"Model Validation in AI: Ensuring Accurate Results | Product Management Insights"
Education
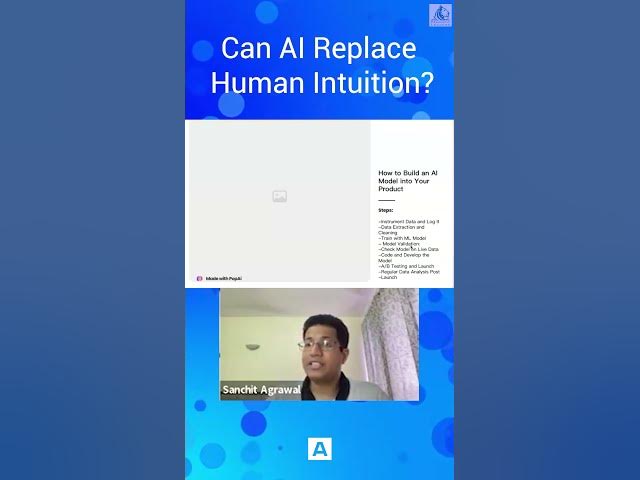
Introduction
Model validation is a crucial step in the artificial intelligence (AI) development process. Once a model has been trained on a dataset, it is imperative to assess its accuracy and reliability by running a validation phase. This process involves comparing the model's outputs to historical data within the same business scenario. By doing so, you can determine how the model’s predictions differ from previous results and the impact of those variations.
During the model validation phase, it's essential to evaluate the performance of the AI model against existing metrics. This includes delving into the significance of various features within the dataset. For example, factors like distance and cost can significantly influence model outcomes. By analyzing these variables, practitioners can assess whether certain features are unduly influencing the results or if their importance is not being properly recognized by the model.
Moreover, the model validation phase is instrumental in identifying and rectifying any biases or inaccuracies that may have emerged during the training phase. It's a meticulous process that involves manual scrutiny of the AI outputs—essentially a thorough "eyeball check." Unlike the training phase, which leverages AI algorithms for predictive capabilities, model validation requires human oversight to ensure that the data model operates optimally and fairly.
In summary, model validation encompasses a series of steps designed to guarantee that the AI model is generating accurate and reliable predictions. This involves comparing past scenarios, evaluating the influence of individual features, and manually checking for flaws or discrepancies in the model outputs.
Keywords
- Model Validation
- AI
- Accuracy
- Reliability
- Historical Data
- Business Scenario
- Feature Importance
- Bias
- Manual Check
- Data Model
FAQ
What is model validation in AI?
Model validation is the process of evaluating an AI model's outputs against historical data and existing metrics to ensure its accuracy and reliability.
Why is model validation important?
It is crucial for confirming that the AI model produces trustworthy and meaningful results, which can impact business decisions.
How is model validation conducted?
Model validation involves testing the model's predictions with past scenarios, adjusting the significance of various input features, and manually inspecting the output for any inconsistencies or biases.
What role do features like distance and cost play in model validation?
These features can significantly impact the model's predictions, so it is important to assess their influence and ensure they are properly accounted for in the model.
Is AI used during the model validation phase?
While AI is utilized during the training phase, model validation relies on human oversight to critically analyze outputs and ensure the model's effectiveness.