The Road to Intelligent Data Apps | The Role That Causality Plays in Future State Agentic AI Systems
Science & Technology
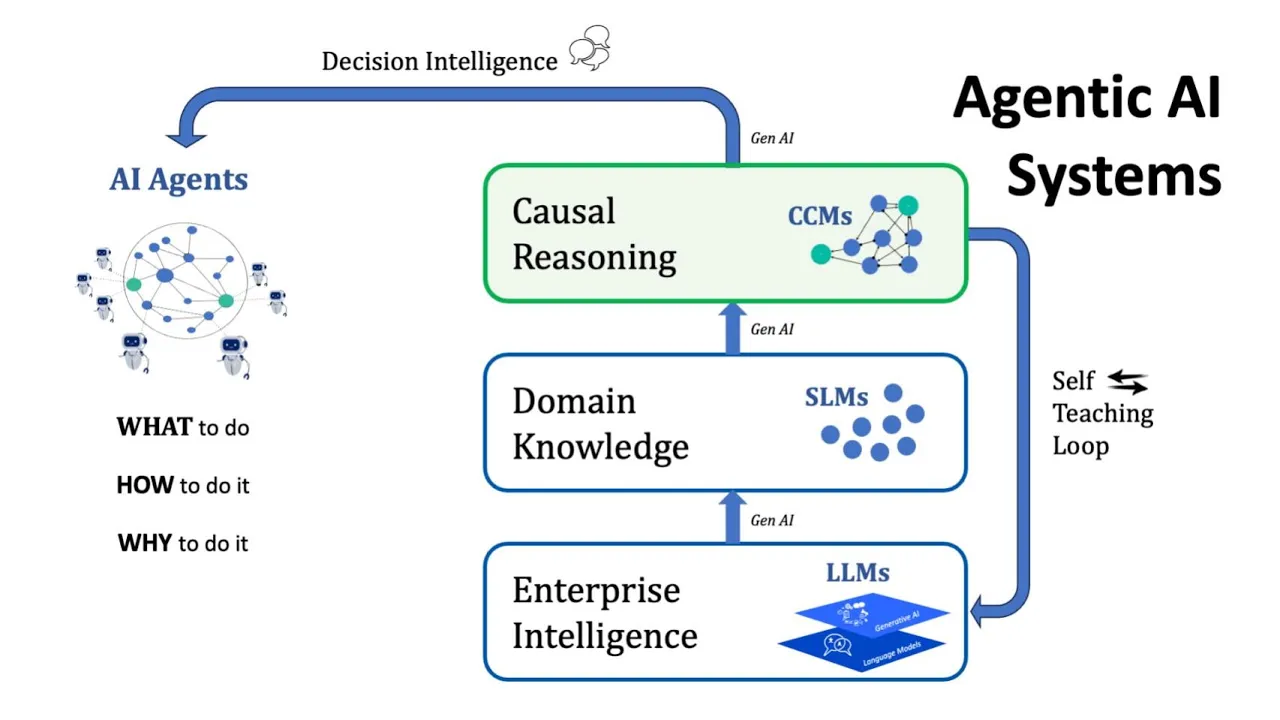
Introduction
In a special edition of the “Road to Intelligent Data Apps,” the rapid evolution of the AI marketplace is once again in focus. With developments in artificial intelligence proliferating at an unprecedented rate, organizations must stay ahead of the curve. Our conversation today features Scott Hebner, our newest principal analyst, who is dedicated to keeping us informed about the latest advancements shaping the future of AI. Scott recently published a research note discussing the critical role that causality plays in future state agentic AI systems.
As Hebner outlined, the landscape of AI is progressing at a pace previously unseen. It’s essential for businesses to not only focus on today’s return on investment (ROI) but also prepare for what’s coming next. The multitude of companies in the AI marketplace necessitates learning from prior experiences to prevent redundant efforts, especially as advancements continue to build upon previous technologies.
Understanding Agentic AI vs. Traditional AI Assistants
A significant aspect of the conversation centered on differentiating agentic AI from traditional AI assistants and chatbots. While statistics indicate that about 70% of global businesses utilize AI chatbots or assistants, these systems typically focus on specific, predefined tasks based on explicit prompts. In contrast, agentic AI systems have a higher level of sophistication by being goal-oriented rather than just task-oriented.
Agentic AI aims to facilitate the achievement of goals that are often dynamic in nature and not strictly defined. This sophistication entails helping users identify how to achieve their goals through collaboration with other agents that each have their own objectives and knowledge bases.
The Challenges and New Solutions
One of the most pressing challenges in the transition from AI assistants to agentic AI systems involves bridging the significant gap in capabilities. Hebner mentioned a Gartner chart illustrating this divide, highlighting that current task-oriented systems lack the adaptability needed for more complex environments.
Causal reasoning is essential to address these challenges. It allows AI systems to understand the relationships between variables and the consequences of decisions made. By incorporating causal reasoning, AI systems can transform decision-making processes to not only identify actions but also explain the reasoning behind those actions.
As Hebner explained, while current AI models yield results based on patterns, causality adds an essential layer of reasoning, making it a critical ingredient for realizing agentic systems. This is akin to how humans think, relying on a complex web of cause-and-effect relationships.
New Ingredients for AI Architecture
To leverage the power of these advanced systems, businesses must utilize various tools and methods to inject causality into their AI architectures. An ecosystem of vendors is emerging that provides these solutions, helping developers integrate causal reasoning into their AI models incrementally.
Hebner compared the integration of these tools to the functioning of the human brain, where larger language models act as the memory and instincts, while causal components facilitate reasoning and decision-making.
Future Research Directions
Looking ahead, Hebner plans to publish additional research focusing on real-world case studies of agentic AI applications, delve into the technology underpinning causal reasoning, and outline how these components can fit into a cohesive architecture for agentic systems.
In conclusion, the fascinating evolution toward agentic AI systems hinges on effectively harnessing causal reasoning to equip businesses with advanced decision intelligence capabilities. This ongoing transformation promises to enrich how organizations interact with AI, making the technologies more intelligent, adaptable, and effective.
Keywords
- Agentic AI
- Causality
- AI Assistants
- Dynamic Goals
- Task-based Systems
- Decision Intelligence
- Adaptive Systems
- Knowledge Graphs
- Correlation vs. Causation
- Ecosystem
FAQ
1. What is the difference between agentic AI and traditional AI assistants?
Agentic AI is goal-driven and sophisticated, focusing on achieving dynamic objectives through collaboration between multiple agents, while traditional AI assistants are primarily task-oriented and limited to explicit commands.
2. Why is causality important in AI systems?
Causality enables AI systems to understand cause-and-effect relationships, allowing for better decision-making and the ability to explain actions and outcomes, which is crucial for realizing agentic AI.
3. What challenges do organizations face when transitioning to agentic AI?
The primary challenge lies in bridging the capabilities gap between simple task-oriented systems and more complex adaptive systems that require causal reasoning to function effectively in dynamic environments.
4. How can businesses start integrating causality into their AI architectures?
Businesses can utilize tools and methods provided by various vendors to inject causality into existing AI frameworks, allowing for incremental advancements without the need to completely start over.
5. What can we expect in future research on agentic AI?
Future research will likely explore real case studies, delve into the technologies that support causal reasoning, and outline cohesive architectures for integrating these systems effectively into organizations.