YOLO v11 vs. YOLO v10 vs. YOLO v8: Which is Best for Object Detection on COCO , OBB-Dota V1 Dataset
Education
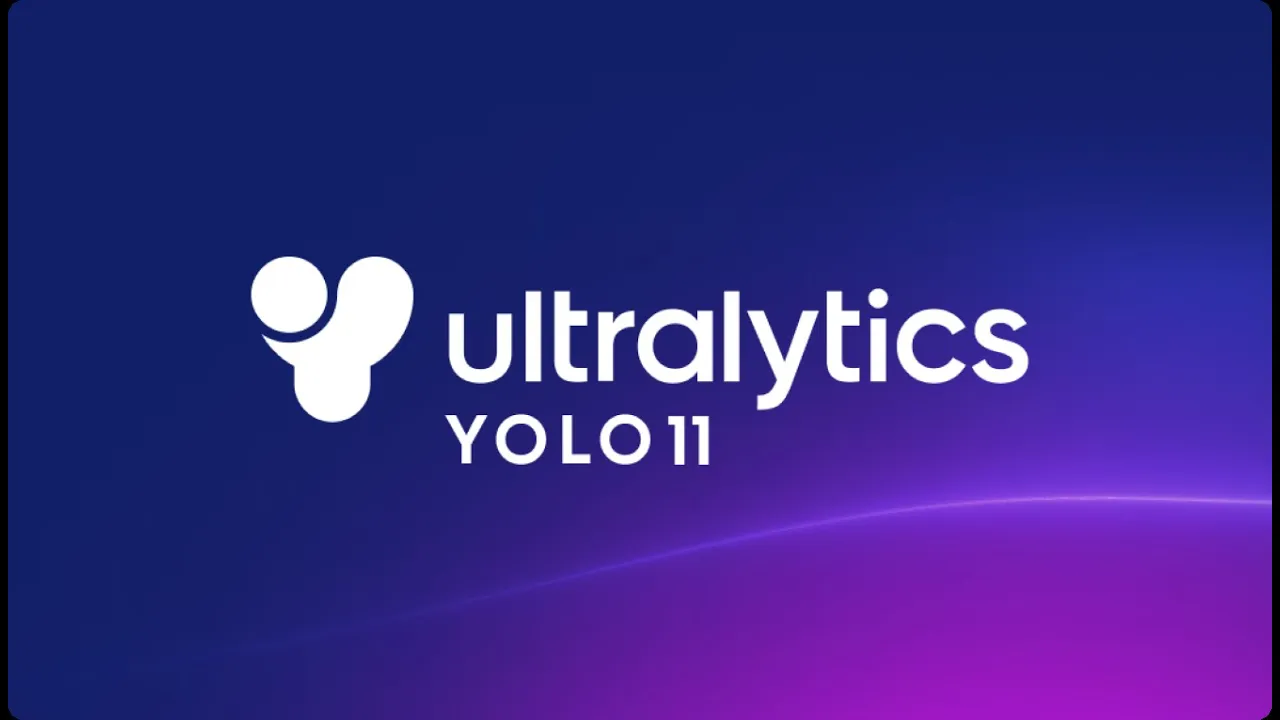
Introduction
In this article, we dive deep into the new YOLO v11 model released by Ultralytics and compare it with its predecessors, YOLO v10 and YOLO v8. We will discuss key differences, including the number of parameters, mean average precision (mAP), and inference speed.
Overview of YOLO Models
The YOLO (You Only Look Once) series has gained immense popularity in the field of object detection. The recent release of YOLO v11 came with exciting updates aimed at optimizing both performance and efficiency.
Key Features of YOLO v11
One of the primary improvements in YOLO v11 is the reduction in the number of parameters compared to YOLO v10 while maintaining a competitive mean average precision (mAP) on the COCO dataset. According to Ultralytics' press release, YOLO v11 has approximately 22% fewer parameters than YOLO v10 while achieving nearly the same mAP. Furthermore, YOLO v11 has reduced inference timings, being almost 2% faster than YOLO v10.
Comparison of Parameters
Below is a comparison of the number of parameters across different YOLO versions:
- YOLO v8 (Extra Large): 68 million parameters
- YOLO v10 (Extra Large): 29 million parameters
- YOLO v11 (Extra Large): Nearly 60 million parameters
For smaller models:
- YOLO v8 (Nano): 3.2 million parameters
- YOLO v10 (Nano): 2.3 million parameters
- YOLO v11 (Nano): 2.67 million parameters
From the analysis, we observe that YOLO v11 sits comfortably between YOLO v8 and YOLO v10 regarding parameters.
Performance Evaluation: Mean Average Precision (mAP)
When comparing mAP across the models:
- YOLO v8 (Extra Large): 53.9%
- YOLO v10 (Extra Large): 54.4%
- YOLO v11 (Extra Large): 54.7%
YOLO v11 shows a slight improvement over YOLO v10 and YOLO v8.
Inference Speed Analysis
To evaluate inference speed, we executed the models on images of varying sizes (from 1024x1024 down to 640x640). While the pre-processing time was slightly increased due to the larger input size, the inference time between YOLO v8 and YOLO v11 was comparable.
For a single image:
- YOLO v8: 3.57 ms (pre-processing), ~50 ms (inference)
- YOLO v11: 4.1 ms (pre-processing), ~50 ms (inference)
The inference time was nearly identical, with YOLO v11 having only a minimal increase of around 1 ms.
Object Detection Accuracy and Confidence Scores
Both the YOLO v8 and YOLO v11 models demonstrated high confidence scores on various objects when tested on the OBB-Dota V1 dataset. YOLO v11 performed slightly better, particularly for smaller objects.
In summary:
- For larger objects, YOLO v8 had better performance.
- For smaller objects and medium-sized detections, YOLO v11 outperformed YOLO v8.
Conclusion
Given the detailed comparisons and evaluations, it seems that while YOLO v11 offers improvements in reducing parameters and obtaining better confidence scores for smaller objects, it does not significantly enhance inference speeds over YOLO v8. Therefore, for tasks that require optimal accuracy with fewer resources, YOLO v11 is a solid choice. However, for larger objects, YOLO v8 may still hold an advantage.
Keywords
- YOLO v11
- YOLO v10
- YOLO v8
- Object detection
- COCO dataset
- OBB-Dota V1
- Mean Average Precision
- Inference speed
- Parameters
FAQ
Q1: What are the main improvements in YOLO v11 compared to v10 and v8?
A1: YOLO v11 has reduced parameters (22% less than v10), offers slightly better mean average precision, and maintains similar inference speeds.
Q2: How do the number of parameters compare among YOLO v8, v10, and v11?
A2: YOLO v8 (Extra Large) has 68 million parameters, YOLO v10 (Extra Large) has 29 million, and YOLO v11 (Extra Large) has nearly 60 million parameters.
Q3: Does YOLO v11 perform better on smaller objects compared to YOLO v8?
A3: Yes, YOLO v11 performs better for smaller object detections than YOLO v8, especially regarding confidence scores.
Q4: What is the impact of input image size on the YOLO model's inference time?
A4: Larger input image sizes lead to increased pre-processing times, while inference times remain relatively consistent across both YOLO v8 and YOLO v11 for single images.