Measuring success as an AI Product Manager #ai #productmanagement
Entertainment
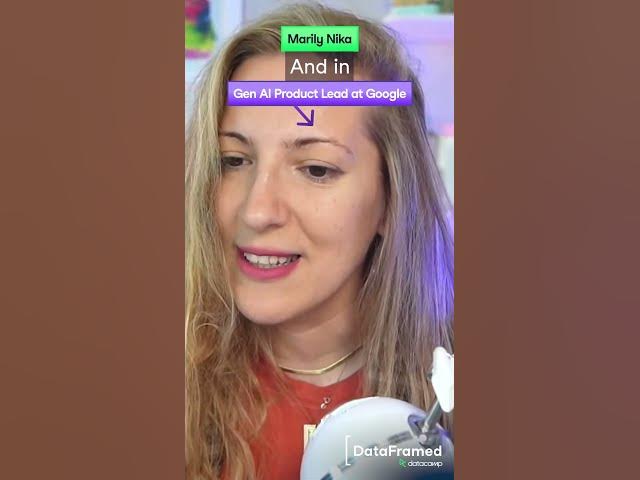
Introduction
In a traditional product management setting, success is often defined by the number of users a product can attract. The approach is linear: execute a series of tasks, such as completing steps A, B, and C, to achieve a desired outcome—let's say, Y. However, for teams that are accustomed to this method, the shift to an AI context can be disorienting. Such teams may find themselves feeling demotivated when the linear path becomes murky, leading to uncertainty about their progress and career advancements.
In an AI product management landscape, it is crucial to foster a culture of experimentation and exploration not only among engineers but also with stakeholders. The nature of AI projects often involves iterating hypotheses and refining concepts. As a product manager, one of the primary tasks is to redefine what success looks like.
For instance, rather than simply celebrating the launch of a product that garners hundreds or thousands of users, an AI product manager might consider success as follows: "We formulated hypothesis A, rigorously tested it, and ultimately concluded that it needed to be pivoted." This shift in perspective transforms success into a series of milestone-based achievements. Each hypothesis tested, regardless of the outcome, represents valuable progress and contributes to the team's overall learning and future trajectory.
Emphasizing a mindset of experimentation allows teams to embrace failures as stepping stones rather than setbacks. In the dynamic field of AI, learning and adapting is key, and understanding that not every hypothesis will yield a positive answer is part of the journey.
In summary, as an AI product manager, redefining success away from traditional metrics towards a more iterative and learning-focused approach is essential. This not only helps in navigating the complexities of AI products but also keeps the team motivated, involved, and aligned on the pathway to real innovation.
Keywords
- AI Product Management
- Success Metrics
- Experimentation Culture
- Hypothesis Testing
- Iterative Learning
- Milestone-based Progress
- Team Motivation
FAQ
Q: How does success differ between traditional product management and AI product management?
A: In traditional product management, success is often measured by user numbers post-launch. In AI product management, success is redefined as the ability to test hypotheses and learn from the outcomes, whether they lead to success or require pivoting.
Q: Why is a culture of experimentation important in AI product management?
A: A culture of experimentation allows teams to explore new ideas and learn from both failures and successes. It keeps team members motivated and engaged, as they understand that not every hypothesis needs to yield a positive result to be considered valuable.
Q: What should AI product managers focus on to maintain team motivation?
A: AI product managers should focus on creating clear milestones based on hypothesis testing and iterated learning rather than just linear outcomes. This approach helps teams understand their progress and fosters a sense of achievement in the exploration process.
Q: How can failures be reframed as part of success in AI product management?
A: Failures in testing hypotheses can be viewed as learning opportunities. Each failure provides insights that can inform future experiments and strategies, ultimately contributing to the team's growth and innovation.