The Future Of AI Video Generation
Science & Technology
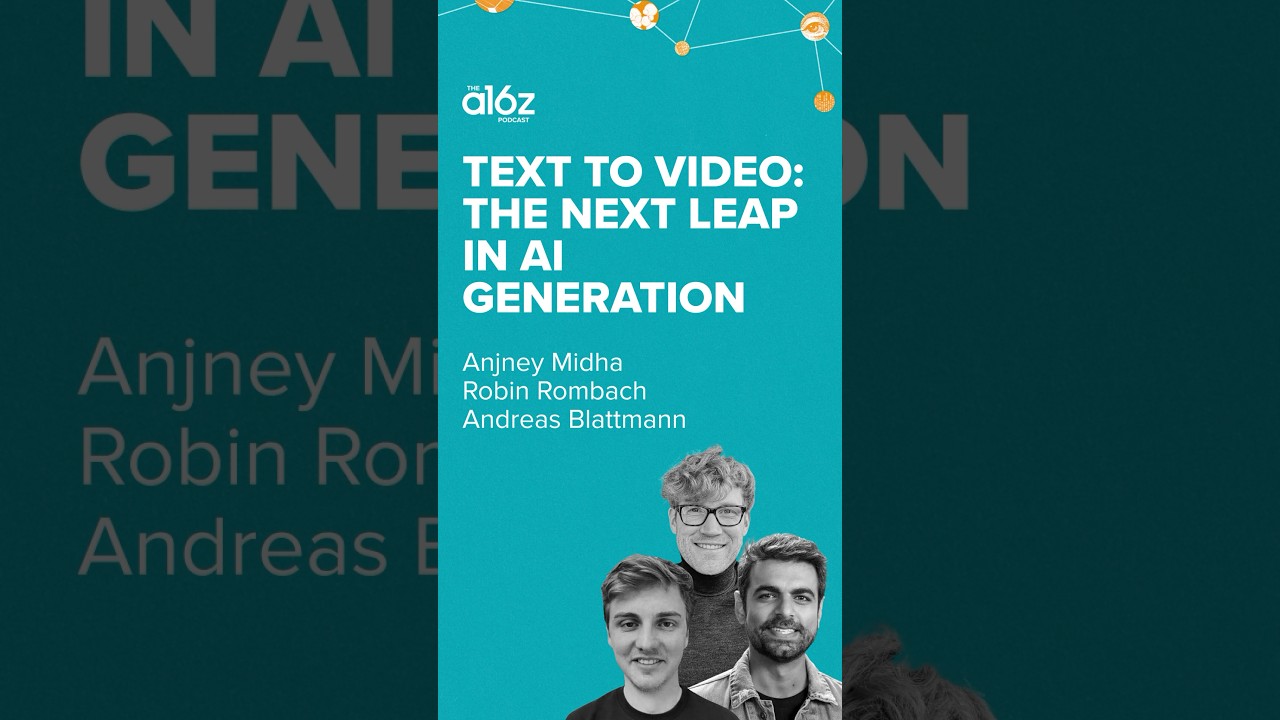
The Future Of AI Video Generation
In recent developments, we've discovered that having a foundational video model provides significantly more benefits than merely generating aesthetically pleasing clips. This article explores how a foundational video model can also learn a robust representation of the world. One key aspect explained in the study is the ease with which such a model can be adapted into a multi-view model.
Our approach involves taking a pre-trained media model that has been exposed to a myriad of objects from different views and various camera movements and fine-tuning it on specialized multi-view orbits around 3D objects. This effectively transforms the video model into a multi-view synthesis model.
A major advantage of this method over previous dominant approaches is highlighted. Prior methods generally involved converting image models, like Stable Diffusion, into multi-view models. However, our study shows that incorporating implicit 3D knowledge captured from numerous videos enables the model to learn and perform more efficiently than starting from a purely image-based model.
By leveraging a foundational video model, we gain an enriched representation of the world that allows for faster and more sophisticated multi-view learning capabilities.
Keywords
- Foundational Video Model
- Representation Learning
- Multi-View Synthesis
- Pre-trained Media Model
- 3D Objects
- Stable Diffusion
- Implicit 3D Knowledge
FAQ
Q: What is a foundational video model?
A: A foundational video model is an AI model trained on a vast amount of video data, capturing various objects, views, and camera movements, thereby developing a rich representation of the world.
Q: How does a foundational video model differ from prior image models like Stable Diffusion?
A: Unlike image models, a foundational video model incorporates implicit 3D knowledge from numerous videos, allowing it to learn and adapt faster for multi-view synthesis tasks.
Q: What are the benefits of using a pre-trained media model for multi-view synthesis?
A: Using a pre-trained media model that has seen different objects and views simplifies the adaptation process for multi-view synthesis and enhances the model’s learning efficiency.
Q: Why is a rich representation of the world important for AI models?
A: A rich representation allows AI models to understand and synthesize visual elements more accurately and efficiently, facilitating advanced applications like multi-view synthesis and 3D object manipulation.