Over-quota Scheduling with Run:ai
Science & Technology
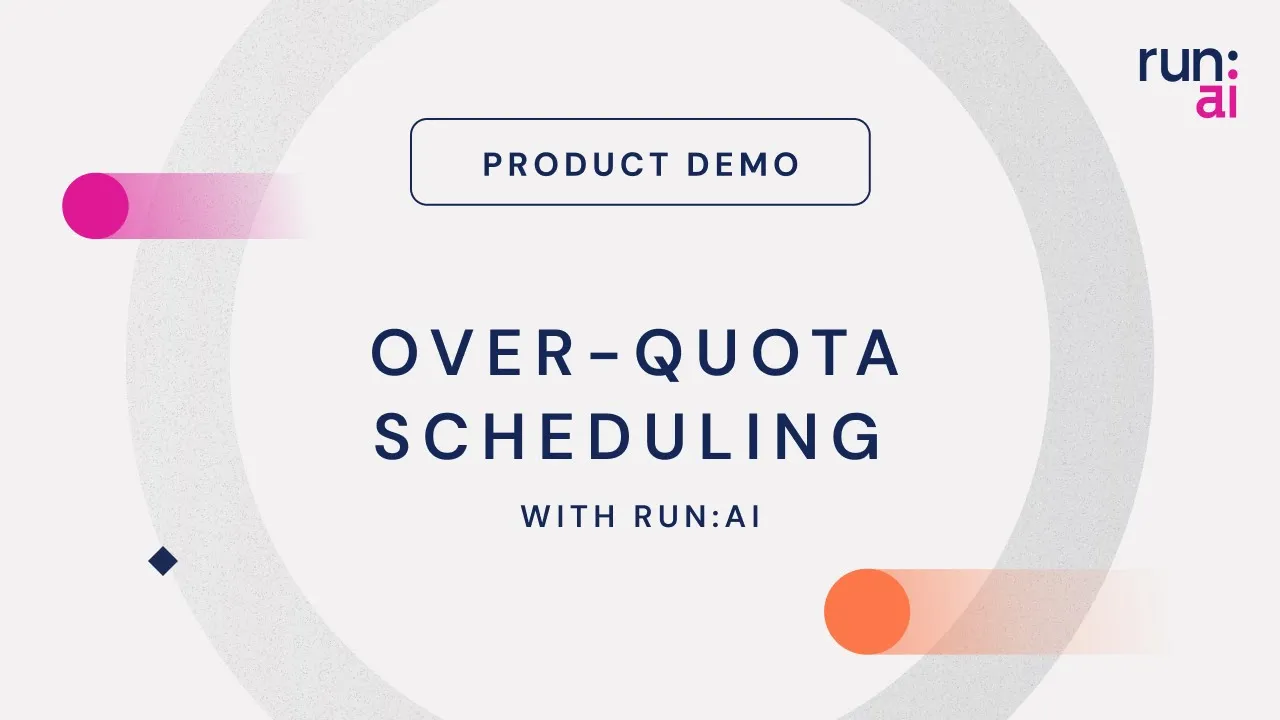
Over-quota Scheduling with Run:ai
In today's data-driven world, organizations are constantly seeking innovative ways to enhance research capabilities and maximize resource utilization. The Run:ai platform stands out as a robust solution for organizations looking to provide researchers with increased access to GPU resources, manage complex heterogeneous GPU infrastructures, and improve overall GPU utilization to achieve a better return on investment (ROI).
The Run:ai user interface offers a clear visualization of GPU resources. For instance, it showcases a Kubernetes cluster composed of four GPU nodes, totaling 32 GPUs. Users can observe the current status of jobs, with some actively running while others remain pending due to resource constraints. Each job is associated with a project, which can represent either an individual researcher or an entire research team.
One of the standout features of Run:ai is its ability to assign GPU quotas to projects. This ensures a fair distribution of available GPUs among teams. For example, if an organization has 32 GPUs, they might allocate 8 GPUs to each project. However, Run:ai goes beyond static GPU allocations by allowing projects to operate over quota. This means that when there are idle GPUs available, projects have the flexibility to utilize more than their allocated amount. Consequently, researchers can run additional experiments, enhance productivity, and ultimately achieve higher GPU utilization.
Before implementing the over-quota capability, a scenario might depict 11 jobs running with a GPU utilization rate of around 50%. By enabling this feature, organizations can nearly double the number of jobs that can run concurrently and increase GPU utilization to approximately 90%. This dramatic improvement empowers data science organizations to carry out significantly more experiments and achieve better productivity levels.
Navigating the platform is user-friendly, with access to detailed job views. Researchers can analyze individual job performance over time, including metrics such as GPU usage, GPU memory, CPU usage, and more. Additionally, the platform provides logs for each job, allowing for thorough monitoring and troubleshooting.
Overall, the Run:ai platform presents an efficient way to manage GPU resources effectively. By embracing over-quota scheduling, organizations can maximize their GPU infrastructure, leading to enhanced research capabilities and improved ROI.
Keywords
GPU resources, Run:ai, over-quota capability, Kubernetes cluster, job management, resource utilization, data science, productivity, infrastructure cost, ROI.
FAQ
Q1: What is Run:ai? A1: Run:ai is a platform designed to help organizations manage GPU resources effectively, providing researchers with better access and visibility to GPU infrastructures.
Q2: What is the main feature of Run:ai that enhances GPU scheduling? A2: The over-quota capability allows research projects to utilize more GPU resources than their assigned quotas when additional GPUs are available, optimizing resource utilization.
Q3: How does over-quota scheduling improve productivity? A3: By allowing projects to access idle GPUs, researchers can run more experiments concurrently, leading to increased productivity and enhanced research outputs.
Q4: Can I monitor job performance on the Run:ai platform? A4: Yes, the platform provides a detailed overview of each job's performance metrics over time, including GPU usage, memory, CPU utilization, and logs for troubleshooting.
Q5: How significant is the ROI improvement when using Run:ai? A5: Organizations can achieve a nearly double increase in GPU utilization and overall productivity, which translates to a significant improvement in the return on infrastructure investment.