Surrogate Modeling of Site, Building, & Bridge System Performance in Regional Earthquake Simulations
Science & Technology
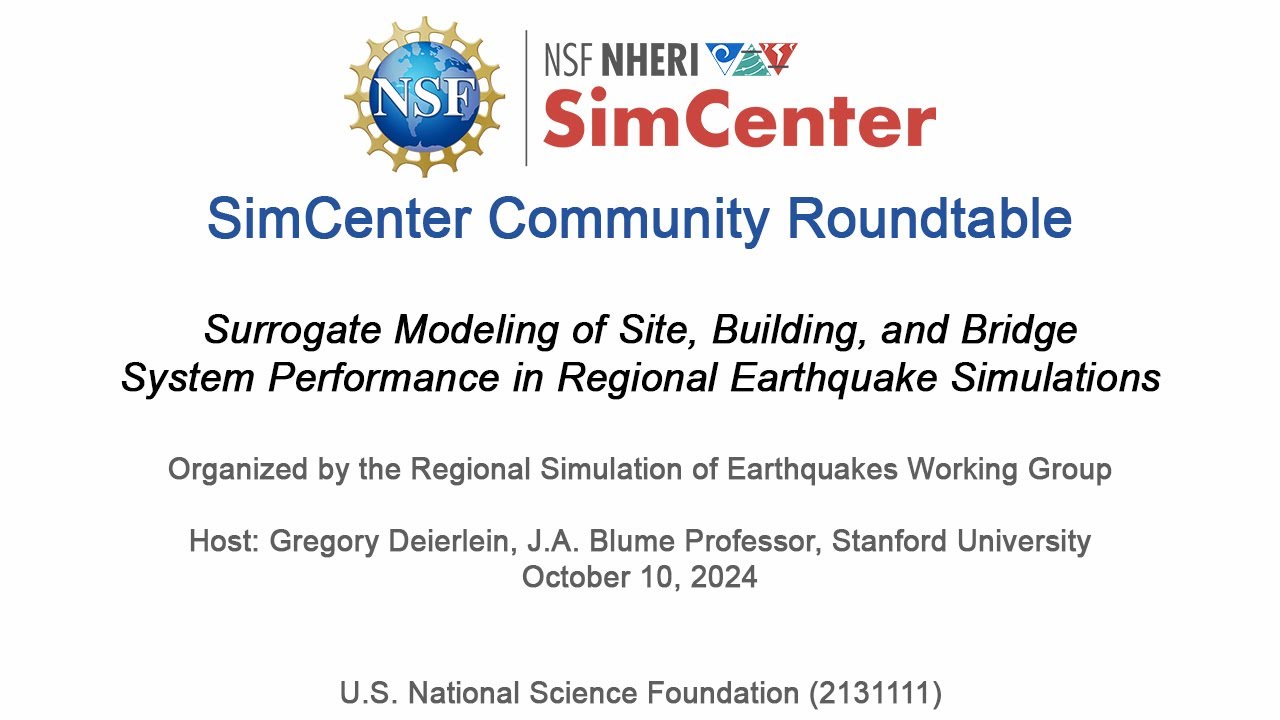
Introduction
Introduction
In recent developments at Stanford University's Sim Center, the focus has been on employing surrogate modeling techniques to enhance the fidelity of simulations in assessing the impact of geotechnical conditions on building and bridge systems in the context of regional earthquake risks. The overarching aim is to provide detailed and high-resolution models that move beyond conventional methods to better inform urban infrastructure resilience and safety.
Motivation for Surrogate Modeling
The mission of the Sim Center is to facilitate high-resolution simulations relevant to different regional geotechnical contexts, as illustrated with the case of San Francisco. Traditional models often rely on coarsely aggregated data, classifying buildings into groups and limiting the ability to differentiate individual structures. In contrast, the Sim Center aims to develop tools that allow for intricate characterization of building inventories, taking into account how built environments respond to seismic hazards.
Surrogate Modeling Framework
The Sim Center employs a range of software modules designed to tackle different components necessary for a thorough regional simulation. These components include:
- Characterizing building and bridge inventories
- Describing earthquake hazards
- Simulating structural responses
- Estimating damages
- Assessing recovery processes
Surrogate models, particularly those that are statistically based, are instrumental when it comes to extending high-fidelity models to larger inventories, facilitating efficient calculations without sacrificing accuracy.
Case Studies and Speaker Insights
During a recent roundtable discussion, several experts shared insights into their work involving surrogate models. Henry Burton from UCLA focused on surrogate modeling for building inventories related to risk and resilience assessment. Brett M from the University of Washington explored geospatial modeling related to soil liquefaction, while Tim Sai from Mill University presented on regional facility analysis of bridge infrastructure.
The conversations underscored the necessity of effective surrogate modeling that can either replicate complex behaviors or enhance efficiency in computations. Each speaker highlighted practical applications, specifically regarding the need for models that are both usable and useful within engineering workflows.
Recommendations for Future Work
- Creating libraries of readily accessible surrogate models.
- Leveraging computational tools that ease the sharing of models across regions and disciplines.
- Encouraging collaborations that bolster expertise in both modeling and data science.
Conclusion
The need for advanced predictive modeling in earthquake simulations is increasingly evident. By focusing on building more robust surrogate models tailored to specific regional contexts, there lies an opportunity for the engineering community to significantly enhance its understanding of seismic risks and facilitate better decision-making in urban infrastructure planning.
Keyword
- Surrogate Modeling
- Earthquake Simulations
- Building Inventory
- Structural Responses
- Soil Liquefaction
- Computational Tools
- Risk Assessment
FAQ
1. What is surrogate modeling in the context of earthquake simulations? Surrogate modeling involves creating simplified versions of complex simulations that retain essential characteristics of the original model. This allows for quicker calculations and analysis, particularly when dealing with large inventories of structures.
2. Why is high-resolution modeling important? High-resolution modeling allows engineers to make more accurate predictions about how specific buildings or bridges will perform during seismic events. This helps in better risk mitigation and recovery planning.
3. How does the Sim Center facilitate the sharing of surrogate models? The Sim Center creates libraries of surrogate models and provides computational tools that allow different stakeholders to access and employ these models in their own analyses.
4. What role does machine learning play in surrogate modeling? Machine learning can be utilized as a tool to develop surrogate models by identifying patterns within the data that can help predict outcomes more efficiently than traditional methods.
5. Can surrogate models be applied across different regions? While surrogate models can often be adapted for use in different regions, it is important to validate and possibly retrain models using local data to ensure their accuracy and relevance to that specific context.