Live Object Detection in Python
Science & Technology
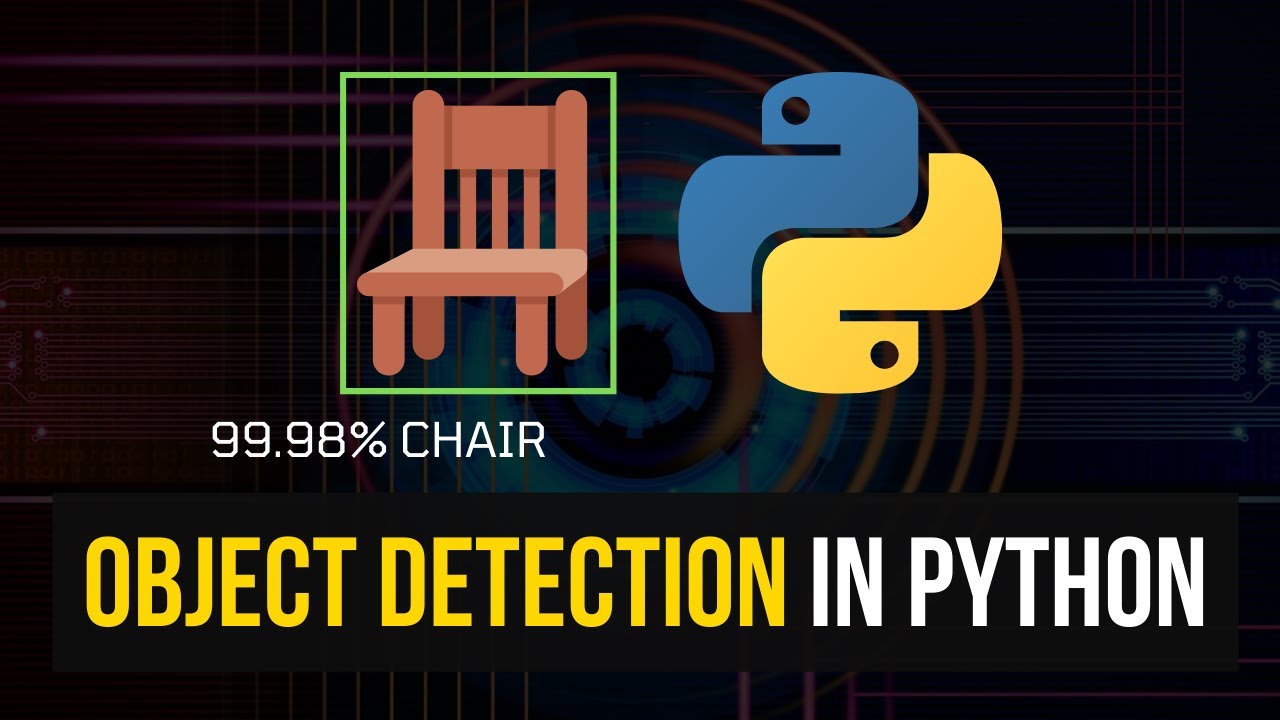
Introduction
In this article, we will implement an object recognition tool using Python. The goal is to leverage a pre-trained model for detecting objects within images or live camera feed. We will utilize OpenCV and a MobileNet SSD model to achieve this task. Let's get started!
Setting Up the Project Structure
To begin, we need to set up the project structure. This involves downloading a couple of external files, specifically a pre-trained model that we'll employ for object recognition. Here’s a step-by-step guide:
Download the Model: Head over to GitHub repository that hosts the MobileNet SSD model. There, you will find a
Caffe
model and aprototxt
file. Download these and place them in a directory namedmodels
within your working directory.Prepare Images: Gather some images for object detection. These images may contain objects like people, cars, or furniture. For this example, we will include images featuring rooms or streets.
Install Required Libraries: You will need to install the following python libraries:
pip install numpy pip install opencv-python
Writing the Code
Import Libraries: Import NumPy for numerical operations and OpenCV for image processing.
import numpy as np import cv2
Specify Paths: Define paths for the model files and the image you wish to analyze.
image_path = 'room_people.jpg' # Path to the image proto_txt_path = 'models/MobileNetSSD_deploy.prototxt' model_path = 'models/MobileNetSSD_deploy.caffemodel' min_confidence = 0.2 # Minimum confidence threshold for detection
List of Classes: Create a list of class labels that the model can identify.
classes = [ "background", "aeroplane", "bicycle", "bird", "boat", "bottle", "bus", "car", "cat", "chair", "cow", "dining table", "dog", "horse", "motorbike", "person", "potted plant", "sheep", "sofa", "train", "tvmonitor" ]
Generate Random Colors for Bounding Boxes: To classify the detected objects with different colors, we can utilize random colors.
np.random.seed(54321) colors = np.random.uniform(0, 255, size=(len(classes), 3))
Load Model: Load the pre-trained model using OpenCV’s DNN module.
net = cv2.dnn.readNetFromCaffe(proto_txt_path, model_path)
Image Processing: Load and prepare the image:
image = cv2.imread(image_path) (height, width) = image.shape[:2]
Process the image for feeding it to the model:
blob = cv2.dnn.blobFromImage(cv2.resize(image, (300, 300)), 0.007843, (300, 300), 130) net.setInput(blob) detected_objects = net.forward()
Draw Predictions: Iterate over the detected objects to draw rectangles around them with their predicted class names.
for i in range(detected_objects.shape[2]): confidence = detected_objects[0, 0, i, 2] if confidence > min_confidence: class_index = int(detected_objects[0, 0, i, 1]) (upper_left_x, upper_left_y) = (int(detected_objects[0, 0, i, 3] * width), int(detected_objects[0, 0, i, 4] * height)) (lower_right_x, lower_right_y) = (int(detected_objects[0, 0, i, 5] * width), int(detected_objects[0, 0, i, 6] * height)) # Draw rectangle and label on the image cv2.rectangle(image, (upper_left_x, upper_left_y), (lower_right_x, lower_right_y), colors[class_index], 2) text = f"(classes[class_index]): (confidence:.2f)" cv2.putText(image, text, (upper_left_x, upper_left_y - 15), cv2.FONT_HERSHEY_SIMPLEX, 0.5, colors[class_index], 2)
Display the Image: Finally, display the resulting image with the detected objects.
cv2.imshow("Output", image) cv2.waitKey(0) cv2.destroyAllWindows()
Live Video Feed
To detect objects in a live camera feed, replace the image-loading code with a video capture stream. Here’s how to achieve that:
cap = cv2.VideoCapture(0)
while True:
ret, frame = cap.read()
if not ret:
break
# Process frame as done previously...
cv2.imshow("Live Detection", frame)
if cv2.waitKey(1) & 0xFF == ord('q'):
break
cap.release()
cv2.destroyAllWindows()
Now, as you run this code, the program will utilize your webcam to detect objects in real-time!
Conclusion
This article demonstrated how to perform object detection in Python using a pre-trained MobileNet SSD model. We emphasized the steps to set up the project structure, write necessary code, and deploy the model both on static images and live video feed.
Keyword
- Python
- Object Detection
- OpenCV
- MobileNet SSD
- Pre-trained Model
- Real-Time Detection
FAQ
Q1: What libraries do I need to install for object detection?
A1: You need to install NumPy and OpenCV. Use the following commands:
pip install numpy
pip install opencv-python
Q2: Where can I find the MobileNet SSD model?
A2: You can download it from the GitHub repository here.
Q3: How can I modify the minimum confidence threshold?
A3: You can modify the value of the min_confidence
variable in your code to adjust sensitivity for object detection.
Q4: Can I use my webcam for live object detection?
A4: Yes! The code example in the article shows how to implement live object detection using your webcam feed.
Q5: How does the random color assignment work?
A5: Random colors are generated using NumPy. This helps to represent different detected classes visually. If you want consistent colors across runs, set a seed with NumPy.