Large Language Models (LLMs) vs Natural Language Understanding (NLU)
Science & Technology
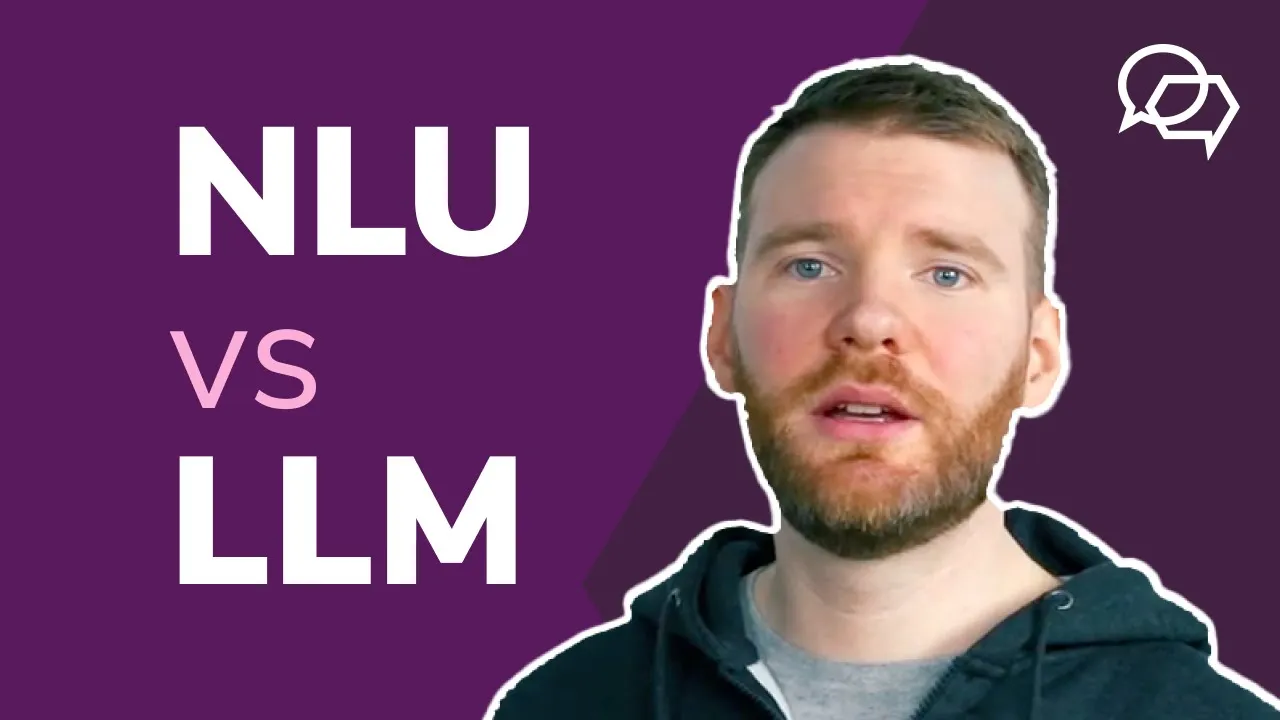
Introduction
We often face the question of why we still need Natural Language Understanding (NLU) when we have Large Language Models (LLMs). From our perspective, it's not about choosing one over the other as both NLU and LLM are tools to process language. However, they have different purposes, strengths, and weaknesses. For enterprises, understanding inquiries in a business-to-consumer context and providing accurate responses to build trust are crucial. Choosing the right tool for the job is essential.
An LLM is trained on vast amounts of general knowledge and excels at text generation. However, it lacks the ability to fully understand context and can give inaccurate responses. On the other hand, NLU makes chatbots subject matter experts by focusing on specific subjects, ensuring precise answers based on context. Combining LLM and NLU can maximize opportunities while minimizing risks.
Keywords:
LLMs, NLU, language processing, chatbots, trust, accuracy, context understanding, text generation, subject matter expert
FAQ:
Why do we still need NLU when we have large language models like LLMS?
- NLU and LLMS serve different purposes and have distinct strengths and weaknesses. NLU ensures precise and context-aware responses, which are crucial for building trust in a business-to-consumer context.
How do LLMS and NLU complement each other in language processing?
- LLMS are excellent tools for generating text efficiently, while NLU focuses on understanding specific subjects to provide accurate answers. Combining both tools enhances the effectiveness of chatbots in processing language.
What are the downsides of relying solely on LLMS for language processing?
- LLMS lack the ability to fully comprehend context, leading to inaccurate or false responses. They may generate answers based on vast training data without considering context-specific information.