The Surprising Truth About AI in Agile Development Nobody Tells You | The AI Pulse
Science & Technology
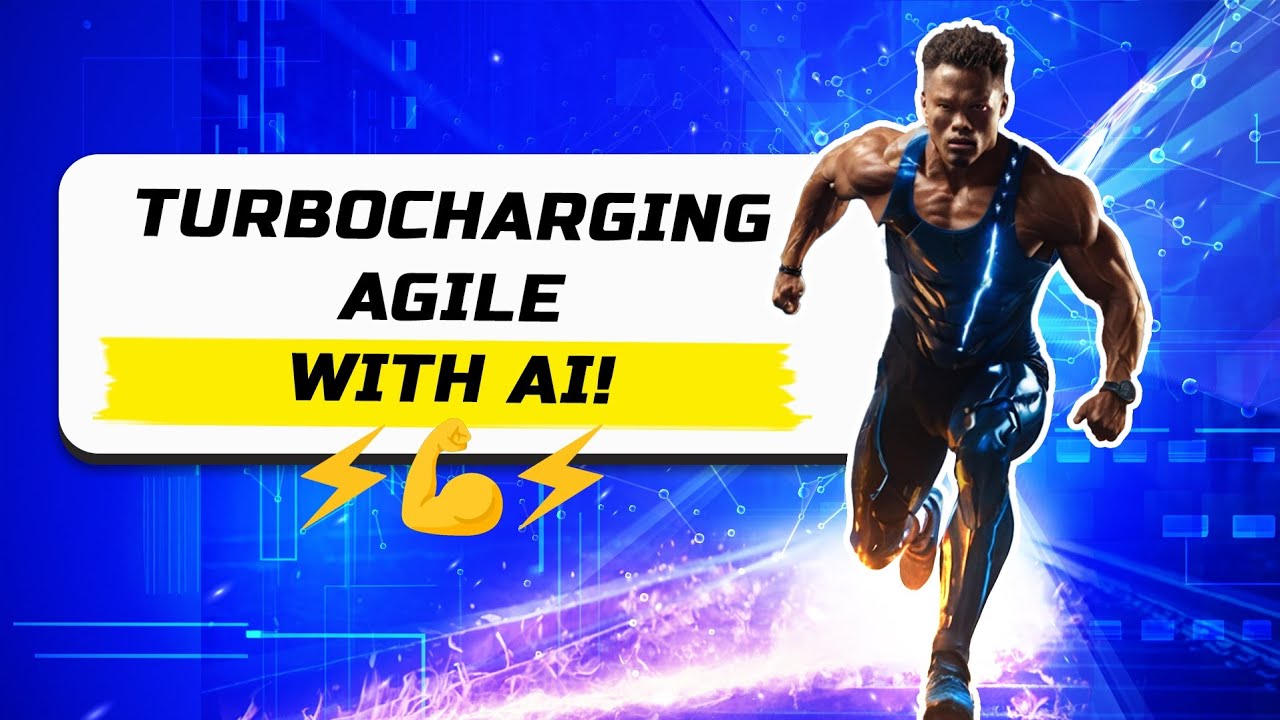
Introduction
Agile teams are on the verge of experiencing a productivity boost of epic proportions, thanks to the transformative power of Artificial Intelligence (AI). Imagine the power of agile's inherent flexibility combined with AI's precision, speed, and predictability. However, one must ponder: can the unpredictable nature of agile truly coexist with AI's data-driven systems? In this article, we will explore the potential synergy between agile methodologies and AI, and how this combination can redefine team functionality.
Understanding Agile Methodologies
To appreciate this synergy, let’s first revisit the core principles of agile. Agile methodologies emphasize flexibility, adaptability, and iterative progress. They are designed for rapid, incremental development, prioritizing collaboration, customer feedback, and swift responses to changes. However, even the best agile teams encounter limitations—communication breakdowns, overwhelming backlogs, and shifting deadlines that can feel chaotic.
Enter AI: The Game Changer
AI emerges as the perfect complement to agile's strengths. While agile teams face bottlenecks such as swelling backlogs and misaligned Sprint goals, AI-powered tools serve as a remedy to these inefficiencies. For instance, imagine an AI system that streamlines backlog grooming and Sprint planning. AI can swiftly prioritize tasks by analyzing previous Sprint data, customer needs, and market trends, effectively slashing hours of debate into seconds.
Tools like Jira’s predictive backlog prioritizer exemplify this functionality. They drastically reduce manual effort and enable faster decision-making. In another vein, platforms like Zoho Sprints utilize AI for accurate Sprint forecasting, evaluating team capacity and velocity to set achievable goals—an unexpected predictability in the agile world.
The Statistics Speak
The integration of AI into agile methodologies has yielded remarkable results, with companies experiencing project delays reduced by 20% and productivity improvements that soar by 30%. AI shines particularly in automating routine tasks; for example, continuous testing can become a breeze with AI-driven tools like Test.io, running numerous test cases in minutes and catching issues before escalating into bigger problems. Facebook's automation tools have shown to cut testing times by a staggering 70%.
Real-Time Insights for Retrospectives
As Sprint cycles conclude and teams hold retrospectives, the typical discussions often brim with subjective opinions. However, imagine utilizing AI to analyze team performance and task efficiency, delivering a fact-based summary of each retrospective meeting. Tools like Range and Standuply can synthesize team activities and communication, presenting actionable insights powered by historical data.
Consider Spotify, a global giant that struggled to scale agile across remote teams. By integrating AI, they streamlined Sprint planning, cut time to market by 30%, and transformed internal communication. The result? Predictable operations with minimized miscommunication.
Empowering Leadership with Data-Driven Decision-Making
Leadership also reaps significant benefits from this AI integration. Data-driven decision-making has always been crucial in agile environments, but with AI, it reaches unprecedented levels, allowing leaders to visualize team performance, mood, engagement, and collaboration quality. With clear visibility into team dynamics, managers can allocate resources more efficiently, tackling potential issues like burnout before they arise. According to McKinsey, agile teams leveraging AI in their leadership frameworks saw a 20% increase in team satisfaction and a 35% boost in operational efficiency.
The Importance of Governance
While AI brings remarkable efficiency, it is imperative to address privacy concerns. Data security is critical, especially as AI analyzes sensitive project information. Additionally, AI systems can reproduce biases inherent in historical data. As such, it is vital to establish strong governance around AI, ensuring diversity in data training and transparency in AI outcomes.
The Future of AI in Agile Development
Looking ahead, the integration of AI into agile methodologies will enhance scalability without sacrificing the human touch. Edge computing and AI at the edge will facilitate real-time decision-making, diminishing delays in agile workflows. Advancements in natural language processing (NLP) will enable AI to manage communication in increasingly sophisticated ways—summarizing entire conversation threads and dismantling information silos.
We stand on the brink of a new era. AI doesn't merely support agile; it turbocharges it. It eliminates bottlenecks, forecasts outcomes, automates repetitive tasks, and provides razor-sharp insights. The flexibility of agile combined with the speed and precision of AI creates an ecosystem where project timelines shrink, team performance elevates, and product quality improves significantly.
As we advance, agile methodologies will no longer rely solely on human intuition; they will be driven by powerful algorithms that learn and adapt with each Sprint. The future is a blend of data-driven retrospectives, automated Sprint forecasts, and AI-powered collaboration tools.
Conclusion
Now it's your turn! How have you integrated AI into your agile workflows? Share your experiences in the comments below. Don’t forget to subscribe if you’re eager to learn about the next wave of AI tools transforming agile practices—you won't want to miss it! Stay ahead of the curve, and stay inspired.
Keyword
AI, Agile Development, Productivity, Backlog Prioritization, Sprint Planning, Data-Driven Decision-Making, Continuous Testing, Leadership, Governance, Natural Language Processing, Team Performance.
FAQ
1. How does AI improve agile workflows?
AI optimizes backlog prioritization, facilitates faster Sprint planning, automates testing, and provides data-driven insights to enhance overall team performance.
2. What are some common tools that integrate AI with agile methodologies?
Some examples include Jira’s predictive backlog prioritizer, Zoho Sprints, Test.io for automated testing, and tools like Range and Standuply for retrospectives.
3. Can AI manage team communication?
Yes, with advancements in natural language processing, AI can summarize communication threads and streamline information sharing within teams.
4. What are the challenges of integrating AI into agile?
Privacy concerns regarding data security, the potential for bias in AI outcomes, and the need for strong AI governance are crucial challenges to address.
5. What benefits do leaders gain from AI in agile environments?
AI provides visibility into team dynamics, enabling data-driven decision-making that can improve resource allocation, address burnout, and boost team satisfaction and operational efficiency.