Using GraphRAG to Generate Business Insights
Science & Technology
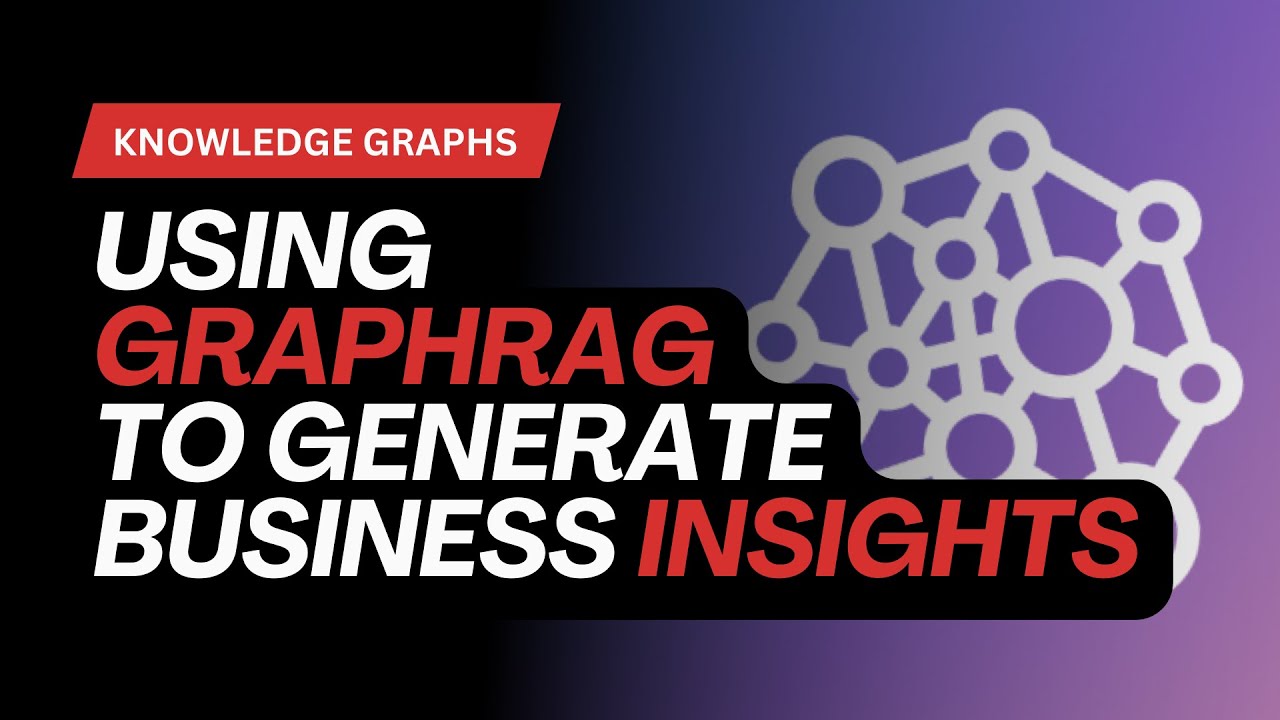
Introduction
Welcome to another insightful installment of the Intelligent Content Academy tutorial series. In today's session, we delve deep into the concept of GraphRAG—an advanced approach to augmenting business insights through intelligent content strategies. Marcello Lehn, the founder of Intelligent Content Academy, introduces this comprehensive topic, featuring guest presenter Allison Coet, a Developer Advocate at Neo4j. Allison takes us through how enterprises can successfully leverage GraphRAG to transform their data into actionable business insights.
What is GraphRAG?
GraphRAG, which stands for Retrieval-Augmented Generation, combines the strengths of generative AI applications with the structured data insights provided by graph databases. Traditional generative AI tools, such as ChatGPT, offer answers based on the input they receive. However, they lack the specificity and grounding in an organization's unique data. GraphRAG addresses that gap.
The process involves taking a user query, retrieving relevant information from a specific enterprise data source, and then providing a curated response, thus ensuring that the answer is both relevant and enhanced by contextual knowledge. This combination allows businesses to ground their insights in actual data, making them more actionable.
The Importance of Knowledge Graphs
Central to the concept of GraphRAG is the knowledge graph—a versatile data structure that organizes various types of relationships and entities to provide richer insights. A knowledge graph can incorporate structured data—such as customer information and transaction histories—as well as unstructured data, like documents and emails. Understanding the interconnections between these data types enhances an organization's ability to generate insights.
Components of a Graph
- Nodes: Represent entities (nouns) like people or products.
- Relationships: Define the connections between these entities.
- Properties: Provide additional depth to both nodes and relationships, allowing for a more nuanced understanding of the data.
By leveraging a knowledge graph, companies can uncover hidden patterns and insights that exist within their data, currently represented in tables and spreadsheets.
The Seven Graphs of Business
Allison introduces the concept of the “Seven Graphs of Business,” illustrating how interconnected various aspects of a business can be:
- Customer Graph: Tracking interactions, payments, and purchase history.
- Network Security Graph: Enabling threat detection and access pattern analysis.
- Employee Graph: Understanding career progression and team dynamics.
- Transaction Graph: Monitoring payments and invoice histories.
- Product Graph: Evaluating product relationships, reviews, and inventory needs.
- Process Graph: Analyzing internal procedures and automation.
- Supply Chain Graph: Understanding dependencies and relationships throughout the supply chain.
These interconnected graphs illustrate that businesses operate not as isolated entities but as complex networks of relationships and interactions.
Trailblazing Insights with GraphRAG
As we dive deeper into the practical applications of GraphRAG, Allison emphasizes the need for high-quality data sets, which are crucial for effective decision-making. She details how chatbots can be used to streamline operations, track conversations, and glean insights from user interactions.
Key aspects discussed include:
- Data Quality: Ensuring reliability and cleanliness of data collection.
- User Interactions: Utilizing conversational logs to analyze how users engage with the AI systems.
- Explaining AI Decisions: Building trust among users by explaining how AI reaches conclusions based on the data it analyzes.
Companies can utilize these insights to enhance customer service, improve employee onboarding, and visualize product connections to tailor marketing strategies effectively.
Conclusion
In summary, Allison’s presentation illustrates the incredible potential of GraphRAG as a tool for generating business insights. By merging generative AI capabilities with the structured, interconnected data provided by knowledge graphs, businesses are better positioned to understand and leverage their data for informed decision-making.
Keyword
GraphRAG, Retrieval-Augmented Generation, intelligent content, business insights, knowledge graph, nodes, relationships, properties, customer graph, network security graph, employee graph, transaction graph, product graph, process graph, supply chain graph, data quality, user interactions, explainable AI.
FAQ
Q1: What is GraphRAG?
A1: GraphRAG stands for Retrieval-Augmented Generation, a method that merges generative AI applications with specific enterprise data to produce well-grounded insights.
Q2: How do knowledge graphs function?
A2: Knowledge graphs organize entities, relationships, and properties, allowing for a richer understanding of interconnected data, enabling businesses to extract insights.
Q3: What are the Seven Graphs of Business?
A3: The Seven Graphs of Business refer to different interconnected data types including customer, network security, employee, transaction, product, process, and supply chain graphs that collectively illustrate a business’s operation as a complex network.
Q4: How can businesses utilize GraphRAG for customer insights?
A4: By employing user interaction logs and ensuring high-quality data, businesses can track inquiries and analyze requests to derive actionable insights for improving customer service and product offerings.
Q5: Why is explainability important in AI tools?
A5: Explainability builds trust in AI tools, enabling users to understand how decisions are made, ensuring better adoption and compliance with regulatory requirements in enterprises.