Text-to-video models explained
People & Blogs
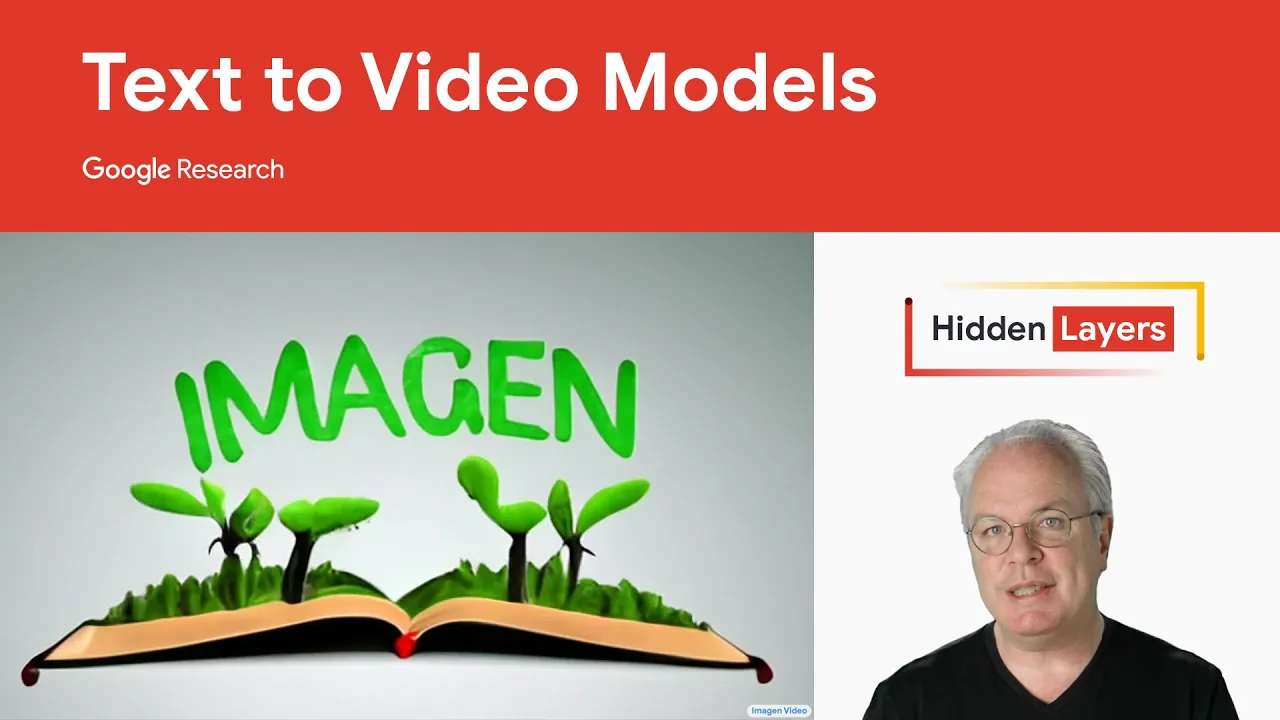
Introduction
In this article, we'll delve into the fascinating world of text-to-video models, specifically how these advanced machine learning algorithms generate videos from text prompts. A prominent example of this technology is the Imagine Video model, which produces impressive videos that can match simple text descriptions. For instance, one can create a short video of "an astronaut riding a horse" purely from this written prompt.
Understanding Diffusion Models
To understand how text-to-video models work, we must first examine diffusion models used for image generation. These models are trained to add noise to an image and subsequently learn how to denoise it back to its original state. The process, however, incorporates a critical element: a text vector that corresponds to the image caption during training. This allows the model to produce images that align with the given text when denoising.
Transitioning to Video Generation
In video generation, the approach remains similar; rather than introducing noise to a single image, noise is applied to several video frames. The denoising model learns to reverse this noise for each frame while considering the related text description. Due to the complexity involved in modeling video data, training often begins with smaller, lower-resolution videos. For example, Imagine Video works with 16 frames captured at three frames per second, producing slightly over five seconds of video at a resolution of 40 by 24 pixels.
The Workflow of Imagine Video
The process of generating high-quality videos, as seen in the examples discussed, involves orchestrating multiple models—specifically seven in the case of Imagine Video. Here’s how it works step by step:
Text Prompt to Initial Video: The initial model transforms the text prompt into a 16-frame video at a rate of three frames per second.
Time Super Resolution (TSR): This output is then fed into the time super-resolution model to interpolate and double the frames from 16 to 32, resulting in a video of six frames per second.
Spatial Super Resolution (SSR): The first spatial super-resolution model ups the video’s resolution from 32 frames at 80 by 48 pixels while maintaining the frame rate.
Enhanced Resolution: A second SSR model further scales the resolution up to 320 by 192 pixels while keeping the frame count constant at 32 frames per second.
Additional TSR Models: Another TSR model doubles the frame count from 32 to 64 frames per second (12 FPS), and yet another doubles it again to achieve 128 frames.
Final Upscaling: The final spatial super-resolution model increases the resolution to 1280 by 768 pixels, creating a 24 frames per second video lasting just over five seconds.
This orchestration of models, adeptly generating and enhancing both spatial and temporal characteristics of the video, allows the underlying system to produce visually stunning results.
Conclusion
The field of text-to-video generation is rapidly progressing. Models like Imagine Video represent a significant step forward, showcasing how machine learning can bridge the gap between textual concepts and rich audiovisual content. As research continues to evolve in this space, viewers can expect even more sophisticated systems in the future.
Keyword
- Text-to-video models
- Imagine Video
- Diffusion models
- Denoising
- Video generation
- Time Super Resolution (TSR)
- Spatial Super Resolution (SSR)
FAQ
Q: What are text-to-video models?
A: Text-to-video models are advanced machine learning algorithms that generate videos based solely on given text prompts.
Q: How do diffusion models work in video generation?
A: Diffusion models add noise to video frames and learn to denoise them back to their original state, using a text caption to guide the process.
Q: Why are video models trained on low-resolution videos initially?
A: Due to the complexity of video data, models are initially trained on smaller, lower-resolution videos to manage the training process.
Q: What is the workflow of Imagine Video?
A: Imagine Video orchestrates multiple models to generate and upscale video from initial low-resolution frames to high-quality videos while enhancing both frame rate and resolution.
Q: What is the significance of Time Super Resolution (TSR) and Spatial Super Resolution (SSR)?
A: TSR increases the number of frames in a video, enhancing temporal smoothness, while SSR improves the video resolution, making it clearer and more visually appealing.