What is Prompt Tuning?
Education
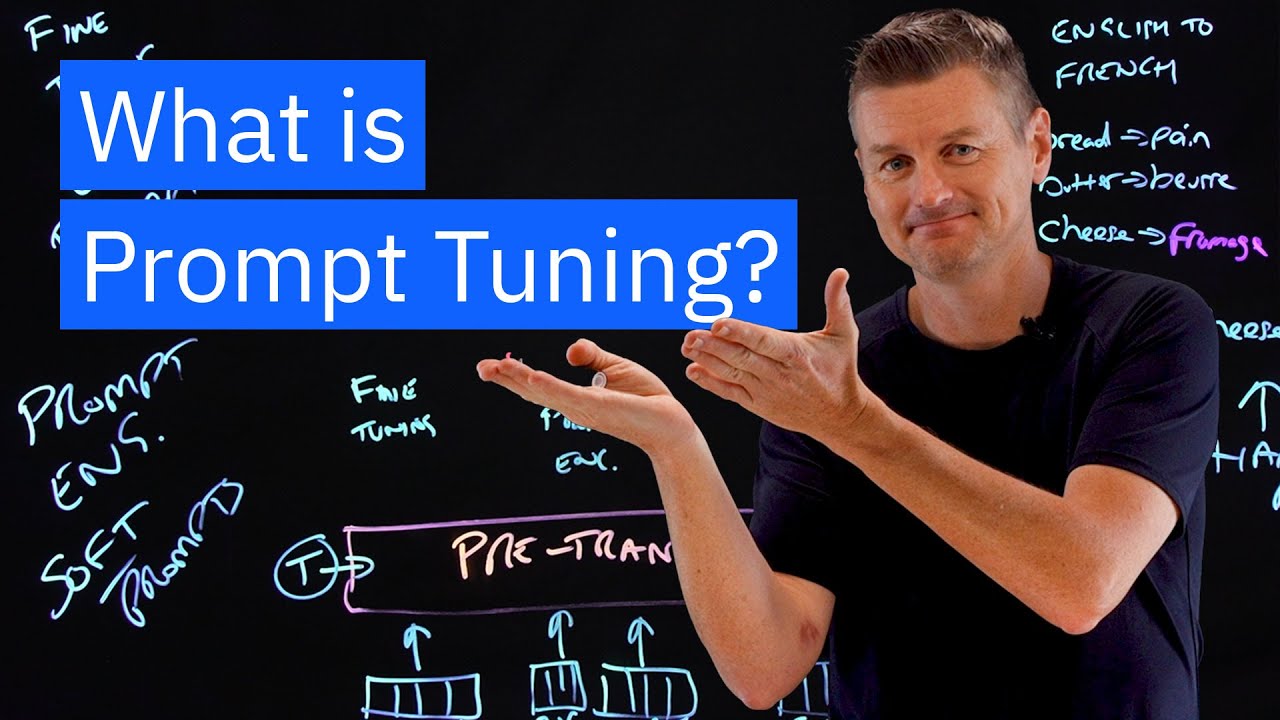
Introduction
Large language models like Chat's GPT are examples of foundation models, large reusable models that have been trained on vast amounts of knowledge on the internet. They are super flexible and can analyze various tasks from analyzing legal documents to writing poems. In the past, fine-tuning was the go-to method to improve the performance of pre-trained language models for specialized tasks. However, a newer, more energy-efficient technique known as prompt tuning has emerged as an alternative.
Prompt tuning allows for tailoring a massive model to a very narrow task without the need for gathering thousands of labeled examples. Instead of fine-tuning the entire model, specific cues or front-end prompts are fed to the AI model to provide task-specific context. Prompt engineering involves developing prompts to guide the model towards specialized tasks, while soft prompts, generated by AI, are replacing human-engineered prompts due to their effectiveness in guiding the model towards desired outputs.
In essence, prompt tuning involves leveraging soft prompts to specialize a pre-trained model by introducing task-specific information without the need for extensive data gathering and retraining. This technique is proving to be a game-changer in various fields, making it easier and faster to adapt models to specialized tasks.
Keywords
- Large language models
- Foundation models
- Fine-tuning
- Prompt tuning
- Prompt engineering
- Soft prompts
- Specialized tasks
- AI model adaptation
FAQ
- What are foundation models like Chat's GPT?
- How does prompt tuning differ from fine-tuning and prompt engineering?
- What are soft prompts and how do they enhance prompt tuning?
- In what areas is prompt tuning proving to be beneficial?