NODES 2024 - Speaker Roundtable
Entertainment
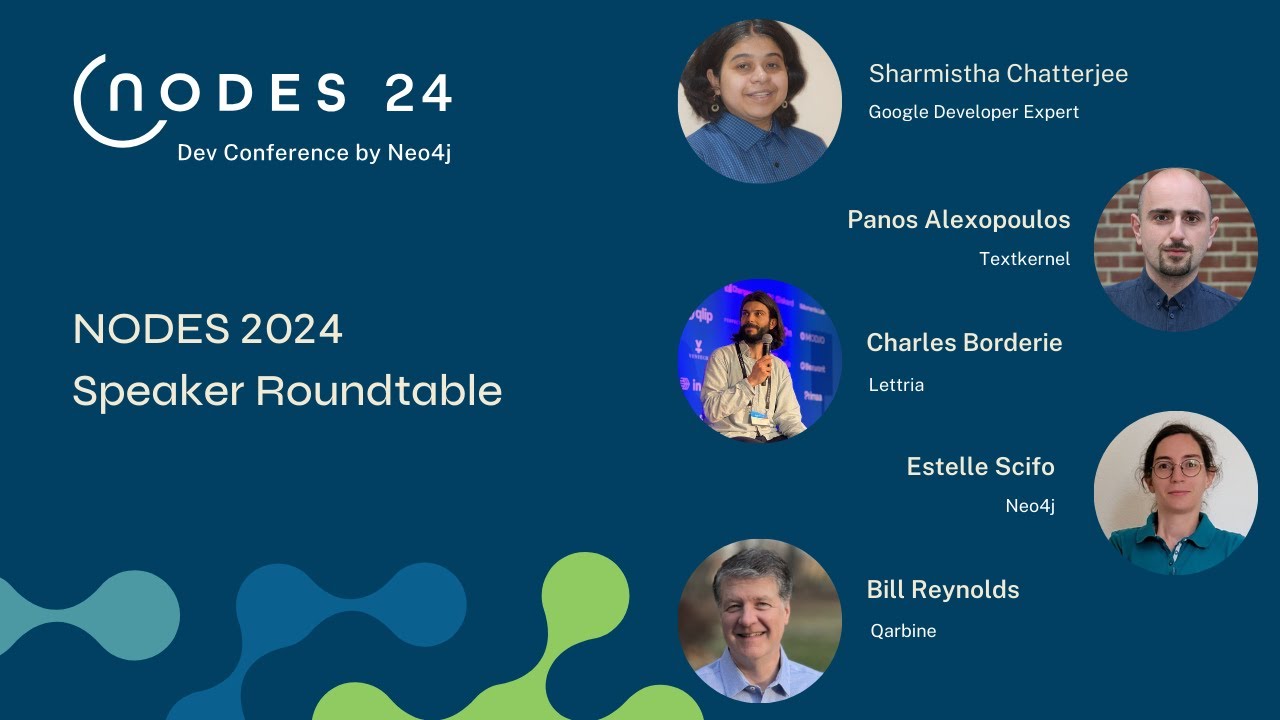
Introduction
As we gear up for NODES 2024, taking place next Thursday, November 7th, this article provides a sneak peek into the discussions and insights from our esteemed panel of speakers. The event promises to be rich in content, featuring key themes related to graph technology, AI, and knowledge graphs.
The speakers at this roundtable included Sh chy, a co-author focused on responsible AI and Google Developer Expert; Panos Alexopulos, a data professional at Textkernel; Charles Bord, CEO of Electrify; Estel Cifo, a machine learning engineer at Neo4j; and Bill Reynolds, the Chief Technology Officer at Carbine.
Highlights from the Discussion
Neo4j and Graph Technology Neo4j is entering a transformative era with its advancements in graph technology, particularly with the release of Neo4j version 5. This version boasts enhanced performance, unbounded scalability, and operational agility, allowing organizations to manage connected data with unprecedented speed and efficiency.
Generative AI and Knowledge Graphs With the integration of Neo4j and large language models (LLMs), the possibilities for leveraging generative AI have expanded significantly. By using LLMs with Neo4j's graph technology, organizations can reduce hallucinations, enhance explainability, and ensure transparency in AI outputs. The combination of deterministic facts from the graph and probabilistic conclusions from LLMs sets a new standard in the field.
Use Cases and Real-Life Applications The discussion touched upon several real-world applications where graph data plays a crucial role. In healthcare, for example, patient journeys can be effectively tracked using knowledge graphs, which provide a holistic view of patient data and interactions. This can lead to timely interventions and improved patient care outcomes.
Similarly, in the financial domain, organizations can leverage knowledge graphs to enhance decision-making processes, allowing finance professionals to derive insights from complex datasets.
Addressing Bias and Fairness A key topic explored was the importance of fairness and the potential biases present in both AI and knowledge graphs. The panelists stressed the importance of incorporating mechanisms to measure fairness and reduce bias, especially when dealing with sensitive data like patient information.
Performance Insights Estel shared insights from their upcoming session on measuring the performance of vector RAG (retrieval-augmented generation) against graph RAG. Their findings suggest that graph RAG consistently yields superior results due to its ability to leverage relationships within the data.
Panos emphasized the importance of understanding the intricacies of both RDF (Resource Description Framework) and property graphs to facilitate effective data modeling, lingering on the need for interoperability between semantic models.
Conclusion
NODES 2024 is set to be an enriching experience for anyone interested in exploring new frontiers of graph technology, AI, and knowledge graphs. Attendees can expect engaging discussions, live demos, and the chance to delve into the future of connected data.
Keywords
Neo4j, graph technology, generative AI, knowledge graphs, performance measurement, fairness, bias, vector RAG, graph RAG, healthcare, finance, data modeling.
FAQ
1. What is Neo4j? Neo4j is a leading graph database technology that allows users to visualize and manage complex data relationships efficiently.
2. What are knowledge graphs? A knowledge graph is a structured representation of data that captures relationships between entities, allowing for enhanced data insights and decision-making.
3. How does generative AI enhance graph technologies? Generative AI can be integrated with graph technologies to improve the accuracy of AI outputs, reduce hallucinations, and provide transparency in decision-making processes.
4. What are vector RAG and graph RAG? Vector RAG refers to retrieval-augmented generation using vector similarity, while graph RAG leverages relationships in graph data to provide richer contextual answers in responses generated by AI systems.
5. Why is fairness important in AI and knowledge graphs? Ensuring fairness helps prevent bias in AI outcomes and guarantees that all user groups are equitably represented, especially when handling sensitive data such as healthcare or financial information.