SCQ 2023 CSS Summer School | 'Big Data, AI, and Society:An Ethical Deep Dive ' by Dr. Tuba Bircan
People & Blogs
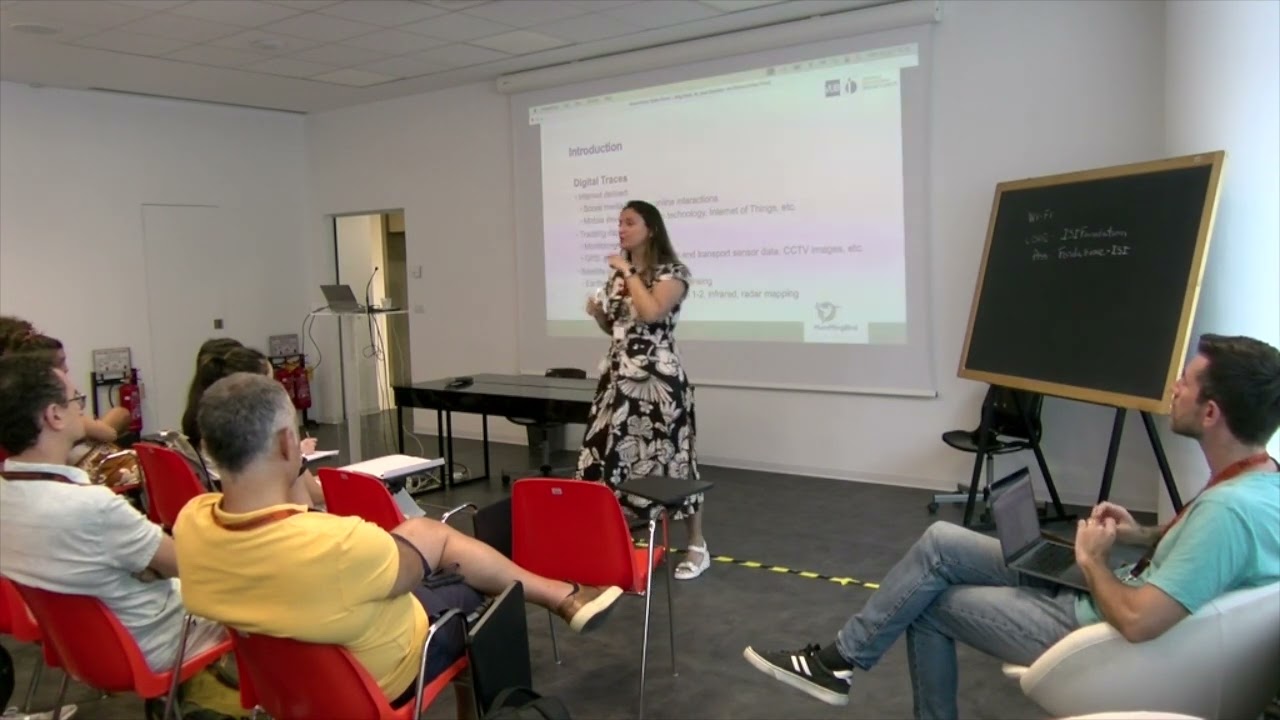
SCQ 2023 CSS Summer School | 'Big Data, AI, and Society: An Ethical Deep Dive' by Dr. Tuba Bircan
Introduction
Dr. Tuba Bircan delivered an extensive lecture on "Big Data, AI, and Society: An Ethical Deep Dive" at the SCQ 2023 CSS Summer School. Her discussions spanned across the challenges and ethics of working with big data and AI in social science research, employing various types of data, and the role of computational methods.
Understanding Big Data
Big data encompasses a variety of sources, including satellite data, mobile phone data, social media data, and ethnographic fieldwork. Bircan underscores the ambition of her project, which involves contributions from 16 partners across six countries. The project integrates different types of data to measure statistics of migration and mobility to inform policies.
Ethical Challenges in Big Data
Dr. Bircan outlined the primary challenges in working with big data:
- Privacy and Confidentiality: Issues arise around what is public data and what ethical data usage entails.
- Accuracy and Bias: The quality of data is often questionable, and biases can emerge in AI models that learn from real-world data.
- Transparency and Accountability: It is crucial to ensure that AI models are transparent and those developing and using these models are held accountable.
Case Studies
Migration Data
The Migration Radar 2 project by the European Space Agency (ESA) intends to analyze migration patterns. However, it raises questions about ethics, including the potential militarization of migration data and violations of migrants' privacy.
Facebook’s Experiments
Facebook's mood manipulation experiment in 2012 and the infamous Cambridge Analytica scandal are examples of how social media data has been misused to influence public opinion without informed consent.
Publicly Shared Data
The case of Strava’s publicly available fitness tracking data inadvertently revealed U.S. military bases' locations around the globe, demonstrating the dangers of seemingly innocuous data.
AI and Ethical Considerations
Highly discussed are the biases inherent in AI:
- Racial and Gender Bias: Dr. Bircan emphasized that AI systems often reflect society's existing biases, for instance, the AI-generated professor profiles that predominantly represented white males.
- Accountability: Determining responsibility in the case something goes wrong with AI—software developers, data analysts, or legal authorities—remains complex.
Mitigation Strategies
Dr. Bircan suggests various strategies to mitigate the issues raised:
- De-biasing Techniques: Actively working to remove bias from training datasets.
- Education and Literacy: Increasing literacy about computational methods among social scientists and vice versa.
- Ethical Oversight: The establishment of ethical boards and frameworks to guide the development and use of AI and big data.
Closing Thoughts
To ensure fairness in AI and big data analytics, social scientists must remain vigilant and actively participate in discussions surrounding these technologies. The aim is to create a balanced approach that leverages technology's benefits while minimizing its potential harm.
Conclusion
Dr. Tuba Bircan’s lecture serves as a critical reminder of the ethical considerations necessary in big data and AI applications, especially regarding social science research. The need for interdisciplinary approaches combining insights from computer science, social science, and law is essential for developing ethical guidelines and ensuring AI and big data work for the public good.
Keywords
- Big Data
- AI Ethics
- Privacy
- Bias in AI
- Social Science
- Migration Data
- Ethical Considerations
- Transparency
- Accountability
FAQ
Q: What are the primary ethical concerns related to big data and AI?
A: The primary concerns include privacy and confidentiality, accuracy and bias, and transparency and accountability in how data and AI models are developed and used.
Q: Can big data be unbiased?
A: While it's incredibly challenging to completely eradicate bias from big data, strategies like de-biasing techniques and ongoing ethical oversight can help minimize biases.
Q: How can we ensure accountability in AI systems?
A: Ensuring accountability involves determining who is responsible—be it software developers, data analysts, or legal authorities—in case something goes wrong, and maintaining transparent practices throughout the AI model's lifecycle.
Q: What is the importance of interdisciplinary approaches in tackling big data ethics?
A: Interdisciplinary approaches are crucial as they bring together insights from different fields like social science, computer science, and law, which are necessary to develop comprehensive ethical guidelines and practical solutions.
Q: Can AI reflect societal biases?
A: Yes, AI often mirrors existing societal biases because it learns from real-world data, which includes all the prejudices and inaccuracies inherent in society.
Q: What is the importance of literacy in big data and AI?
A: Literacy in these areas is vital. Social scientists must become familiar with computational methods, and computer scientists need to understand the social implications of their work to develop ethical and effective AI systems.