Proactive Security Strategies for AI Integration
Education
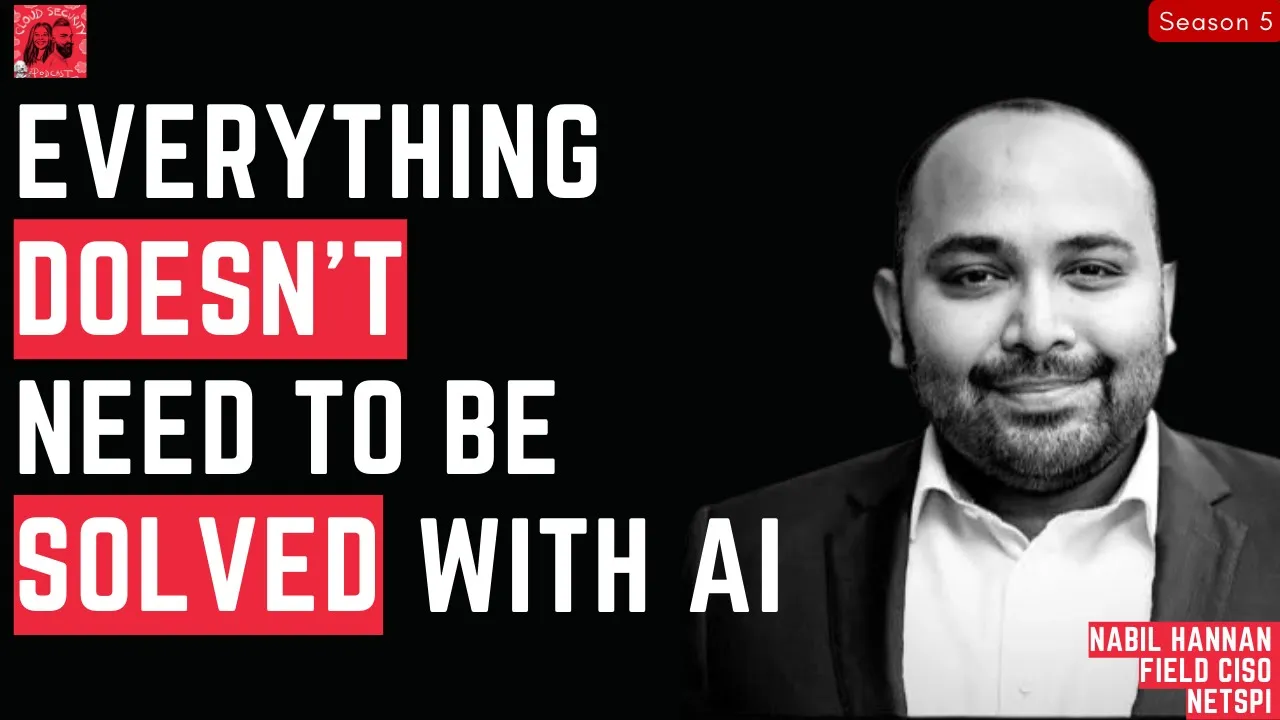
Introduction
Introduction Implementing AI in business applications has become a widespread trend as organizations aim to leverage the technology for a competitive edge. However, there's often a misunderstanding of AI's nature and its security implications. Hanan, the Field Chief Information Security Officer (CISO) for NetSPI, highlights the critical points about AI security, common attack scenarios, and foundational steps for implementing and testing AI technology to prevent exposure and breaches.
The Misconception of AI One critical issue in the discourse around AI is the term "artificial intelligence" itself. Many systems labeled as AI are not truly intelligent but rather advanced mathematical algorithms processing large datasets. This misunderstanding leads to improper security measures and an over-reliance on AI to solve all problems.
Challenges in AI Adoption Organizations frequently bypass basic security hygiene in their rush to adopt AI technologies, hoping for shortcuts to business advantages. Yet, this hurried approach often creates significant security gaps. Testing AI applications, especially generative models like OpenAI's ChatGPT, presents unique challenges due to their non-deterministic nature.
Common Attack Scenarios Hanan outlines several prevalent attack scenarios in the AI space:
Jailbreaking AI Models: Attackers manipulate AI to perform unintended actions, such as generating malware or providing confidential information. This exploitation can occur through cleverly crafted prompts that circumvent the model's intended restrictions.
Evasion Techniques: By creating mathematical overlays on data, attackers can trick models into misinterpreting inputs. For example, altering a stop sign's appearance to a model such that it reads it as a 55 mph sign, potentially leading to disastrous outcomes in self-driving cars.
Data Poisoning and Hallucinations: Inadequately managed training data can lead to AI models giving confidently incorrect or fabricated responses. This issue becomes critical when the outputs are used in legal, financial, or otherwise sensitive contexts.
Proactive Security Measures To secure AI integrations effectively, organizations should:
- Understand the Context: Clearly define the AI's intended use and ensure traditional security measures are in place before integrating AI solutions.
- Basic Security Hygiene: Implement foundational measures such as network segmentation, multifactor authentication, data encryption, and proper data classification.
- Thoughtful Adoption of AI: Not all problems require AI for their solution. Evaluate if AI truly adds value over traditional software solutions.
Pentesting AI Applications Penetration testing for AI applications differs depending on whether an organization is building or integrating AI models. While building AI involves extensive mathematical and computer science expertise, integrating AI focuses more on traditional security measures and understanding the operational context of the AI model. Prioritize testing the integration points and the overall system's basic security hygiene over directly testing proprietary models where feasible.
Conclusion The rapid adoption of AI necessitates a mindful approach to security. Executives and security leaders must balance the operational gains from AI with the critical need for security. By ensuring proper data governance, understanding AI's true capabilities and limitations, and focusing on proactive measures, organizations can better manage their security posture in the AI era.
Keywords
AI security, Jailbreaking AI, Data poisoning, Evasion techniques, Basic security hygiene, Pentesting AI, AI integration, Data governance, Generative AI, Artificial intelligence
FAQ
Q1: What is jailbreaking AI models? A: Jailbreaking AI refers to manipulating AI models to perform actions they were not designed to do, such as generating malicious code or divulging sensitive information.
Q2: How can evasion techniques affect AI models? A: Evasion techniques involve creating overlays on data inputs that trick AI models into misinterpreting their nature, potentially leading to severe real-world implications such as misread road signs in autonomous vehicles.
Q3: What foundational security measures should be in place before integrating AI? A: Organizations should ensure network segmentation, multifactor authentication, data encryption (both at rest and in transit), and adequate data classification and governance.
Q4: Is penetration testing of AI models different from traditional applications? A: Yes, pen testing AI models, particularly those built in-house, involves additional complexity requiring specialized expertise in mathematics and AI. In contrast, testing AI integration focuses more on traditional security measures and the operational context of the AI model.
Q5: How do data poisoning and hallucinations impact AI’s reliability? A: Data poisoning involves injecting malicious data during the model's training phase, leading to incorrect outputs. Hallucinations refer to AI models generating confident yet incorrect or fabricated responses, potentially causing significant issues in critical applications.