NODES 2024 - Keynote: Tracking the Pulse of Generative AI (APAC)
Entertainment
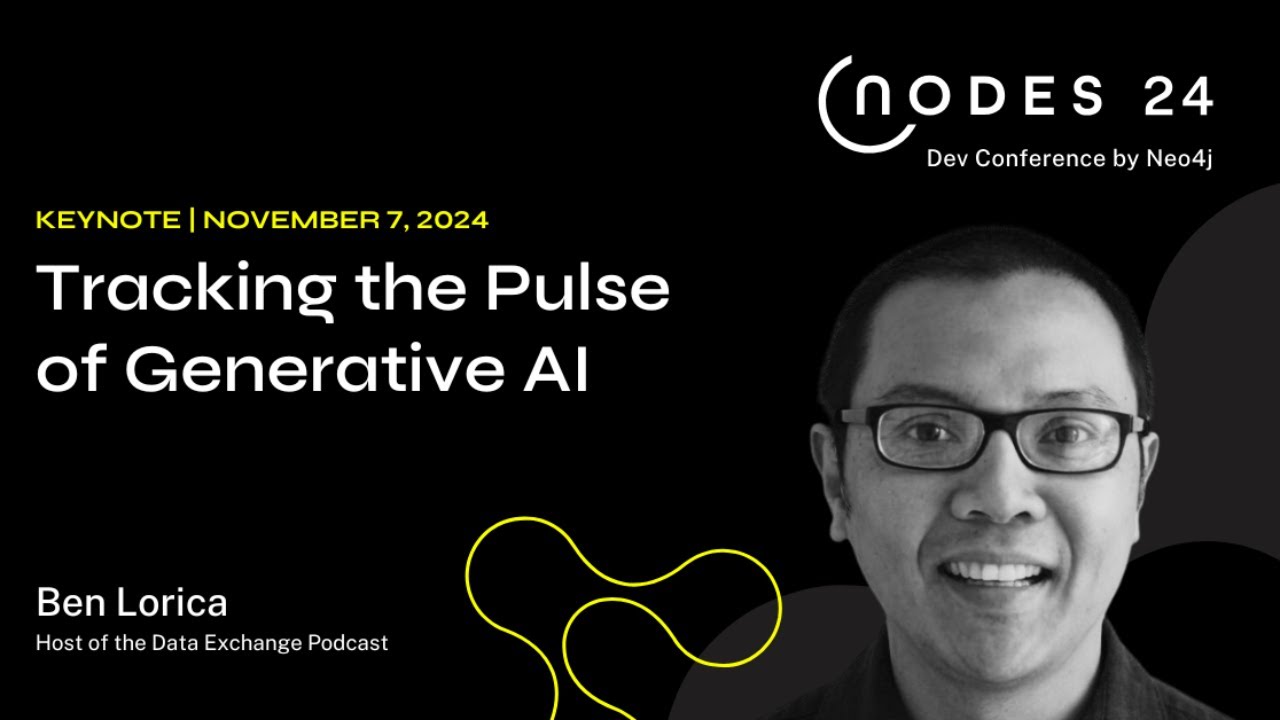
Introduction
Thank you to the organizers at S4J for inviting me to keynote at what promises to be an exciting conference. Today, I will take you on a rapid tour through the most interesting developments in generative AI. This discussion will be informed by my experience as an advisor and investor in numerous leading companies, alongside serving as a program chair in several prominent industry conferences.
Foundation Models and Large Language Models
To start, let’s discuss foundation models—the large language models (LLMs) that have become integral to our daily tasks. I checked this morning, and I currently have easy access to over 30 models, although I primarily use a handful from OpenAI, Anthropic, Gemini, and Llama.
The key takeaway from discussions with model builders is their continued focus on model size. They are guided by what are known as scaling laws, which indicate that larger models generally perform better as you increase their size, training data, and compute. This means that models considered cutting-edge today will only grow larger, further increasing the cost associated with training them.
Despite a significant revenue stream and impressive growth, raising model prices may be challenging due to the availability of alternatives—if one service encounters an outage, users can easily shift to another provider. Thus, for startups in this space, the constant need for funding at escalating valuations may pose a significant challenge amid fierce competition.
Adoption of Generative AI
In brighter news, there’s strong adoption of generative AI across various sectors. Earlier this year, we conducted a survey among healthcare professionals that revealed over a third planned to double their generative AI budgets, while nearly half intended to increase theirs by at least 50%. Content creation remains the most popular application, yet the use of generative AI as coding assistants has become increasingly widespread among developers.
Moving forward, I want to focus on some main challenges AI teams face. In a comparative study between the hype cycles of big data and generative AI, I have concluded that we have not yet reached the peak of the hype with generative AI. There remains untapped potential as we progress.
Despite widespread usage, many teams report facing bottlenecks related to data management and infrastructure challenges. Our healthcare survey noted that nearly half of respondents use multiple models, with this number likely having risen since our data collection. As models improve through additional training, fine-tuning options are becoming more important.
Post-training strategies will be crucial, enabling teams to refine pre-existing models rather than starting from scratch, which is exorbitantly expensive. Techniques such as reinforcement learning, fine-tuning, and model optimization are essential for maintaining a competitive edge.
Data Strategy in Generative AI
Regarding data, it’s crucial to differentiate between training data required for initial model training (which most will not need) and the fine-tuning datasets necessary for optimizing applications. Quality still matters, even with large models, and attention should be given to the pre-training data’s quality.
Graphs can play a key role in managing data quality, especially in generating high-quality datasets for training and fine-tuning. As interest grows in synthetic data’s role in AI, it should be noted that it must serve as a supplement, not a complete replacement for organic, human-generated data.
I also want to discuss retrieval-augmented generation (RAG) and its potential. The RAG community is active, and while numerous companies offer RAG as a service, teams often choose to build their systems. This necessitates strategies to optimize the (often computationally intensive) setup of RAG applications.
The Prominent Role of Agents
Now, let’s dive into the rise of AI agents, which are becoming increasingly capable of making decisions and interacting with environments with minimal human input. However, it’s critical to utilize agents for well-defined tasks. The technology stacks we see emerging for these agents are varied, and there’s still exploration needed to determine best practices and effective frameworks for building them.
In terms of application, analysts and mathematicians are actively leveraging large language models in their workflows. Analysts employ LLMs throughout their tasks, from ideation through reporting, whereas mathematicians use them as assistant tools, mostly for ideation or translating complex concepts into programming languages.
Infrastructure Trends
As the market evolves, custom AI platforms are becoming more common among tech companies that want tailored solutions. Most prefer this route to accommodate their unique needs, workflows, and data systems while also managing costs effectively.
One prominent technology supporting these custom platforms is Ray, an open-source distributed computing framework that many companies are beginning to adopt for AI deployment.
The Future of Generative AI
As generative AI keeps developing, I am particularly interested in the move toward multimodal understanding—where models can genuinely grasp video content and engage with it interactively. Though the technology has advanced significantly, there remains much ground to cover.
Overall, the risk management aspect of AI needs serious attention as many teams lack incident response plans and structured approaches. Collaboration across departments—such as technical, product, marketing, and legal—will be essential for efficient risk assessment and management.
Conclusion
To wrap up, several takeaways stand out:
Design for Flexibility: Ensure your application can switch model providers readily and can incorporate multiple models.
Post-Training Capacity: Invest in infrastructure that enables effective post-training on models.
Data Strategy: Develop a robust data management strategy for handling large amounts of unstructured data, possibly leveraging graphs for improved quality.
Scale Matters: Utilize computational resources effectively to optimize your applications.
Risk Management: Prioritize the development of AI incident response plans and ensure collaboration across teams.
In conclusion, I believe the future is bright for generative AI, and the potential for advancement remains significant. Thank you for your attention, and if you'd like to follow my ongoing work, subscribe to my newsletter at Gradient Flow on Substack.
Keywords
- Generative AI
- Foundation Models
- Large Language Models (LLMs)
- Model Size
- Adoption
- Healthcare
- Post-training
- Data Quality
- Retrieval-Augmented Generation (RAG)
- AI Agents
- Infrastructure
- Multimodal Understanding
- Risk Management
FAQ
What are foundation models? Foundation models are large-scale AI models trained on a vast amount of data and can perform various tasks, especially in the realm of natural language processing.
What is post-training in the context of AI? Post-training refers to refining and optimizing pre-trained models for specific tasks, rather than training models from scratch, which can be costly.
How is data quality significant in generative AI? High-quality data is crucial for the success of both fine-tuning and training data for generative models. Poor data can lead to ineffective or biased AI outputs.
What are agents in AI? Agents are advanced AI systems capable of making decisions and interacting with their environments with minimal human input.
Why is risk management important in AI? Managing risks is essential to ensure that AI systems behave correctly, ethically, and comply with regulations, reducing the likelihood of negative outcomes.