The Future of Knowledge Graphs in a World of Large Language Models
Science & Technology
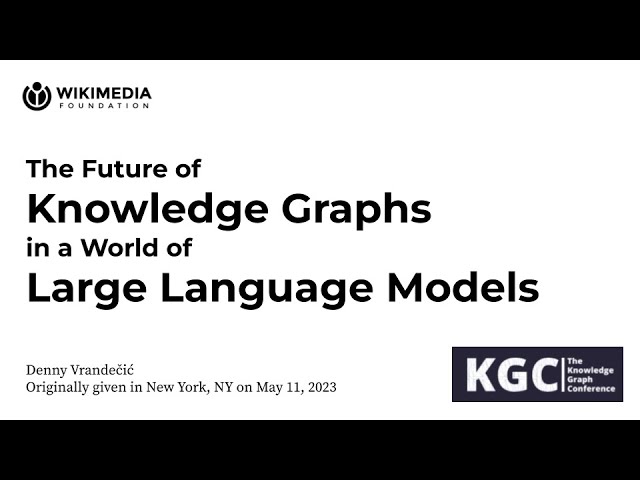
Introduction
In May 2023, a keynote speaker was invited to the Knowledge Graph conference in New York City. The speaker was given the topic of discussing knowledge graphs and large language models. Although filled with doubts, the speaker believed that knowledge graphs still have a future in a world dominated by large language models. They acknowledged that something big is happening with the rapid adoption of technology. However, they also highlighted the challenges and limitations of large language models, such as cost, consistency, knowledge ambiguity, and lack of robustness.
The speaker emphasized the importance of knowledge graphs as a reliable source of ground truth for large language models. They compared the efficiency and cost-effectiveness of knowledge graph queries against the computational complexity and expense of large language model computations. They also discussed the potential benefits of augmenting language models with knowledge graphs, allowing for more efficient operations and better accuracy in specific tasks.
The speaker highlighted the need for accurate and consistent knowledge in the age of large language models. They discussed the challenges of determining the truth in an information ecosystem where different sources may yield conflicting answers. They also explored the limitations of large language models in math computations and language understanding.
The speaker proposed the idea of externalizing knowledge from large language models and storing it in knowledge graphs. By leveraging the strengths of both knowledge graphs and large language models, they argued for a more efficient and accurate system. They suggested the use of augmented language models, such as Metasalama and WikiFunctions, to enhance the capabilities and reliability of large language models.
In summary, the speaker expressed their belief that knowledge graphs still have a future in a world dominated by large language models. They emphasized the importance of storing valuable knowledge in a symbolic system, like a knowledge graph, and leveraging large language models for efficient knowledge extraction. They also discussed the need for improvements in consistency, accuracy, and robustness in the integration of knowledge graphs and large language models.
Keywords:
Knowledge Graphs, Large Language Models, Ground Truth, Augmented Language Models, Computational Complexity, Cost-effectiveness, Consistency, Knowledge Ambiguity, Externalizing Knowledge
FAQ:
- What is the future of knowledge graphs in a world of large language models?
- How do knowledge graphs compare to large language models in terms of efficiency and cost?
- What are the limitations of large language models in math computations and language understanding?
- How can knowledge graphs be augmented with large language models to enhance their capabilities?
- What are the challenges of determining the truth in an information ecosystem influenced by different sources?