The future of AI in medicine | Conor Judge | TEDxGalway
Nonprofits & Activism
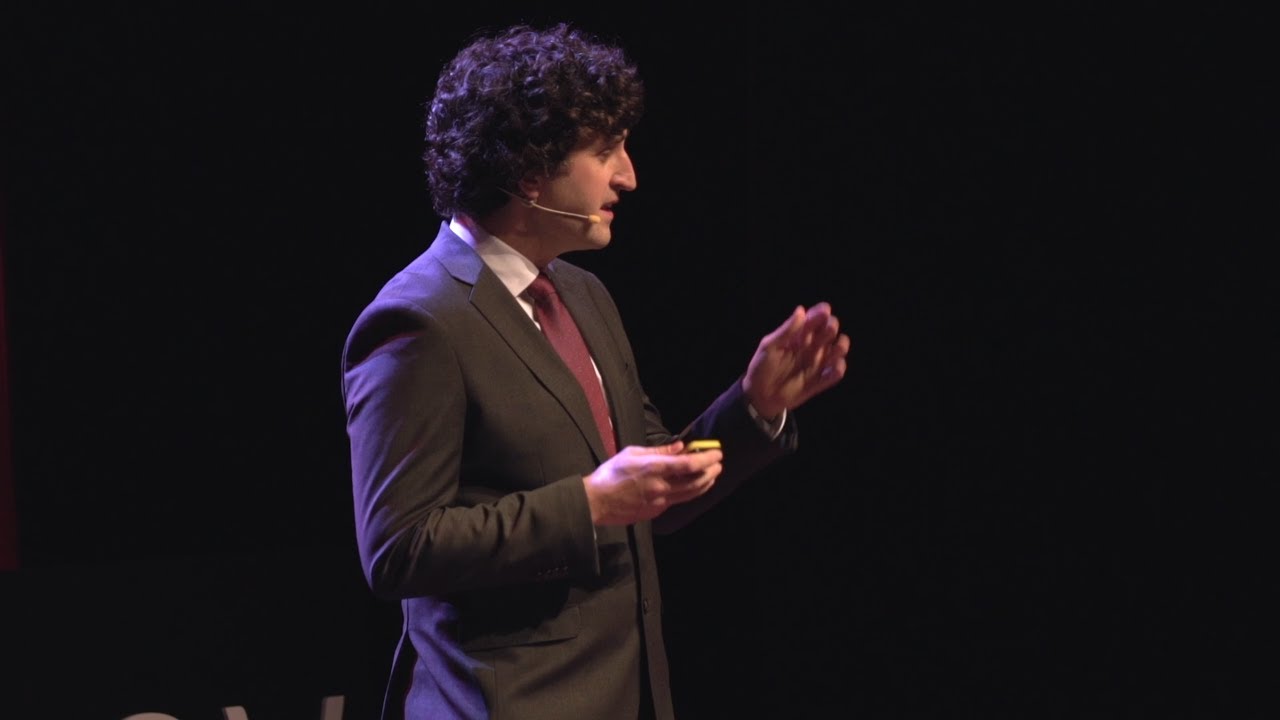
Introduction
[Applause]
[Music]
The last time I stood on the stage of the Town Hall Theater was 26 years ago. I was a handsome 12-year-old boy, participating in a drama competition for local schools, acting in a play written by my best friend. In that play, I portrayed a detective trying to solve the mystery of who robbed a fictional hotel called Hotel El Chipo. At that time, I was also a boy with a stammer, struggling to remember and articulate my lines. I spent about 70% of my time collecting data to solve the mystery.
Fast forward 26 years, and not much has changed. Now, I work as a medical consultant in the hospital for half of my time, and as a senior lecturer in applied clinical data analytics at the university for the other half. The context has shifted from solving a mystery about a hotel robbery to diagnosing the cause of illness in the patients before me. I still spend about 70% of my time gathering patient information and only about 30% making decisions and communicating with them.
The data I collect comes from various sources—patient medical histories, blood pressure readings, and blood test results. This imbalance in healthcare delivery, known as the 70-30 ratio, is recognized globally across many medical specialties. The introduction of electronic health records has worsened this imbalance, as these systems were designed more for billing than for efficiency in healthcare. Consequently, doctors face an increased administrative workload, which reduces the vital FaceTime with patients—an interaction we are trained for and that patients desire.
The idea worth spreading that I want to share tonight presents a potential solution: the responsible use of medical AI. Today, I’ll introduce a new type of artificial intelligence: multimodal AI. Unlike single model AI, which analyzes one type of data at a time—text, images, or numbers—multimodal AI processes various forms of data simultaneously.
In November last year, media coverage surrounding AI exploded with the release of ChatGPT by OpenAI. ChatGPT is a large language model (LLM) but is not the only type of AI. Other types include machine learning, computer vision, and natural language processing, which typically deal with a single type of data, referred to as single model AI.
Here are three cutting-edge applications of single model AI in healthcare:
Chest X-Rays: A software called ChestLink by Oxy Pit is the first AI system to receive regulatory approval for fully autonomous reporting on chest x-rays, identifying 75 abnormalities. If no abnormalities are found, it reports the x-ray as normal without human input.
Eye Disease Diagnosis: Researchers from University College London developed an AI model trained on 1.6 million retinal images that can diagnose eye diseases such as macular degeneration. Impressively, this model can predict conditions like Parkinson's disease years before symptoms appear.
Language Models: Google released a medical LLM called Med-PaLM that passed a US medical licensing exam. The newer version, Med-PaLM 2, achieved a passing score of 86%, demonstrating expertise.
Just weeks ago, OpenAI released a multimodal version of ChatGPT. An example I recently tested involved inputting an ECG (electrocardiogram) and a patient scenario, featuring a 60-year-old male with palpitations. While the initial response was vague, a follow-up interaction yielded practical advice.
Advances in multimodal AI include models like Med-PaLM M from Google, which can analyze various medical images and text data for multiple medical tasks. It has outperformed human radiologist reports 40% of the time. However, to implement multimodal AI safely, we must address three key areas: trust, explainability, and randomized clinical trials.
Trust: A survey in the U.S. showed that over half of respondents would feel anxious if their healthcare relied on AI. Approximately 75% feared a hasty integration of AI without understanding potential risks.
Explainability: We need to understand why an AI system might recommend a specific treatment, rather than blindly following its conclusions.
Randomized Clinical Trials: AI models should undergo trials similar to those used for testing new medicinal treatments to ensure safety and effectiveness.
So, where does the art of medicine fit into this equation? As medical students, we are taught to consider the patient’s context before interpreting any results. A patient's visual assessment in emergency departments, sometimes more effective than sophisticated models, supports this notion.
In the future, I envision a healthcare landscape where various patient inputs (like images and videos) are integrated into multimodal AI systems.
Reflecting on my past performance as a nervous 12-year-old, the parallels are striking: just as I sought data to solve my fictional mystery, we now collect data in healthcare for something more profound: making healthcare more efficient, personalized, and accessible. Imagine a scenario where remote areas in low and middle-income countries gain access to healthcare insights through these models.
As we look toward this future, it’s imperative to emphasize compassion and understanding, bridging the relationship between AI and human healthcare providers. This will ultimately allow doctors more time to connect with their patients and enhance their chances for health and happiness.
Thank you.
[Music]
Keywords
AI in medicine, multimodal AI, single model AI, ChestLink, eye disease diagnosis, Med-PaLM, randomized clinical trials, explainability, patient care, healthcare efficiency.
FAQ
Q: What is multimodal AI? A: Multimodal AI refers to artificial intelligence systems that can process multiple types of data simultaneously, such as text, images, and numbers.
Q: What are some examples of single model AI in healthcare? A: Examples include ChestLink for analyzing chest x-rays, AI systems for diagnosing eye diseases, and Med-PaLM for medical question answering.
Q: How can AI improve patient care? A: AI can help reduce the administrative burden on physicians, allowing them to spend more time with patients, thereby improving care quality.
Q: Why is trust in AI essential in healthcare? A: Trust is essential because patients need confidence that AI-driven recommendations and treatment strategies are safe and effective.
Q: What does explainability in AI refer to? A: Explainability refers to understanding how AI models arrive at specific recommendations or results, which is crucial in a healthcare context.
Q: How can AI models be validated? A: AI models should undergo randomized clinical trials, similar to how new medicines are tested, to ensure they are safe and effective for patient use.