? Ultimate AI Text-To-Video Model Comparison: Kling Pro vs MiniMax vs Luma Dream Machine
Science & Technology
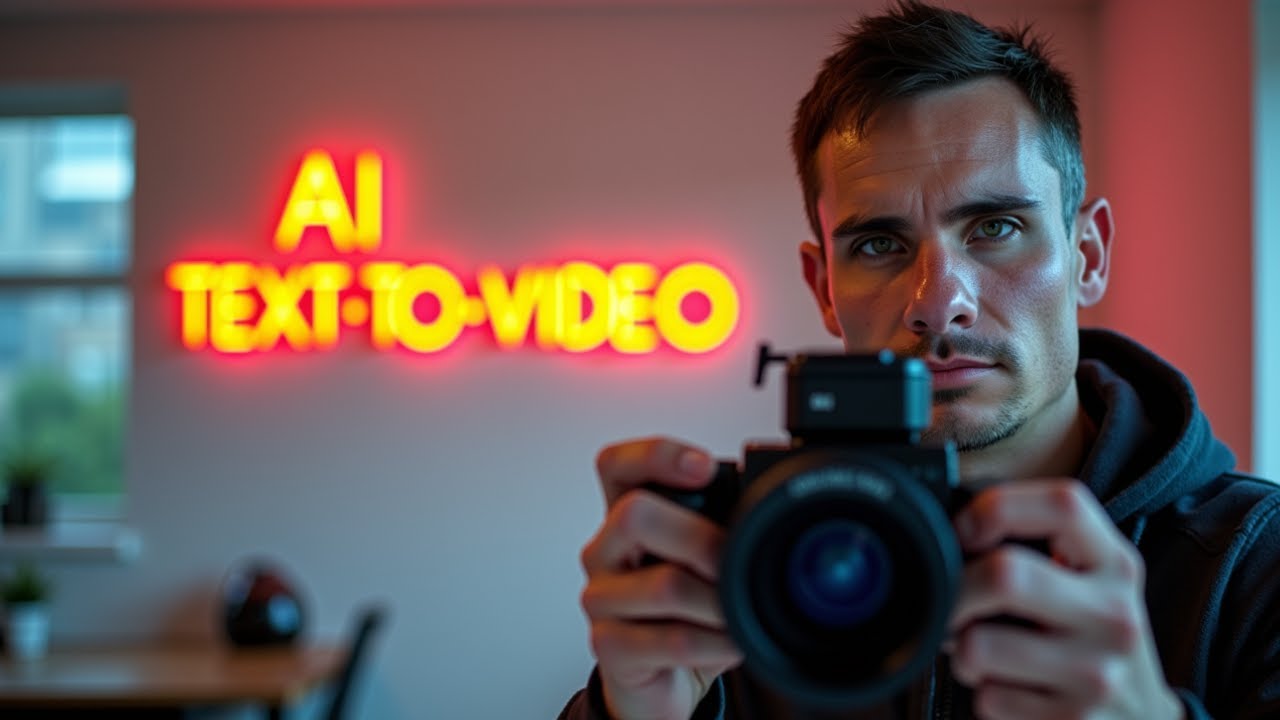
Introduction
Recent advancements in generative AI have sparked an exciting trend in the video space, particularly in the realms of image-to-video and text-to-video technologies. Given the emergence of sophisticated models like Flux, we are witnessing a rapid evolution in the capabilities of AI video generation tools. In this article, we will delve into a comprehensive comparison of three prominent AI text-to-video models: Kling Pro, MiniMax, and Luma Dream Machine.
To conduct this comparison, we will utilize Pixel Dojo, a platform that centralizes cutting-edge AI image and video generation tools. Additionally, we will employ the new movie Benchmark released by Meta, which provides a thousand diverse prompts for benchmarking text-to-video models. By selecting a handful of prompts from this extensive database, we will generate videos using each model and evaluate their performance.
Model Testing
We will evaluate each model based on specific prompts, starting with the prompt "a crab and octopus under the ocean."
Luma Dream Machine Results:
Luma's output featured a crab and octopus in the ocean. However, the animation was somewhat jerky and did not portray realistic movements. Unusual morphing effects disrupted the scene’s coherence, resulting in a confusing visual outcome.
MiniMax Results:
In contrast, MiniMax provided a better result, showcasing a more detailed octopus and crab with realistic movements, although there were minor issues, such as an oversized crab and a missing antenna. Still, the animation quality surpassed that of Luma Dream Machine.
Kling Pro Results:
The Kling Pro model produced varied results. It included interesting perspectives but also failed to capture recognizable creatures like crabs and octopus accurately. Although the motion reflected the perspective of a scuba diver, the output fell short in terms of clarity and coherence compared to MiniMax.
Second Test: Basketball Prompt
The next prompt set for evaluation was "a basketball through a hoop then explodes."
Luma Dream Machine Results:
Luma’s output featured a slow-motion scene where the basketball appeared to deteriorate visually. The prompt adherence was lacking, with no clear explosion occurring.
MiniMax Results:
MiniMax performed significantly better here, capturing the action of the basketball flying through the air followed by a more defined explosion, showcasing improvements in the overall animation.
Kling Pro Results:
Kling Pro presented a basketball scene as well, but the model displayed unexpected elements, such as water splashes that detracted from the main focus of the animation, further indicating a need for refinement.
Celebrity Prompt: Will Smith Eating Spaghetti
The subsequent prompt asked for “Will Smith eating a plate of spaghetti.”
Luma Dream Machine Results:
This model featured a character that bore only a slight resemblance to Will Smith and presented bizarre animations, such as noodles emerging from his mouth.
Kling Pro Results:
Kling Pro generated a visually appealing plate of spaghetti, but again, did not depict the character as Will Smith, and there was no mouth movement or actual eating depicted.
MiniMax Results:
MiniMax stood out by presenting a character that closely resembled Will Smith, and the character interacted with the spaghetti, marking it as the best of the three outputs for this particular prompt.
A Cinematic Pirate Battle
Next, we evaluated a more complex prompt: "photorealistic video of two pirate ships battling each other in a cup of coffee."
Luma Dream Machine Results:
Luma’s result was visually appealing but lacked dynamic motion within the scene. The ships appeared to float while the overall movement of the coffee was limited.
MiniMax Results:
MiniMax output included ships that appeared to battle but also lacked resolution details and motion.
Kling Pro Results:
Kling Pro’s entry produced a more dynamic scene with better motion dynamics, ultimately ranking favorably among the three.
Final Challenge: Image-to-Video Testing
In the last comparison, we performed image-to-video tests using original images generated in Pixel Dojo. We evaluated Kling Pro, Runway Gen 3, and MiniMax using the prompt "a woman smiles then walks off."
Runway Gen 3 Results:
Runway Gen 3 produced a decent video within a minute where the woman appeared to smile and turn, though there were minor details lost in terms of logo representation.
MiniMax Results:
In MiniMax, the woman smiled and walked backwards, but it retained the logo from Pixel Dojo, showing a distinct output.
Kling Pro Results:
Kling Pro's output revealed some limitations in detail but demonstrated overall action, providing less coherent results than Runway and MiniMax.
Conclusion
Each of these AI text-to-video models presents unique strengths and weaknesses. While MiniMax often had a consistent edge in terms of motion fluidity and adherence to prompts, Kling Pro occasionally showcased more dynamic outputs. Luma Dream Machine, while visually appealing, generally lacked the depth and realism seen in the other models. As these technologies continue to evolve, we can expect even more impressive results in the realm of AI-generated videos.
Keywords
- AI video generation
- Text-to-video models
- Kling Pro
- MiniMax
- Luma Dream Machine
- Pixel Dojo
- Benchmark prompts
- Animation quality
FAQ
1. What are the three models compared in this article?
The three models compared are Kling Pro, MiniMax, and Luma Dream Machine.
2. Where can I find the benchmarks used for the comparisons?
The benchmarks were taken from a recent release by Meta, which includes a thousand different prompts for text-to-video generation.
3. Which model performed the best overall in the tests?
MiniMax frequently showcased better results in terms of animation fluidity and prompt adherence across various testing scenarios.
4. What is Pixel Dojo?
Pixel Dojo is a platform that provides access to cutting-edge AI image and video generation tools, enabling users to experiment with different models and techniques.
5. How does AI text-to-video generation work?
AI text-to-video generation takes descriptive text prompts and uses algorithms to create animated video sequences that visually represent the input descriptions, using deep learning models trained on large datasets of images and videos.