses. Few shot prompting, on the other hand, includes providing examples to guide the model's understanding and improve the context of the prompt.
Chain of thought prompting is a technique that encourages the model to document its thinking process, leading to more detailed and transparent responses. This type of prompting can improve the quality of the model's answers by encouraging it to consider alternative perspectives and different approaches.
Keywords: Large Language Models, Zero Shot Prompting, Few Shot Prompting, Chain of Thought Prompting, Reasoning Abilities.
FAQ
Q: What is zero shot prompting?
A: Zero shot prompting involves providing a single question or instruction without additional context to a large language model, relying solely on the model's pre-existing knowledge to generate a response.
Q: How does few shot prompting improve responses from large language models?
A: Few shot prompting provides examples to guide the model's understanding and improve the context of the prompt, helping the model generate more accurate and relevant responses.
Q: What is chain of thought prompting, and how does it benefit large language models?
A: Chain of thought prompting encourages the model to document its thinking process, leading to more detailed and transparent responses, and helping the model consider alternative perspectives for more comprehensive answers.